How have the patterns of American military sacrifice changed throughout the 20th century, and how have said changes influenced our politics in the current year? This question has lingered in my mind for years. While it is a question tangentially related to my dissertation research, it has been a distraction that I cannot shake. I’ve written on this topic for Antiwar.com and on this blog. In both pieces, I argued a thesis that I still hold, that the sectional, economic, and racial disparities in military sacrifice have led to our current political strife.
But what about the past? How did we get here? Assuming that the latter is true, is it a new phenomenon? To answer it, I have, over the last few months, analyzed military fatality data available through the Defense Casualty Analysis System (DCAS) for the Global War on Terror (GWOT), the Vietnam War, as well as the Korean War. Yet, to really flesh this thing out, I needed records for the Second World War…and those are surprisingly difficult to obtain, at least in a transcribed form.
So I decided to do it myself and hand-jammed the county tabulations in the “WWII Army and Army Air Force Casualties” records currently available in pdf form from the National Archives. The records contain 3,123 county figures for all 48 states plus Washington, D.C., and total up to 307,757 U.S. Army and U.S. Army Air Forces killed and missing in action. I should note that the records also contain the names and geographic tabulations of those who hailed from America’s various territories (including Hawaii and Alaska).
These records do not contain figures for those who served within the Department of the Navy, which includes sailors, marines, and coastguardsmen. While the Navy records do list individuals and their street addresses, they do not offer county-level tabulations. I have, however, digitized the Navy Department’s state records and posted a CSV file with them and the Department of the Army figures. The said file is available on my GitHub.
Attempting a long-term analysis of county-level military fatalities without data from the Navy is, in the parlance of our times, problematic. The NARA records record that 66,370 sailors, marines, and coastguardmen were killed in the war. But there’s no way that I can, as a single person, transcribe every name and then geocode every address.
Spoiler alert: we’re still going to proceed, but before doing so, I’d like to pause and note that according to these datasets, 17.9% of American lives were lost during the war came from those who served within the Department of the Navy. They were not spread out evenly as 19 states endured slanted naval losses above the national average, including some highly populated states like California (graphic 1).
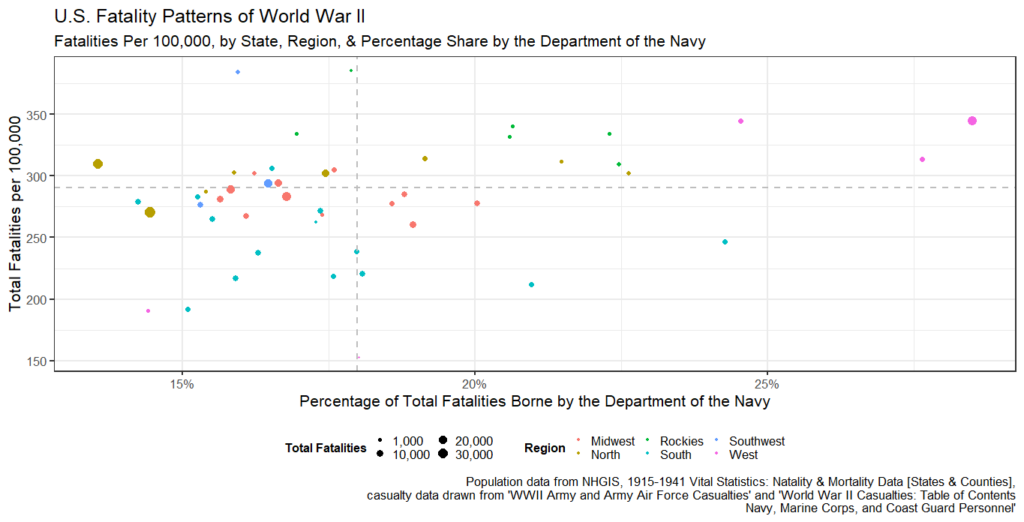
Even with incomplete records, that is, only those county-level statistics from the Department of the Army, I think we can still proceed with our inquiry. To make sense of the fatality records, I analyzed them against demographic data from the U.S. Census and the “1915-1941 Vital Statistics: Natality & Mortality Data [States & Counties]” made available through the National Historical Geographic Information System.1
Generating per capita statistics for the Department of the Army losses during the Second World War yields the following results (see interactive table below).
The table lists the county, state, and number of Army fatalities per 100k losses and the county’s percentage difference from the national per 100k (233.455). If you sort the records by the number of losses, unsurprisingly, the most populous county in 1940, Cook County, which contains Chicago, is at the top of the list. But, what is different from subsequent wars (to varying degrees, as we shall see) is that these highly populated counties all meet, exceed, or at the least come close to the national per 100k.
County | State | Number | Population in 1940 | Fatalities Per 100k | Difference from National Per 100k |
---|---|---|---|---|---|
Autauga | Alabama | 32 | 20977 | 152.5 | 65.3% |
Baldwin | Alabama | 44 | 32324 | 136.1 | 58.3% |
Barbour | Alabama | 36 | 32722 | 110.0 | 47.1% |
Bibb | Alabama | 39 | 20155 | 193.5 | 82.9% |
Blount | Alabama | 44 | 29490 | 149.2 | 63.9% |
Bullock | Alabama | 20 | 19810 | 101.0 | 43.2% |
Butler | Alabama | 58 | 32447 | 178.8 | 76.6% |
Calhoun | Alabama | 169 | 63319 | 266.9 | 114.3% |
Chambers | Alabama | 58 | 42146 | 137.6 | 58.9% |
Cherokee | Alabama | 29 | 19928 | 145.5 | 62.3% |
Chilton | Alabama | 49 | 27955 | 175.3 | 75.1% |
Choctaw | Alabama | 31 | 20195 | 153.5 | 65.8% |
Clarke | Alabama | 38 | 27636 | 137.5 | 58.9% |
Clay | Alabama | 27 | 16907 | 159.7 | 68.4% |
Cleburne | Alabama | 22 | 13629 | 161.4 | 69.1% |
Coffee | Alabama | 52 | 31987 | 162.6 | 69.6% |
Colbert | Alabama | 74 | 34093 | 217.1 | 93.0% |
Conecuh | Alabama | 27 | 25489 | 105.9 | 45.4% |
Coosa | Alabama | 30 | 13460 | 222.9 | 95.5% |
Covington | Alabama | 88 | 42417 | 207.5 | 88.9% |
Crenshaw | Alabama | 36 | 23631 | 152.3 | 65.3% |
Cullman | Alabama | 116 | 47343 | 245.0 | 105.0% |
Dale | Alabama | 35 | 22685 | 154.3 | 66.1% |
Dallas | Alabama | 71 | 55245 | 128.5 | 55.1% |
De Kalb | Alabama | 103 | 43075 | 239.1 | 102.4% |
Elmore | Alabama | 69 | 34546 | 199.7 | 85.6% |
Escambia | Alabama | 60 | 30671 | 195.6 | 83.8% |
Etowah | Alabama | 143 | 72580 | 197.0 | 84.4% |
Fayette | Alabama | 52 | 21651 | 240.2 | 102.9% |
Franklin | Alabama | 69 | 27552 | 250.4 | 107.3% |
Geneva | Alabama | 55 | 29172 | 188.5 | 80.8% |
Greene | Alabama | 10 | 19185 | 52.1 | 22.3% |
Hale | Alabama | 26 | 25533 | 101.8 | 43.6% |
Henry | Alabama | 23 | 21912 | 105.0 | 45.0% |
Houston | Alabama | 73 | 45665 | 159.9 | 68.5% |
Jackson | Alabama | 106 | 41802 | 253.6 | 108.6% |
Jefferson | Alabama | 874 | 459930 | 190.0 | 81.4% |
Lamar | Alabama | 39 | 19708 | 197.9 | 84.8% |
Lauderdale | Alabama | 90 | 46230 | 194.7 | 83.4% |
Lawrence | Alabama | 43 | 27880 | 154.2 | 66.1% |
Lee | Alabama | 62 | 36455 | 170.1 | 72.9% |
Limestone | Alabama | 59 | 35642 | 165.5 | 70.9% |
Lowndes | Alabama | 15 | 22661 | 66.2 | 28.4% |
Macon | Alabama | 28 | 27654 | 101.3 | 43.4% |
Madison | Alabama | 132 | 66317 | 199.0 | 85.3% |
Marengo | Alabama | 29 | 35736 | 81.2 | 34.8% |
Marion | Alabama | 74 | 28776 | 257.2 | 110.2% |
Marshall | Alabama | 102 | 42395 | 240.6 | 103.1% |
Mobile | Alabama | 249 | 141974 | 175.4 | 75.1% |
Monroe | Alabama | 24 | 29465 | 81.5 | 34.9% |
Montgomery | Alabama | 209 | 114420 | 182.7 | 78.2% |
Morgan | Alabama | 110 | 48148 | 228.5 | 97.9% |
Perry | Alabama | 21 | 26610 | 78.9 | 33.8% |
Pickens | Alabama | 40 | 27671 | 144.6 | 61.9% |
Pike | Alabama | 36 | 32493 | 110.8 | 47.5% |
Randolph | Alabama | 54 | 25516 | 211.6 | 90.7% |
Russell | Alabama | 51 | 35775 | 142.6 | 61.1% |
Shelby | Alabama | 41 | 28962 | 141.6 | 60.6% |
St Clair | Alabama | 63 | 27336 | 230.5 | 98.7% |
Sumter | Alabama | 21 | 27321 | 76.9 | 32.9% |
Talladega | Alabama | 132 | 51832 | 254.7 | 109.1% |
Tallapoosa | Alabama | 59 | 35270 | 167.3 | 71.7% |
Tuscaloosa | Alabama | 149 | 76036 | 196.0 | 83.9% |
Walker | Alabama | 150 | 64201 | 233.6 | 100.1% |
Washington | Alabama | 31 | 16188 | 191.5 | 82.0% |
Wilcox | Alabama | 34 | 26279 | 129.4 | 55.4% |
Winston | Alabama | 42 | 18746 | 224.0 | 96.0% |
Arkansas | Arkansas | 52 | 24437 | 212.8 | 91.1% |
Ashley | Arkansas | 52 | 26785 | 194.1 | 83.2% |
Baxter | Arkansas | 30 | 10281 | 291.8 | 125.0% |
Benton | Arkansas | 99 | 36148 | 273.9 | 117.3% |
Boone | Arkansas | 47 | 15860 | 296.3 | 126.9% |
Bradley | Arkansas | 39 | 18097 | 215.5 | 92.3% |
Calhoun | Arkansas | 29 | 9636 | 301.0 | 128.9% |
Carroll | Arkansas | 36 | 14737 | 244.3 | 104.6% |
Chicot | Arkansas | 32 | 27452 | 116.6 | 49.9% |
Clark | Arkansas | 49 | 24402 | 200.8 | 86.0% |
Clay | Arkansas | 65 | 28386 | 229.0 | 98.1% |
Cleburne | Arkansas | 26 | 13134 | 198.0 | 84.8% |
Cleveland | Arkansas | 22 | 12570 | 175.0 | 75.0% |
Columbia | Arkansas | 48 | 29822 | 161.0 | 68.9% |
Conway | Arkansas | 32 | 21536 | 148.6 | 63.6% |
Craighead | Arkansas | 100 | 47200 | 211.9 | 90.8% |
Crawford | Arkansas | 62 | 23920 | 259.2 | 111.0% |
Crittenden | Arkansas | 25 | 42473 | 58.9 | 25.2% |
Cross | Arkansas | 57 | 26046 | 218.8 | 93.7% |
Dallas | Arkansas | 22 | 14471 | 152.0 | 65.1% |
Desha | Arkansas | 46 | 27160 | 169.4 | 72.5% |
Drew | Arkansas | 30 | 19831 | 151.3 | 64.8% |
Faulkner | Arkansas | 62 | 25880 | 239.6 | 102.6% |
Franklin | Arkansas | 36 | 15683 | 229.5 | 98.3% |
Fulton | Arkansas | 27 | 10253 | 263.3 | 112.8% |
Garland | Arkansas | 95 | 41664 | 228.0 | 97.7% |
Grant | Arkansas | 24 | 10477 | 229.1 | 98.1% |
Greene | Arkansas | 50 | 30204 | 165.5 | 70.9% |
Hempstead | Arkansas | 52 | 32770 | 158.7 | 68.0% |
Hot Spring | Arkansas | 55 | 18916 | 290.8 | 124.5% |
Howard | Arkansas | 35 | 16621 | 210.6 | 90.2% |
Independence | Arkansas | 62 | 25643 | 241.8 | 103.6% |
Izard | Arkansas | 34 | 12834 | 264.9 | 113.5% |
Jackson | Arkansas | 50 | 26427 | 189.2 | 81.0% |
Jefferson | Arkansas | 86 | 65101 | 132.1 | 56.6% |
Johnson | Arkansas | 36 | 18795 | 191.5 | 82.0% |
Lafayette | Arkansas | 19 | 16851 | 112.8 | 48.3% |
Lawrence | Arkansas | 47 | 22651 | 207.5 | 88.9% |
Lee | Arkansas | 24 | 26810 | 89.5 | 38.3% |
Lincoln | Arkansas | 28 | 19709 | 142.1 | 60.9% |
Little River | Arkansas | 31 | 15932 | 194.6 | 83.3% |
Logan | Arkansas | 60 | 25967 | 231.1 | 99.0% |
Lonoke | Arkansas | 55 | 29802 | 184.6 | 79.1% |
Madison | Arkansas | 29 | 14531 | 199.6 | 85.5% |
Marion | Arkansas | 17 | 9464 | 179.6 | 76.9% |
Miller | Arkansas | 55 | 31874 | 172.6 | 73.9% |
Mississippi | Arkansas | 115 | 80217 | 143.4 | 61.4% |
Monroe | Arkansas | 33 | 21133 | 156.2 | 66.9% |
Montgomery | Arkansas | 22 | 8876 | 247.9 | 106.2% |
Nevada | Arkansas | 43 | 19869 | 216.4 | 92.7% |
Newton | Arkansas | 23 | 10881 | 211.4 | 90.5% |
Ouachita | Arkansas | 52 | 31151 | 166.9 | 71.5% |
Perry | Arkansas | 17 | 8392 | 202.6 | 86.8% |
Phillips | Arkansas | 53 | 45970 | 115.3 | 49.4% |
Pike | Arkansas | 29 | 11786 | 246.1 | 105.4% |
Poinsett | Arkansas | 85 | 37670 | 225.6 | 96.7% |
Polk | Arkansas | 47 | 15832 | 296.9 | 127.2% |
Pope | Arkansas | 35 | 25682 | 136.3 | 58.4% |
Prairie | Arkansas | 34 | 15304 | 222.2 | 95.2% |
Pulaski | Arkansas | 327 | 156085 | 209.5 | 89.7% |
Randolph | Arkansas | 30 | 18319 | 163.8 | 70.1% |
Saline | Arkansas | 38 | 19163 | 198.3 | 84.9% |
Scott | Arkansas | 26 | 13300 | 195.5 | 83.7% |
Searcy | Arkansas | 24 | 11942 | 201.0 | 86.1% |
Sebastian | Arkansas | 154 | 62809 | 245.2 | 105.0% |
Sevier | Arkansas | 34 | 15248 | 223.0 | 95.5% |
Sharp | Arkansas | 27 | 11497 | 234.8 | 100.6% |
St Francis | Arkansas | 41 | 36043 | 113.8 | 48.7% |
St.one | Arkansas | 14 | 8603 | 162.7 | 69.7% |
Union | Arkansas | 94 | 50461 | 186.3 | 79.8% |
Van Buren | Arkansas | 36 | 12518 | 287.6 | 123.2% |
Washington | Arkansas | 105 | 41114 | 255.4 | 109.4% |
White | Arkansas | 76 | 37176 | 204.4 | 87.6% |
Woodruff | Arkansas | 32 | 22133 | 144.6 | 61.9% |
Yell | Arkansas | 48 | 20970 | 228.9 | 98.0% |
Apache | Arizona | 51 | 24095 | 211.7 | 90.7% |
Cochise | Arizona | 111 | 34627 | 320.6 | 137.3% |
Coconino | Arizona | 49 | 18770 | 261.1 | 111.8% |
Gila | Arizona | 87 | 23867 | 364.5 | 156.1% |
Graham | Arizona | 49 | 12113 | 404.5 | 173.3% |
Greenlee | Arizona | 31 | 8698 | 356.4 | 152.7% |
Maricopa | Arizona | 514 | 186193 | 276.1 | 118.2% |
Mohave | Arizona | 22 | 8591 | 256.1 | 109.7% |
Navajo | Arizona | 64 | 25309 | 252.9 | 108.3% |
Pima | Arizona | 239 | 72838 | 328.1 | 140.6% |
Pinal | Arizona | 115 | 28841 | 398.7 | 170.8% |
Santa Cruz | Arizona | 43 | 9482 | 453.5 | 194.3% |
Yavapai | Arizona | 80 | 26511 | 301.8 | 129.3% |
Yuma | Arizona | 80 | 19326 | 414.0 | 177.3% |
Alameda | California | 1266 | 513011 | 246.8 | 105.7% |
Alpine | California | 1 | 323 | 309.6 | 132.6% |
Amador | California | 22 | 8973 | 245.2 | 105.0% |
Butte | California | 107 | 42840 | 249.8 | 107.0% |
Calaveras | California | 21 | 8221 | 255.4 | 109.4% |
Colusa | California | 25 | 9788 | 255.4 | 109.4% |
Contra Costa | California | 320 | 100450 | 318.6 | 136.5% |
Del Norte | California | 7 | 4745 | 147.5 | 63.2% |
El Dorado | California | 27 | 13229 | 204.1 | 87.4% |
Fresno | California | 511 | 178565 | 286.2 | 122.6% |
Glenn | California | 32 | 12195 | 262.4 | 112.4% |
Humboldt | California | 114 | 45812 | 248.8 | 106.6% |
Imperial | California | 107 | 59740 | 179.1 | 76.7% |
Inyo | California | 28 | 7625 | 367.2 | 157.3% |
Kern | California | 340 | 135124 | 251.6 | 107.8% |
Kings | California | 104 | 35168 | 295.7 | 126.7% |
Lake | California | 26 | 8069 | 322.2 | 138.0% |
Lassen | California | 49 | 14479 | 338.4 | 145.0% |
Los Angeles | California | 6674 | 2785643 | 239.6 | 102.6% |
Madera | California | 79 | 23314 | 338.9 | 145.1% |
Marin | California | 103 | 52907 | 194.7 | 83.4% |
Mariposa | California | 6 | 5605 | 107.0 | 45.9% |
Mendocino | California | 54 | 27864 | 193.8 | 83.0% |
Merced | California | 103 | 46988 | 219.2 | 93.9% |
Modoc | California | 23 | 8713 | 264.0 | 113.1% |
Mono | California | 11 | 2299 | 478.5 | 205.0% |
Monterey | California | 240 | 73032 | 328.6 | 140.8% |
Napa | California | 57 | 28503 | 200.0 | 85.7% |
Nevada | California | 42 | 19283 | 217.8 | 93.3% |
Orange | California | 334 | 130760 | 255.4 | 109.4% |
Placer | California | 75 | 28108 | 266.8 | 114.3% |
Plumas | California | 49 | 11548 | 424.3 | 181.8% |
Riverside | California | 314 | 105524 | 297.6 | 127.5% |
Sacramento | California | 441 | 170333 | 258.9 | 110.9% |
San Benito | California | 36 | 11392 | 316.0 | 135.4% |
San Bernardino | California | 441 | 161108 | 273.7 | 117.3% |
San Diego | California | 775 | 289348 | 267.8 | 114.7% |
San Francisco | California | 1365 | 634536 | 215.1 | 92.1% |
San Joaquin | California | 283 | 134207 | 210.9 | 90.3% |
San Luis Obispo | California | 89 | 33246 | 267.7 | 114.7% |
San Mateo | California | 220 | 111782 | 196.8 | 84.3% |
Santa Barbara | California | 153 | 70555 | 216.9 | 92.9% |
Santa Clara | California | 441 | 174949 | 252.1 | 108.0% |
Santa Cruz | California | 88 | 45057 | 195.3 | 83.7% |
Shasta | California | 69 | 28800 | 239.6 | 102.6% |
Sierra | California | 12 | 3025 | 396.7 | 169.9% |
Siskiyou | California | 72 | 28598 | 251.8 | 107.8% |
Solano | California | 150 | 49118 | 305.4 | 130.8% |
Sonoma | California | 165 | 69052 | 239.0 | 102.4% |
St.anislaus | California | 178 | 74866 | 237.8 | 101.8% |
Sutter | California | 61 | 18680 | 326.6 | 139.9% |
Tehama | California | 17 | 14316 | 118.7 | 50.9% |
Trinity | California | 9 | 3970 | 226.7 | 97.1% |
Tulare | California | 246 | 107152 | 229.6 | 98.3% |
Tuolumne | California | 26 | 10887 | 238.8 | 102.3% |
Ventura | California | 216 | 69685 | 310.0 | 132.8% |
Yolo | California | 72 | 27243 | 264.3 | 113.2% |
Yuba | California | 45 | 17034 | 264.2 | 113.2% |
Adams | Colorado | 42 | 22481 | 186.8 | 80.0% |
Alamosa | Colorado | 34 | 10484 | 324.3 | 138.9% |
Arapahoe | Colorado | 64 | 32150 | 199.1 | 85.3% |
Archuleta | Colorado | 17 | 3806 | 446.7 | 191.3% |
Baca | Colorado | 14 | 6207 | 225.6 | 96.6% |
Bent | Colorado | 25 | 9653 | 259.0 | 110.9% |
Boulder | Colorado | 88 | 37438 | 235.1 | 100.7% |
Chaffee | Colorado | 18 | 8109 | 222.0 | 95.1% |
Cheyenne | Colorado | 9 | 2964 | 303.6 | 130.1% |
Clear Creek | Colorado | 9 | 3784 | 237.8 | 101.9% |
Conejos | Colorado | 31 | 11648 | 266.1 | 114.0% |
Costilla | Colorado | 11 | 7533 | 146.0 | 62.5% |
Crowley | Colorado | 22 | 5398 | 407.6 | 174.6% |
Custer | Colorado | 7 | 2270 | 308.4 | 132.1% |
Delta | Colorado | 44 | 16470 | 267.2 | 114.4% |
Denver | Colorado | 786 | 322412 | 243.8 | 104.4% |
Dolores | Colorado | 1 | 1958 | 51.1 | 21.9% |
Douglas | Colorado | 7 | 3496 | 200.2 | 85.8% |
Eagle | Colorado | 7 | 5361 | 130.6 | 55.9% |
Elbert | Colorado | 3 | 5460 | 54.9 | 23.5% |
El Paso | Colorado | 141 | 54025 | 261.0 | 111.8% |
Fremont | Colorado | 53 | 19742 | 268.5 | 115.0% |
Garfield | Colorado | 29 | 10560 | 274.6 | 117.6% |
Gilpin | Colorado | 1 | 1625 | 61.5 | 26.4% |
Grand | Colorado | 14 | 3587 | 390.3 | 167.2% |
Gunnison | Colorado | 16 | 6192 | 258.4 | 110.7% |
Hinsdale | Colorado | 2 | 349 | 573.1 | 245.5% |
Huerfano | Colorado | 42 | 16088 | 261.1 | 111.8% |
Jackson | Colorado | 6 | 1798 | 333.7 | 142.9% |
Jefferson | Colorado | 57 | 30725 | 185.5 | 79.5% |
Kiowa | Colorado | 15 | 2793 | 537.1 | 230.0% |
Kit Carson | Colorado | 16 | 7512 | 213.0 | 91.2% |
Lake | Colorado | 19 | 6883 | 276.0 | 118.2% |
La Plata | Colorado | 39 | 15494 | 251.7 | 107.8% |
Larimer | Colorado | 77 | 35539 | 216.7 | 92.8% |
Las Animas | Colorado | 81 | 32369 | 250.2 | 107.2% |
Lincoln | Colorado | 17 | 5882 | 289.0 | 123.8% |
Logan | Colorado | 39 | 18370 | 212.3 | 90.9% |
Mesa | Colorado | 73 | 33791 | 216.0 | 92.5% |
Mineral | Colorado | 1 | 975 | 102.6 | 43.9% |
Moffat | Colorado | 15 | 5086 | 294.9 | 126.3% |
Montezuma | Colorado | 20 | 10463 | 191.1 | 81.9% |
Montrose | Colorado | 45 | 15418 | 291.9 | 125.0% |
Morgan | Colorado | 50 | 17214 | 290.5 | 124.4% |
Otero | Colorado | 39 | 23571 | 165.5 | 70.9% |
Ouray | Colorado | 4 | 2089 | 191.5 | 82.0% |
Park | Colorado | 7 | 3272 | 213.9 | 91.6% |
Phillips | Colorado | 18 | 4948 | 363.8 | 155.8% |
Pitkin | Colorado | 2 | 1836 | 108.9 | 46.7% |
Prowers | Colorado | 44 | 12304 | 357.6 | 153.2% |
Pueblo | Colorado | 151 | 68870 | 219.3 | 93.9% |
Rio Blanco | Colorado | 14 | 2943 | 475.7 | 203.8% |
Rio Grande | Colorado | 30 | 12404 | 241.9 | 103.6% |
Routt | Colorado | 25 | 10525 | 237.5 | 101.7% |
Saguache | Colorado | 14 | 6173 | 226.8 | 97.1% |
San Juan | Colorado | 3 | 1439 | 208.5 | 89.3% |
San Miguel | Colorado | 8 | 3664 | 218.3 | 93.5% |
Sedgwick | Colorado | 9 | 5294 | 170.0 | 72.8% |
Summit | Colorado | 2 | 1754 | 114.0 | 48.8% |
Teller | Colorado | 15 | 6463 | 232.1 | 99.4% |
Washington | Colorado | 11 | 8336 | 132.0 | 56.5% |
Weld | Colorado | 136 | 63747 | 213.3 | 91.4% |
Yuma | Colorado | 30 | 12102 | 247.9 | 106.2% |
Fairfield | Connecticut | 1097 | 418384 | 262.2 | 112.3% |
Hartford | Connecticut | 1238 | 450189 | 275.0 | 117.8% |
Litchfield | Connecticut | 232 | 87041 | 266.5 | 114.2% |
Middlesex | Connecticut | 136 | 55999 | 242.9 | 104.0% |
New Haven | Connecticut | 1143 | 484316 | 236.0 | 101.1% |
New London | Connecticut | 250 | 125224 | 199.6 | 85.5% |
Tolland | Connecticut | 66 | 31866 | 207.1 | 88.7% |
Windham | Connecticut | 147 | 56223 | 261.5 | 112.0% |
District Of Columbia | District of Columbia | 3029 | 663091 | 456.8 | 195.7% |
Kent | Delaware | 59 | 34441 | 171.3 | 73.4% |
New Castle | Delaware | 392 | 179562 | 218.3 | 93.5% |
Sussex | Delaware | 91 | 52502 | 173.3 | 74.2% |
Alachua | Florida | 59 | 38607 | 152.8 | 65.5% |
Baker | Florida | 18 | 6510 | 276.5 | 118.4% |
Bay | Florida | 56 | 20686 | 270.7 | 116.0% |
Bradford | Florida | 17 | 8717 | 195.0 | 83.5% |
Brevard | Florida | 26 | 16142 | 161.1 | 69.0% |
Broward | Florida | 50 | 39794 | 125.6 | 53.8% |
Calhoun | Florida | 24 | 8218 | 292.0 | 125.1% |
Charlotte | Florida | 8 | 3663 | 218.4 | 93.6% |
Citrus | Florida | 16 | 5846 | 273.7 | 117.2% |
Clay | Florida | 13 | 6468 | 201.0 | 86.1% |
Collier | Florida | 8 | 5102 | 156.8 | 67.2% |
Columbia | Florida | 27 | 16859 | 160.2 | 68.6% |
De Soto | Florida | 13 | 7792 | 166.8 | 71.5% |
Dixie | Florida | 10 | 7018 | 142.5 | 61.0% |
Duval | Florida | 392 | 210143 | 186.5 | 79.9% |
Escambia | Florida | 124 | 74667 | 166.1 | 71.1% |
Flagler | Florida | 5 | 3008 | 166.2 | 71.2% |
Franklin | Florida | 11 | 5991 | 183.6 | 78.6% |
Gadsden | Florida | 43 | 31450 | 136.7 | 58.6% |
Gilchrist | Florida | 9 | 4250 | 211.8 | 90.7% |
Glades | Florida | 6 | 2745 | 218.6 | 93.6% |
Gulf | Florida | 17 | 6951 | 244.6 | 104.8% |
Hamilton | Florida | 13 | 9778 | 133.0 | 56.9% |
Hardee | Florida | 25 | 10158 | 246.1 | 105.4% |
Hendry | Florida | 16 | 5237 | 305.5 | 130.9% |
Hernando | Florida | 15 | 5641 | 265.9 | 113.9% |
Highlands | Florida | 28 | 9246 | 302.8 | 129.7% |
Hillsborough | Florida | 363 | 180148 | 201.5 | 86.3% |
Holmes | Florida | 35 | 15447 | 226.6 | 97.1% |
Indian River | Florida | 16 | 8957 | 178.6 | 76.5% |
Jackson | Florida | 54 | 34428 | 156.8 | 67.2% |
Jefferson | Florida | 9 | 12032 | 74.8 | 32.0% |
Lafayette | Florida | 6 | 4405 | 136.2 | 58.3% |
Lake | Florida | 53 | 27255 | 194.5 | 83.3% |
Lee | Florida | 43 | 17488 | 245.9 | 105.3% |
Leon | Florida | 56 | 31646 | 177.0 | 75.8% |
Levy | Florida | 23 | 12550 | 183.3 | 78.5% |
Liberty | Florida | 10 | 3752 | 266.5 | 114.2% |
Madison | Florida | 19 | 16190 | 117.4 | 50.3% |
Manatee | Florida | 46 | 26098 | 176.3 | 75.5% |
Marion | Florida | 44 | 31243 | 140.8 | 60.3% |
Martin | Florida | 7 | 6295 | 111.2 | 47.6% |
Dade | Florida | 503 | 267739 | 187.9 | 80.5% |
Monroe | Florida | 30 | 14078 | 213.1 | 91.3% |
Nassau | Florida | 15 | 10826 | 138.6 | 59.3% |
Okaloosa | Florida | 34 | 12900 | 263.6 | 112.9% |
Okeechobee | Florida | 7 | 3000 | 233.3 | 99.9% |
Orange | Florida | 173 | 70074 | 246.9 | 105.8% |
Osceola | Florida | 14 | 10119 | 138.4 | 59.3% |
Palm Beach | Florida | 122 | 79989 | 152.5 | 65.3% |
Pasco | Florida | 29 | 13981 | 207.4 | 88.8% |
Pinellas | Florida | 138 | 91852 | 150.2 | 64.4% |
Polk | Florida | 200 | 86665 | 230.8 | 98.9% |
Putnam | Florida | 25 | 18698 | 133.7 | 57.3% |
Santa Rosa | Florida | 28 | 16085 | 174.1 | 74.6% |
Sarasota | Florida | 33 | 16106 | 204.9 | 87.8% |
Seminole | Florida | 37 | 22304 | 165.9 | 71.1% |
St Johns | Florida | 41 | 20012 | 204.9 | 87.8% |
St Lucie | Florida | 20 | 11871 | 168.5 | 72.2% |
Sumter | Florida | 19 | 11041 | 172.1 | 73.7% |
Suwannee | Florida | 29 | 17073 | 169.9 | 72.8% |
Taylor | Florida | 16 | 11565 | 138.3 | 59.3% |
Union | Florida | 9 | 7094 | 126.9 | 54.3% |
Volusia | Florida | 87 | 53710 | 162.0 | 69.4% |
Wakulla | Florida | 8 | 5463 | 146.4 | 62.7% |
Walton | Florida | 31 | 14246 | 217.6 | 93.2% |
Washington | Florida | 25 | 12302 | 203.2 | 87.0% |
Appling | Georgia | 33 | 14497 | 227.6 | 97.5% |
Atkinson | Georgia | 12 | 7093 | 169.2 | 72.5% |
Bacon | Georgia | 15 | 8096 | 185.3 | 79.4% |
Baker | Georgia | 5 | 7344 | 68.1 | 29.2% |
Baldwin | Georgia | 29 | 24190 | 119.9 | 51.4% |
Banks | Georgia | 11 | 8733 | 126.0 | 54.0% |
Barrow | Georgia | 40 | 13064 | 306.2 | 131.2% |
Bartow | Georgia | 47 | 25283 | 185.9 | 79.6% |
Ben Hill | Georgia | 22 | 14523 | 151.5 | 64.9% |
Berrien | Georgia | 18 | 15370 | 117.1 | 50.2% |
Bibb | Georgia | 160 | 83783 | 191.0 | 81.8% |
Bleckley | Georgia | 22 | 9655 | 227.9 | 97.6% |
Brantley | Georgia | 17 | 6871 | 247.4 | 106.0% |
Brooks | Georgia | 23 | 20497 | 112.2 | 48.1% |
Bryan | Georgia | 12 | 6288 | 190.8 | 81.7% |
Bulloch | Georgia | 33 | 26010 | 126.9 | 54.3% |
Burke | Georgia | 24 | 26520 | 90.5 | 38.8% |
Butts | Georgia | 19 | 9182 | 206.9 | 88.6% |
Calhoun | Georgia | 16 | 10438 | 153.3 | 65.7% |
Camden | Georgia | 7 | 5910 | 118.4 | 50.7% |
Candler | Georgia | 11 | 9103 | 120.8 | 51.8% |
Carroll | Georgia | 78 | 34156 | 228.4 | 97.8% |
Catoosa | Georgia | 19 | 12199 | 155.8 | 66.7% |
Charlton | Georgia | 17 | 5256 | 323.4 | 138.5% |
Chatham | Georgia | 223 | 117970 | 189.0 | 81.0% |
Chattahoochee | Georgia | 12 | 15138 | 79.3 | 34.0% |
Chattooga | Georgia | 43 | 18532 | 232.0 | 99.4% |
Cherokee | Georgia | 42 | 20126 | 208.7 | 89.4% |
Clarke | Georgia | 59 | 28398 | 207.8 | 89.0% |
Clay | Georgia | 9 | 7064 | 127.4 | 54.6% |
Clayton | Georgia | 25 | 11655 | 214.5 | 91.9% |
Clinch | Georgia | 7 | 6437 | 108.7 | 46.6% |
Cobb | Georgia | 70 | 38272 | 182.9 | 78.3% |
Coffee | Georgia | 42 | 21541 | 195.0 | 83.5% |
Colquitt | Georgia | 58 | 33012 | 175.7 | 75.3% |
Columbia | Georgia | 12 | 9433 | 127.2 | 54.5% |
Cook | Georgia | 30 | 11919 | 251.7 | 107.8% |
Coweta | Georgia | 42 | 26972 | 155.7 | 66.7% |
Crawford | Georgia | 12 | 7128 | 168.4 | 72.1% |
Crisp | Georgia | 24 | 17540 | 136.8 | 58.6% |
Dade | Georgia | 13 | 5894 | 220.6 | 94.5% |
Dawson | Georgia | 9 | 4479 | 200.9 | 86.1% |
Decatur | Georgia | 49 | 22234 | 220.4 | 94.4% |
Dekalb | Georgia | 120 | 86942 | 138.0 | 59.1% |
Dodge | Georgia | 33 | 21022 | 157.0 | 67.2% |
Dooly | Georgia | 25 | 16886 | 148.1 | 63.4% |
Dougherty | Georgia | 55 | 28565 | 192.5 | 82.5% |
Douglas | Georgia | 15 | 10053 | 149.2 | 63.9% |
Early | Georgia | 14 | 18679 | 75.0 | 32.1% |
Echols | Georgia | 4 | 2964 | 135.0 | 57.8% |
Effingham | Georgia | 13 | 9646 | 134.8 | 57.7% |
Elbert | Georgia | 31 | 19618 | 158.0 | 67.7% |
Emanuel | Georgia | 32 | 23517 | 136.1 | 58.3% |
Evans | Georgia | 10 | 7401 | 135.1 | 57.9% |
Fannin | Georgia | 30 | 14752 | 203.4 | 87.1% |
Fayette | Georgia | 14 | 8170 | 171.4 | 73.4% |
Floyd | Georgia | 115 | 56141 | 204.8 | 87.7% |
Forsyth | Georgia | 13 | 11322 | 114.8 | 49.2% |
Franklin | Georgia | 24 | 15612 | 153.7 | 65.8% |
Fulton | Georgia | 883 | 392886 | 224.7 | 96.3% |
Gilmer | Georgia | 26 | 9001 | 288.9 | 123.7% |
Glascock | Georgia | 10 | 4547 | 219.9 | 94.2% |
Glynn | Georgia | 60 | 21920 | 273.7 | 117.2% |
Gordon | Georgia | 43 | 18445 | 233.1 | 99.9% |
Grady | Georgia | 33 | 19654 | 167.9 | 71.9% |
Greene | Georgia | 27 | 13709 | 197.0 | 84.4% |
Gwinnett | Georgia | 57 | 29087 | 196.0 | 83.9% |
Habersham | Georgia | 30 | 14771 | 203.1 | 87.0% |
Hall | Georgia | 99 | 34822 | 284.3 | 121.8% |
Hancock | Georgia | 18 | 12764 | 141.0 | 60.4% |
Haralson | Georgia | 28 | 14377 | 194.8 | 83.4% |
Harris | Georgia | 16 | 11428 | 140.0 | 60.0% |
Hart | Georgia | 26 | 15512 | 167.6 | 71.8% |
Heard | Georgia | 12 | 8610 | 139.4 | 59.7% |
Henry | Georgia | 20 | 15119 | 132.3 | 56.7% |
Houston | Georgia | 12 | 11303 | 106.2 | 45.5% |
Irwin | Georgia | 10 | 12936 | 77.3 | 33.1% |
Jackson | Georgia | 48 | 20089 | 238.9 | 102.3% |
Jasper | Georgia | 19 | 8772 | 216.6 | 92.8% |
Jeff Davis | Georgia | 13 | 8841 | 147.0 | 63.0% |
Jefferson | Georgia | 32 | 20040 | 159.7 | 68.4% |
Jenkins | Georgia | 16 | 11843 | 135.1 | 57.9% |
Johnson | Georgia | 18 | 12953 | 139.0 | 59.5% |
Jones | Georgia | 10 | 8331 | 120.0 | 51.4% |
Lamar | Georgia | 23 | 10091 | 227.9 | 97.6% |
Lanier | Georgia | 13 | 5632 | 230.8 | 98.9% |
Laurens | Georgia | 76 | 33606 | 226.2 | 96.9% |
Lee | Georgia | 8 | 7837 | 102.1 | 43.7% |
Liberty | Georgia | 12 | 8595 | 139.6 | 59.8% |
Lincoln | Georgia | 7 | 7042 | 99.4 | 42.6% |
Long | Georgia | 6 | 4086 | 146.8 | 62.9% |
Lowndes | Georgia | 50 | 31860 | 156.9 | 67.2% |
Lumpkin | Georgia | 14 | 6223 | 225.0 | 96.4% |
Macon | Georgia | 44 | 15947 | 275.9 | 118.2% |
Madison | Georgia | 32 | 13431 | 238.3 | 102.1% |
Marion | Georgia | 5 | 6954 | 71.9 | 30.8% |
Mcduffie | Georgia | 31 | 10878 | 285.0 | 122.1% |
Meriwether | Georgia | 36 | 22055 | 163.2 | 69.9% |
Miller | Georgia | 12 | 9998 | 120.0 | 51.4% |
Mitchell | Georgia | 27 | 23261 | 116.1 | 49.7% |
Monroe | Georgia | 11 | 10749 | 102.3 | 43.8% |
Montgomery | Georgia | 7 | 9668 | 72.4 | 31.0% |
Morgan | Georgia | 20 | 12713 | 157.3 | 67.4% |
Murray | Georgia | 23 | 11137 | 206.5 | 88.5% |
Muscogee | Georgia | 207 | 75494 | 274.2 | 117.5% |
Newton | Georgia | 36 | 18576 | 193.8 | 83.0% |
Oconee | Georgia | 16 | 7576 | 211.2 | 90.5% |
Oglethorpe | Georgia | 6 | 12430 | 48.3 | 20.7% |
Paulding | Georgia | 21 | 12832 | 163.7 | 70.1% |
Peach | Georgia | 20 | 10378 | 192.7 | 82.5% |
Pickens | Georgia | 13 | 9136 | 142.3 | 61.0% |
Pierce | Georgia | 24 | 11800 | 203.4 | 87.1% |
Pike | Georgia | 18 | 10375 | 173.5 | 74.3% |
Polk | Georgia | 64 | 28467 | 224.8 | 96.3% |
Pulaski | Georgia | 12 | 9829 | 122.1 | 52.3% |
Putnam | Georgia | 12 | 8514 | 140.9 | 60.4% |
Quitman | Georgia | 8 | 3435 | 232.9 | 99.8% |
Rabun | Georgia | 20 | 7821 | 255.7 | 109.5% |
Randolph | Georgia | 12 | 16609 | 72.2 | 30.9% |
Richmond | Georgia | 138 | 81863 | 168.6 | 72.2% |
Rockdale | Georgia | 14 | 7724 | 181.3 | 77.6% |
Schley | Georgia | 8 | 5033 | 159.0 | 68.1% |
Screven | Georgia | 31 | 20353 | 152.3 | 65.2% |
Seminole | Georgia | 13 | 8492 | 153.1 | 65.6% |
Spalding | Georgia | 62 | 28427 | 218.1 | 93.4% |
St.ephens | Georgia | 38 | 12972 | 292.9 | 125.5% |
St.ewart | Georgia | 10 | 10603 | 94.3 | 40.4% |
Sumter | Georgia | 35 | 24502 | 142.8 | 61.2% |
Talbot | Georgia | 5 | 8141 | 61.4 | 26.3% |
Taliaferro | Georgia | 4 | 6278 | 63.7 | 27.3% |
Tattnall | Georgia | 26 | 16243 | 160.1 | 68.6% |
Taylor | Georgia | 17 | 10768 | 157.9 | 67.6% |
Telfair | Georgia | 26 | 15145 | 171.7 | 73.5% |
Terrell | Georgia | 24 | 16675 | 143.9 | 61.7% |
Thomas | Georgia | 49 | 31289 | 156.6 | 67.1% |
Tift | Georgia | 34 | 18599 | 182.8 | 78.3% |
Toombs | Georgia | 31 | 16952 | 182.9 | 78.3% |
Towns | Georgia | 13 | 4925 | 264.0 | 113.1% |
Treutlen | Georgia | 9 | 7632 | 117.9 | 50.5% |
Troup | Georgia | 84 | 43879 | 191.4 | 82.0% |
Turner | Georgia | 16 | 10846 | 147.5 | 63.2% |
Twiggs | Georgia | 5 | 9117 | 54.8 | 23.5% |
Union | Georgia | 14 | 7680 | 182.3 | 78.1% |
Upson | Georgia | 45 | 25064 | 179.5 | 76.9% |
Walker | Georgia | 83 | 31024 | 267.5 | 114.6% |
Walton | Georgia | 31 | 20777 | 149.2 | 63.9% |
Ware | Georgia | 58 | 27929 | 207.7 | 89.0% |
Warren | Georgia | 13 | 10236 | 127.0 | 54.4% |
Washington | Georgia | 34 | 24230 | 140.3 | 60.1% |
Wayne | Georgia | 21 | 13122 | 160.0 | 68.6% |
Webster | Georgia | 1 | 4726 | 21.2 | 9.1% |
Wheeler | Georgia | 17 | 8535 | 199.2 | 85.3% |
White | Georgia | 12 | 6417 | 187.0 | 80.1% |
Whitfield | Georgia | 56 | 26105 | 214.5 | 91.9% |
Wilcox | Georgia | 27 | 12755 | 211.7 | 90.7% |
Wilkes | Georgia | 9 | 15084 | 59.7 | 25.6% |
Wilkinson | Georgia | 12 | 11025 | 108.8 | 46.6% |
Worth | Georgia | 19 | 21374 | 88.9 | 38.1% |
Adair | Iowa | 34 | 13196 | 257.7 | 110.4% |
Adams | Iowa | 27 | 10156 | 265.9 | 113.9% |
Allamakee | Iowa | 30 | 17184 | 174.6 | 74.8% |
Appanoose | Iowa | 65 | 24245 | 268.1 | 114.8% |
Audubon | Iowa | 31 | 11790 | 262.9 | 112.6% |
Benton | Iowa | 54 | 22879 | 236.0 | 101.1% |
Black Hawk | Iowa | 201 | 79946 | 251.4 | 107.7% |
Boone | Iowa | 66 | 29782 | 221.6 | 94.9% |
Bremer | Iowa | 29 | 17932 | 161.7 | 69.3% |
Buchanan | Iowa | 33 | 20991 | 157.2 | 67.3% |
Buena Vista | Iowa | 35 | 19838 | 176.4 | 75.6% |
Butler | Iowa | 38 | 17986 | 211.3 | 90.5% |
Calhoun | Iowa | 43 | 17584 | 244.5 | 104.7% |
Carroll | Iowa | 50 | 22770 | 219.6 | 94.1% |
Cass | Iowa | 47 | 18647 | 252.1 | 108.0% |
Cedar | Iowa | 35 | 16884 | 207.3 | 88.8% |
Cerro Gordo | Iowa | 112 | 43845 | 255.4 | 109.4% |
Cherokee | Iowa | 37 | 19258 | 192.1 | 82.3% |
Chickasaw | Iowa | 30 | 15227 | 197.0 | 84.4% |
Clarke | Iowa | 20 | 10233 | 195.4 | 83.7% |
Clay | Iowa | 30 | 17762 | 168.9 | 72.3% |
Clayton | Iowa | 36 | 24334 | 147.9 | 63.4% |
Clinton | Iowa | 101 | 44722 | 225.8 | 96.7% |
Crawford | Iowa | 56 | 20538 | 272.7 | 116.8% |
Dallas | Iowa | 55 | 24649 | 223.1 | 95.6% |
Davis | Iowa | 27 | 11136 | 242.5 | 103.9% |
Decatur | Iowa | 47 | 14012 | 335.4 | 143.7% |
Delaware | Iowa | 35 | 18487 | 189.3 | 81.1% |
Des Moines | Iowa | 105 | 36804 | 285.3 | 122.2% |
Dickinson | Iowa | 23 | 12185 | 188.8 | 80.9% |
Dubuque | Iowa | 162 | 63768 | 254.0 | 108.8% |
Emmet | Iowa | 40 | 13406 | 298.4 | 127.8% |
Fayette | Iowa | 55 | 29151 | 188.7 | 80.8% |
Floyd | Iowa | 49 | 20169 | 242.9 | 104.1% |
Franklin | Iowa | 24 | 16379 | 146.5 | 62.8% |
Fremont | Iowa | 46 | 14645 | 314.1 | 134.5% |
Greene | Iowa | 46 | 16599 | 277.1 | 118.7% |
Grundy | Iowa | 34 | 13518 | 251.5 | 107.7% |
Guthrie | Iowa | 38 | 17210 | 220.8 | 94.6% |
Hamilton | Iowa | 46 | 19922 | 230.9 | 98.9% |
Hancock | Iowa | 26 | 15402 | 168.8 | 72.3% |
Hardin | Iowa | 32 | 22530 | 142.0 | 60.8% |
Harrison | Iowa | 45 | 22767 | 197.7 | 84.7% |
Henry | Iowa | 39 | 17994 | 216.7 | 92.8% |
Howard | Iowa | 29 | 13531 | 214.3 | 91.8% |
Humboldt | Iowa | 32 | 13459 | 237.8 | 101.8% |
Ida | Iowa | 23 | 11047 | 208.2 | 89.2% |
Iowa | Iowa | 33 | 17016 | 193.9 | 83.1% |
Jackson | Iowa | 27 | 19181 | 140.8 | 60.3% |
Jasper | Iowa | 64 | 31496 | 203.2 | 87.0% |
Jefferson | Iowa | 43 | 15762 | 272.8 | 116.9% |
Johnson | Iowa | 75 | 33191 | 226.0 | 96.8% |
Jones | Iowa | 33 | 19950 | 165.4 | 70.9% |
Keokuk | Iowa | 43 | 18406 | 233.6 | 100.1% |
Kossuth | Iowa | 69 | 26630 | 259.1 | 111.0% |
Lee | Iowa | 98 | 41074 | 238.6 | 102.2% |
Linn | Iowa | 213 | 89142 | 238.9 | 102.4% |
Louisa | Iowa | 25 | 11384 | 219.6 | 94.1% |
Lucas | Iowa | 21 | 14571 | 144.1 | 61.7% |
Lyon | Iowa | 27 | 15374 | 175.6 | 75.2% |
Madison | Iowa | 23 | 14525 | 158.3 | 67.8% |
Mahaska | Iowa | 54 | 26485 | 203.9 | 87.3% |
Marion | Iowa | 35 | 27019 | 129.5 | 55.5% |
Marshall | Iowa | 52 | 35406 | 146.9 | 62.9% |
Mills | Iowa | 31 | 15064 | 205.8 | 88.1% |
Mitchell | Iowa | 32 | 14121 | 226.6 | 97.1% |
Monona | Iowa | 41 | 18238 | 224.8 | 96.3% |
Monroe | Iowa | 32 | 14553 | 219.9 | 94.2% |
Montgomery | Iowa | 46 | 15697 | 293.0 | 125.5% |
Muscatine | Iowa | 64 | 31296 | 204.5 | 87.6% |
O'brien | Iowa | 42 | 19293 | 217.7 | 93.2% |
Osceola | Iowa | 13 | 10607 | 122.6 | 52.5% |
Page | Iowa | 56 | 24887 | 225.0 | 96.4% |
Palo Alto | Iowa | 44 | 16170 | 272.1 | 116.6% |
Plymouth | Iowa | 54 | 23502 | 229.8 | 98.4% |
Pocahontas | Iowa | 33 | 16266 | 202.9 | 86.9% |
Polk | Iowa | 450 | 195835 | 229.8 | 98.4% |
Pottawattamie | Iowa | 152 | 66756 | 227.7 | 97.5% |
Poweshiek | Iowa | 41 | 18758 | 218.6 | 93.6% |
Ringgold | Iowa | 28 | 11137 | 251.4 | 107.7% |
Sac | Iowa | 28 | 17639 | 158.7 | 68.0% |
Scott | Iowa | 168 | 84748 | 198.2 | 84.9% |
Shelby | Iowa | 29 | 16720 | 173.4 | 74.3% |
Sioux | Iowa | 66 | 27209 | 242.6 | 103.9% |
St.ory | Iowa | 95 | 33434 | 284.1 | 121.7% |
Tama | Iowa | 39 | 22428 | 173.9 | 74.5% |
Taylor | Iowa | 29 | 14258 | 203.4 | 87.1% |
Union | Iowa | 33 | 16280 | 202.7 | 86.8% |
Van Buren | Iowa | 31 | 12053 | 257.2 | 110.2% |
Wapello | Iowa | 99 | 44280 | 223.6 | 95.8% |
Warren | Iowa | 47 | 17695 | 265.6 | 113.8% |
Washington | Iowa | 54 | 20055 | 269.3 | 115.3% |
Wayne | Iowa | 31 | 13308 | 232.9 | 99.8% |
Webster | Iowa | 97 | 41521 | 233.6 | 100.1% |
Winnebago | Iowa | 33 | 13972 | 236.2 | 101.2% |
Winneshiek | Iowa | 30 | 22263 | 134.8 | 57.7% |
Woodbury | Iowa | 234 | 103627 | 225.8 | 96.7% |
Worth | Iowa | 28 | 11449 | 244.6 | 104.8% |
Wright | Iowa | 39 | 20038 | 194.6 | 83.4% |
Ada | Idaho | 119 | 50401 | 236.1 | 101.1% |
Adams | Idaho | 12 | 3407 | 352.2 | 150.9% |
Bannock | Idaho | 89 | 34759 | 256.0 | 109.7% |
Bear Lake | Idaho | 21 | 7911 | 265.5 | 113.7% |
Benewah | Idaho | 20 | 7332 | 272.8 | 116.8% |
Bingham | Idaho | 49 | 21044 | 232.8 | 99.7% |
Blaine | Idaho | 8 | 5295 | 151.1 | 64.7% |
Boise | Idaho | 9 | 2333 | 385.8 | 165.2% |
Bonner | Idaho | 44 | 15667 | 280.8 | 120.3% |
Bonneville | Idaho | 69 | 25697 | 268.5 | 115.0% |
Boundary | Idaho | 17 | 5987 | 283.9 | 121.6% |
Butte | Idaho | 4 | 1877 | 213.1 | 91.3% |
Camas | Idaho | 6 | 1360 | 441.2 | 189.0% |
Canyon | Idaho | 110 | 40987 | 268.4 | 115.0% |
Caribou | Idaho | 9 | 2284 | 394.0 | 168.8% |
Cassia | Idaho | 36 | 14430 | 249.5 | 106.9% |
Clark | Idaho | 5 | 1005 | 497.5 | 213.1% |
Clearwater | Idaho | 27 | 8243 | 327.6 | 140.3% |
Custer | Idaho | 10 | 3549 | 281.8 | 120.7% |
Elmore | Idaho | 15 | 5518 | 271.8 | 116.4% |
Franklin | Idaho | 17 | 10229 | 166.2 | 71.2% |
Fremont | Idaho | 23 | 10304 | 223.2 | 95.6% |
Gem | Idaho | 26 | 9544 | 272.4 | 116.7% |
Gooding | Idaho | 24 | 9257 | 259.3 | 111.1% |
Idaho | Idaho | 24 | 12691 | 189.1 | 81.0% |
Jefferson | Idaho | 20 | 10762 | 185.8 | 79.6% |
Jerome | Idaho | 46 | 9900 | 464.6 | 199.0% |
Kootenai | Idaho | 66 | 22283 | 296.2 | 126.9% |
Latah | Idaho | 54 | 18804 | 287.2 | 123.0% |
Lemhi | Idaho | 20 | 6521 | 306.7 | 131.4% |
Lewis | Idaho | 18 | 4666 | 385.8 | 165.2% |
Lincoln | Idaho | 12 | 4230 | 283.7 | 121.5% |
Madison | Idaho | 24 | 9186 | 261.3 | 111.9% |
Minidoka | Idaho | 26 | 9870 | 263.4 | 112.8% |
Nez Perce | Idaho | 63 | 18873 | 333.8 | 143.0% |
Oneida | Idaho | 14 | 5417 | 258.4 | 110.7% |
Owyhee | Idaho | 10 | 5652 | 176.9 | 75.8% |
Payette | Idaho | 23 | 9511 | 241.8 | 103.6% |
Power | Idaho | 15 | 3965 | 378.3 | 162.0% |
Shoshone | Idaho | 50 | 21230 | 235.5 | 100.9% |
Teton | Idaho | 11 | 3601 | 305.5 | 130.8% |
Twin Falls | Idaho | 86 | 36403 | 236.2 | 101.2% |
Valley | Idaho | 14 | 4035 | 347.0 | 148.6% |
Washington | Idaho | 26 | 8853 | 293.7 | 125.8% |
Adams | Illinois | 127 | 65229 | 194.7 | 83.4% |
Alexander | Illinois | 64 | 25496 | 251.0 | 107.5% |
Bond | Illinois | 48 | 14540 | 330.1 | 141.4% |
Boone | Illinois | 27 | 15202 | 177.6 | 76.1% |
Brown | Illinois | 25 | 8053 | 310.4 | 133.0% |
Bureau | Illinois | 85 | 37600 | 226.1 | 96.8% |
Calhoun | Illinois | 21 | 8207 | 255.9 | 109.6% |
Carroll | Illinois | 32 | 17987 | 177.9 | 76.2% |
Cass | Illinois | 37 | 16425 | 225.3 | 96.5% |
Champaign | Illinois | 181 | 70578 | 256.5 | 109.9% |
Christian | Illinois | 81 | 38564 | 210.0 | 90.0% |
Clark | Illinois | 35 | 18842 | 185.8 | 79.6% |
Clay | Illinois | 50 | 18947 | 263.9 | 113.0% |
Clinton | Illinois | 45 | 22912 | 196.4 | 84.1% |
Coles | Illinois | 95 | 38470 | 246.9 | 105.8% |
Cook | Illinois | 9721 | 4063342 | 239.2 | 102.5% |
Crawford | Illinois | 61 | 21294 | 286.5 | 122.7% |
Cumberland | Illinois | 19 | 11698 | 162.4 | 69.6% |
De Kalb | Illinois | 117 | 34388 | 340.2 | 145.7% |
De Witt | Illinois | 42 | 18244 | 230.2 | 98.6% |
Douglas | Illinois | 48 | 17590 | 272.9 | 116.9% |
Du Page | Illinois | 253 | 103480 | 244.5 | 104.7% |
Edgar | Illinois | 55 | 24430 | 225.1 | 96.4% |
Edwards | Illinois | 11 | 8974 | 122.6 | 52.5% |
Effingham | Illinois | 67 | 22034 | 304.1 | 130.3% |
Fayette | Illinois | 66 | 29159 | 226.3 | 97.0% |
Ford | Illinois | 31 | 15007 | 206.6 | 88.5% |
Franklin | Illinois | 129 | 53137 | 242.8 | 104.0% |
Fulton | Illinois | 68 | 44627 | 152.4 | 65.3% |
Gallatin | Illinois | 23 | 11414 | 201.5 | 86.3% |
Greene | Illinois | 43 | 20292 | 211.9 | 90.8% |
Grundy | Illinois | 44 | 18398 | 239.2 | 102.4% |
Hamilton | Illinois | 45 | 13454 | 334.5 | 143.3% |
Hancock | Illinois | 65 | 26297 | 247.2 | 105.9% |
Hardin | Illinois | 28 | 7759 | 360.9 | 154.6% |
Henderson | Illinois | 18 | 8949 | 201.1 | 86.2% |
Henry | Illinois | 74 | 43798 | 169.0 | 72.4% |
Iroquois | Illinois | 61 | 32496 | 187.7 | 80.4% |
Jackson | Illinois | 89 | 37920 | 234.7 | 100.5% |
Jasper | Illinois | 42 | 13431 | 312.7 | 133.9% |
Jefferson | Illinois | 118 | 34375 | 343.3 | 147.0% |
Jersey | Illinois | 33 | 13636 | 242.0 | 103.7% |
Jo Daviess | Illinois | 49 | 19989 | 245.1 | 105.0% |
Johnson | Illinois | 30 | 10727 | 279.7 | 119.8% |
Kane | Illinois | 304 | 130206 | 233.5 | 100.0% |
Kankakee | Illinois | 131 | 60877 | 215.2 | 92.2% |
Kendall | Illinois | 26 | 11105 | 234.1 | 100.3% |
Knox | Illinois | 113 | 52250 | 216.3 | 92.6% |
Lake | Illinois | 257 | 121094 | 212.2 | 90.9% |
La Salle | Illinois | 250 | 97801 | 255.6 | 109.5% |
Lawrence | Illinois | 62 | 21075 | 294.2 | 126.0% |
Lee | Illinois | 84 | 34604 | 242.7 | 104.0% |
Livingston | Illinois | 87 | 38838 | 224.0 | 96.0% |
Logan | Illinois | 71 | 29438 | 241.2 | 103.3% |
Macon | Illinois | 176 | 84693 | 207.8 | 89.0% |
Macoupin | Illinois | 102 | 46304 | 220.3 | 94.4% |
Madison | Illinois | 346 | 149349 | 231.7 | 99.2% |
Marion | Illinois | 102 | 47989 | 212.5 | 91.0% |
Marshall | Illinois | 28 | 13179 | 212.5 | 91.0% |
Mason | Illinois | 46 | 15358 | 299.5 | 128.3% |
Massac | Illinois | 33 | 14937 | 220.9 | 94.6% |
Mcdonough | Illinois | 65 | 26944 | 241.2 | 103.3% |
Mchenry | Illinois | 89 | 37311 | 238.5 | 102.2% |
Mclean | Illinois | 169 | 73930 | 228.6 | 97.9% |
Menard | Illinois | 15 | 10663 | 140.7 | 60.3% |
Mercer | Illinois | 44 | 17701 | 248.6 | 106.5% |
Monroe | Illinois | 20 | 12754 | 156.8 | 67.2% |
Montgomery | Illinois | 87 | 34499 | 252.2 | 108.0% |
Morgan | Illinois | 80 | 36378 | 219.9 | 94.2% |
Moultrie | Illinois | 32 | 13477 | 237.4 | 101.7% |
Ogle | Illinois | 65 | 29869 | 217.6 | 93.2% |
Peoria | Illinois | 343 | 153374 | 223.6 | 95.8% |
Perry | Illinois | 46 | 23438 | 196.3 | 84.1% |
Piatt | Illinois | 39 | 14659 | 266.0 | 114.0% |
Pike | Illinois | 61 | 25340 | 240.7 | 103.1% |
Pope | Illinois | 15 | 7999 | 187.5 | 80.3% |
Pulaski | Illinois | 26 | 15875 | 163.8 | 70.2% |
Putnam | Illinois | 12 | 5289 | 226.9 | 97.2% |
Randolph | Illinois | 65 | 33608 | 193.4 | 82.8% |
Richland | Illinois | 43 | 17137 | 250.9 | 107.5% |
Rock Island | Illinois | 267 | 113323 | 235.6 | 100.9% |
Saline | Illinois | 80 | 38066 | 210.2 | 90.0% |
Sangamon | Illinois | 271 | 117912 | 229.8 | 98.4% |
Schuyler | Illinois | 22 | 11430 | 192.5 | 82.4% |
Scott | Illinois | 24 | 8176 | 293.5 | 125.7% |
Shelby | Illinois | 67 | 26290 | 254.8 | 109.2% |
St.ark | Illinois | 20 | 8881 | 225.2 | 96.5% |
St Clair | Illinois | 349 | 166899 | 209.1 | 89.6% |
St.ephenson | Illinois | 82 | 40646 | 201.7 | 86.4% |
Tazewell | Illinois | 101 | 58362 | 173.1 | 74.1% |
Union | Illinois | 42 | 21528 | 195.1 | 83.6% |
Vermilion | Illinois | 184 | 86791 | 212.0 | 90.8% |
Wabash | Illinois | 37 | 13724 | 269.6 | 115.5% |
Warren | Illinois | 45 | 21286 | 211.4 | 90.6% |
Washington | Illinois | 24 | 15801 | 151.9 | 65.1% |
Wayne | Illinois | 60 | 22092 | 271.6 | 116.3% |
White | Illinois | 53 | 20027 | 264.6 | 113.4% |
Whiteside | Illinois | 116 | 43338 | 267.7 | 114.7% |
Will | Illinois | 271 | 114210 | 237.3 | 101.6% |
Williamson | Illinois | 111 | 51424 | 215.9 | 92.5% |
Winnebago | Illinois | 285 | 121178 | 235.2 | 100.7% |
Woodford | Illinois | 50 | 19124 | 261.5 | 112.0% |
Adams | Indiana | 54 | 21254 | 254.1 | 108.8% |
Allen | Indiana | 291 | 155084 | 187.6 | 80.4% |
Bartholomew | Indiana | 68 | 28276 | 240.5 | 103.0% |
Benton | Indiana | 49 | 11117 | 440.8 | 188.8% |
Blackford | Indiana | 35 | 13783 | 253.9 | 108.8% |
Boone | Indiana | 41 | 22081 | 185.7 | 79.5% |
Brown | Indiana | 9 | 6189 | 145.4 | 62.3% |
Carroll | Indiana | 41 | 15410 | 266.1 | 114.0% |
Cass | Indiana | 88 | 36908 | 238.4 | 102.1% |
Clark | Indiana | 91 | 31020 | 293.4 | 125.7% |
Clay | Indiana | 61 | 25365 | 240.5 | 103.0% |
Clinton | Indiana | 63 | 28411 | 221.7 | 95.0% |
Crawford | Indiana | 31 | 10171 | 304.8 | 130.6% |
Daviess | Indiana | 59 | 26163 | 225.5 | 96.6% |
Dearborn | Indiana | 62 | 23053 | 268.9 | 115.2% |
Decatur | Indiana | 35 | 17722 | 197.5 | 84.6% |
De Kalb | Indiana | 47 | 24756 | 189.9 | 81.3% |
Delaware | Indiana | 158 | 74963 | 210.8 | 90.3% |
Dubois | Indiana | 43 | 22579 | 190.4 | 81.6% |
Elkhart | Indiana | 181 | 72634 | 249.2 | 106.7% |
Fayette | Indiana | 41 | 19411 | 211.2 | 90.5% |
Floyd | Indiana | 78 | 35061 | 222.5 | 95.3% |
Fountain | Indiana | 41 | 18299 | 224.1 | 96.0% |
Franklin | Indiana | 27 | 14412 | 187.3 | 80.2% |
Fulton | Indiana | 34 | 15577 | 218.3 | 93.5% |
Gibson | Indiana | 66 | 30709 | 214.9 | 92.1% |
Grant | Indiana | 113 | 55813 | 202.5 | 86.7% |
Greene | Indiana | 65 | 31330 | 207.5 | 88.9% |
Hamilton | Indiana | 48 | 24614 | 195.0 | 83.5% |
Hancock | Indiana | 33 | 17302 | 190.7 | 81.7% |
Harrison | Indiana | 53 | 17106 | 309.8 | 132.7% |
Hendricks | Indiana | 36 | 20151 | 178.7 | 76.5% |
Henry | Indiana | 87 | 40208 | 216.4 | 92.7% |
Howard | Indiana | 116 | 47752 | 242.9 | 104.1% |
Huntington | Indiana | 67 | 29931 | 223.8 | 95.9% |
Jackson | Indiana | 72 | 26612 | 270.6 | 115.9% |
Jasper | Indiana | 34 | 14397 | 236.2 | 101.2% |
Jay | Indiana | 56 | 22601 | 247.8 | 106.1% |
Jefferson | Indiana | 37 | 19912 | 185.8 | 79.6% |
Jennings | Indiana | 35 | 13680 | 255.8 | 109.6% |
Johnson | Indiana | 52 | 22493 | 231.2 | 99.0% |
Knox | Indiana | 109 | 43973 | 247.9 | 106.2% |
Kosciusko | Indiana | 46 | 29561 | 155.6 | 66.7% |
Lagrange | Indiana | 24 | 14352 | 167.2 | 71.6% |
Lake | Indiana | 815 | 293195 | 278.0 | 119.1% |
La Porte | Indiana | 183 | 63660 | 287.5 | 123.1% |
Lawrence | Indiana | 77 | 35045 | 219.7 | 94.1% |
Madison | Indiana | 228 | 88575 | 257.4 | 110.3% |
Marion | Indiana | 1055 | 460926 | 228.9 | 98.0% |
Marshall | Indiana | 63 | 25935 | 242.9 | 104.1% |
Martin | Indiana | 23 | 10300 | 223.3 | 95.7% |
Miami | Indiana | 66 | 27926 | 236.3 | 101.2% |
Monroe | Indiana | 92 | 36534 | 251.8 | 107.9% |
Montgomery | Indiana | 70 | 27231 | 257.1 | 110.1% |
Morgan | Indiana | 57 | 19801 | 287.9 | 123.3% |
Newton | Indiana | 31 | 10775 | 287.7 | 123.2% |
Noble | Indiana | 49 | 22776 | 215.1 | 92.2% |
Ohio | Indiana | 9 | 3782 | 238.0 | 101.9% |
Orange | Indiana | 38 | 17311 | 219.5 | 94.0% |
Owen | Indiana | 16 | 12090 | 132.3 | 56.7% |
Parke | Indiana | 34 | 17358 | 195.9 | 83.9% |
Perry | Indiana | 50 | 17770 | 281.4 | 120.5% |
Pike | Indiana | 30 | 17045 | 176.0 | 75.4% |
Porter | Indiana | 49 | 27836 | 176.0 | 75.4% |
Posey | Indiana | 56 | 19183 | 291.9 | 125.0% |
Pulaski | Indiana | 43 | 12056 | 356.7 | 152.8% |
Putnam | Indiana | 49 | 20839 | 235.1 | 100.7% |
Randolph | Indiana | 59 | 26766 | 220.4 | 94.4% |
Ripley | Indiana | 39 | 18898 | 206.4 | 88.4% |
Rush | Indiana | 31 | 18927 | 163.8 | 70.2% |
Scott | Indiana | 40 | 8978 | 445.5 | 190.8% |
Shelby | Indiana | 56 | 25953 | 215.8 | 92.4% |
Spencer | Indiana | 49 | 16211 | 302.3 | 129.5% |
St.arke | Indiana | 19 | 12258 | 155.0 | 66.4% |
St.euben | Indiana | 37 | 13740 | 269.3 | 115.3% |
St Joseph | Indiana | 430 | 161823 | 265.7 | 113.8% |
Sullivan | Indiana | 63 | 27014 | 233.2 | 99.9% |
Switzerland | Indiana | 16 | 8167 | 195.9 | 83.9% |
Tippecanoe | Indiana | 146 | 51020 | 286.2 | 122.6% |
Tipton | Indiana | 29 | 15135 | 191.6 | 82.1% |
Union | Indiana | 15 | 6017 | 249.3 | 106.8% |
Vanderburgh | Indiana | 298 | 130783 | 227.9 | 97.6% |
Vermillion | Indiana | 50 | 21787 | 229.5 | 98.3% |
Vigo | Indiana | 211 | 99709 | 211.6 | 90.6% |
Wabash | Indiana | 56 | 26601 | 210.5 | 90.2% |
Warren | Indiana | 25 | 9055 | 276.1 | 118.3% |
Warrick | Indiana | 54 | 19435 | 277.8 | 119.0% |
Washington | Indiana | 66 | 17008 | 388.1 | 166.2% |
Wayne | Indiana | 134 | 59229 | 226.2 | 96.9% |
Wells | Indiana | 28 | 19099 | 146.6 | 62.8% |
White | Indiana | 36 | 17037 | 211.3 | 90.5% |
Whitley | Indiana | 38 | 17001 | 223.5 | 95.7% |
Allen | Kansas | 37 | 19874 | 186.2 | 79.7% |
Anderson | Kansas | 26 | 11658 | 223.0 | 95.5% |
Atchison | Kansas | 45 | 22222 | 202.5 | 86.7% |
Barber | Kansas | 35 | 9073 | 385.8 | 165.2% |
Barton | Kansas | 64 | 25010 | 255.9 | 109.6% |
Bourbon | Kansas | 50 | 20944 | 238.7 | 102.3% |
Brown | Kansas | 45 | 17395 | 258.7 | 110.8% |
Butler | Kansas | 100 | 32013 | 312.4 | 133.8% |
Chase | Kansas | 15 | 6345 | 236.4 | 101.3% |
Chautauqua | Kansas | 28 | 9233 | 303.3 | 129.9% |
Cherokee | Kansas | 77 | 29817 | 258.2 | 110.6% |
Cheyenne | Kansas | 10 | 6221 | 160.7 | 68.9% |
Clark | Kansas | 12 | 4081 | 294.0 | 126.0% |
Clay | Kansas | 29 | 13281 | 218.4 | 93.5% |
Cloud | Kansas | 39 | 17247 | 226.1 | 96.9% |
Coffey | Kansas | 20 | 12278 | 162.9 | 69.8% |
Comanche | Kansas | 13 | 4412 | 294.7 | 126.2% |
Cowley | Kansas | 85 | 38139 | 222.9 | 95.5% |
Crawford | Kansas | 123 | 44191 | 278.3 | 119.2% |
Decatur | Kansas | 23 | 7434 | 309.4 | 132.5% |
Dickinson | Kansas | 56 | 22929 | 244.2 | 104.6% |
Doniphan | Kansas | 30 | 12936 | 231.9 | 99.3% |
Douglas | Kansas | 74 | 25171 | 294.0 | 125.9% |
Edwards | Kansas | 21 | 6377 | 329.3 | 141.1% |
Elk | Kansas | 19 | 8180 | 232.3 | 99.5% |
Ellis | Kansas | 41 | 17508 | 234.2 | 100.3% |
Ellsworth | Kansas | 18 | 9855 | 182.6 | 78.2% |
Finney | Kansas | 32 | 10092 | 317.1 | 135.8% |
Ford | Kansas | 55 | 17254 | 318.8 | 136.5% |
Franklin | Kansas | 34 | 20889 | 162.8 | 69.7% |
Geary | Kansas | 65 | 15222 | 427.0 | 182.9% |
Gove | Kansas | 12 | 4793 | 250.4 | 107.2% |
Graham | Kansas | 14 | 6071 | 230.6 | 98.8% |
Grant | Kansas | 5 | 1946 | 256.9 | 110.1% |
Gray | Kansas | 16 | 4773 | 335.2 | 143.6% |
Greeley | Kansas | 10 | 1638 | 610.5 | 261.5% |
Greenwood | Kansas | 41 | 16495 | 248.6 | 106.5% |
Hamilton | Kansas | 11 | 2645 | 415.9 | 178.1% |
Harper | Kansas | 21 | 12068 | 174.0 | 74.5% |
Harvey | Kansas | 67 | 21712 | 308.6 | 132.2% |
Haskell | Kansas | 10 | 2088 | 478.9 | 205.1% |
Hodgeman | Kansas | 8 | 3535 | 226.3 | 96.9% |
Jackson | Kansas | 43 | 13382 | 321.3 | 137.6% |
Jefferson | Kansas | 35 | 12718 | 275.2 | 117.9% |
Jewell | Kansas | 30 | 11970 | 250.6 | 107.4% |
Johnson | Kansas | 68 | 33327 | 204.0 | 87.4% |
Kearny | Kansas | 6 | 2525 | 237.6 | 101.8% |
Kingman | Kansas | 37 | 12001 | 308.3 | 132.1% |
Kiowa | Kansas | 10 | 5112 | 195.6 | 83.8% |
Labette | Kansas | 79 | 30352 | 260.3 | 111.5% |
Lane | Kansas | 4 | 2821 | 141.8 | 60.7% |
Leavenworth | Kansas | 93 | 41112 | 226.2 | 96.9% |
Lincoln | Kansas | 28 | 8338 | 335.8 | 143.8% |
Linn | Kansas | 23 | 11969 | 192.2 | 82.3% |
Logan | Kansas | 21 | 3688 | 569.4 | 243.9% |
Lyon | Kansas | 93 | 26424 | 352.0 | 150.8% |
Marion | Kansas | 37 | 18951 | 195.2 | 83.6% |
Marshall | Kansas | 36 | 20986 | 171.5 | 73.5% |
Mcpherson | Kansas | 58 | 24152 | 240.1 | 102.9% |
Meade | Kansas | 13 | 5522 | 235.4 | 100.8% |
Miami | Kansas | 31 | 19489 | 159.1 | 68.1% |
Mitchell | Kansas | 24 | 11339 | 211.7 | 90.7% |
Montgomery | Kansas | 138 | 49729 | 277.5 | 118.9% |
Morris | Kansas | 45 | 10363 | 434.2 | 186.0% |
Morton | Kansas | 4 | 2186 | 183.0 | 78.4% |
Nemaha | Kansas | 31 | 16761 | 185.0 | 79.2% |
Neosho | Kansas | 48 | 22210 | 216.1 | 92.6% |
Ness | Kansas | 16 | 6864 | 233.1 | 99.8% |
Norton | Kansas | 22 | 9831 | 223.8 | 95.9% |
Osage | Kansas | 30 | 15118 | 198.4 | 85.0% |
Osborne | Kansas | 28 | 9835 | 284.7 | 121.9% |
Ottawa | Kansas | 24 | 9224 | 260.2 | 111.5% |
Pawnee | Kansas | 18 | 10300 | 174.8 | 74.9% |
Phillips | Kansas | 20 | 10435 | 191.7 | 82.1% |
Pottawatomie | Kansas | 37 | 14015 | 264.0 | 113.1% |
Pratt | Kansas | 35 | 12348 | 283.4 | 121.4% |
Rawlins | Kansas | 10 | 6618 | 151.1 | 64.7% |
Reno | Kansas | 109 | 52165 | 209.0 | 89.5% |
Republic | Kansas | 28 | 13124 | 213.3 | 91.4% |
Rice | Kansas | 44 | 17213 | 255.6 | 109.5% |
Riley | Kansas | 62 | 20617 | 300.7 | 128.8% |
Rooks | Kansas | 20 | 8497 | 235.4 | 100.8% |
Rush | Kansas | 17 | 8285 | 205.2 | 87.9% |
Russell | Kansas | 40 | 13464 | 297.1 | 127.3% |
Saline | Kansas | 70 | 29535 | 237.0 | 101.5% |
Scott | Kansas | 9 | 3773 | 238.5 | 102.2% |
Sedgwick | Kansas | 397 | 143311 | 277.0 | 118.7% |
Seward | Kansas | 12 | 6540 | 183.5 | 78.6% |
Shawnee | Kansas | 172 | 91247 | 188.5 | 80.7% |
Sheridan | Kansas | 11 | 5312 | 207.1 | 88.7% |
Sherman | Kansas | 16 | 6421 | 249.2 | 106.7% |
Smith | Kansas | 31 | 10582 | 293.0 | 125.5% |
St.afford | Kansas | 25 | 10487 | 238.4 | 102.1% |
St.anton | Kansas | 2 | 1443 | 138.6 | 59.4% |
St.evens | Kansas | 13 | 3193 | 407.1 | 174.4% |
Sumner | Kansas | 70 | 26163 | 267.6 | 114.6% |
Thomas | Kansas | 22 | 6425 | 342.4 | 146.7% |
Trego | Kansas | 19 | 5822 | 326.3 | 139.8% |
Wabaunsee | Kansas | 12 | 9219 | 130.2 | 55.8% |
Wallace | Kansas | 3 | 2216 | 135.4 | 58.0% |
Washington | Kansas | 40 | 15921 | 251.2 | 107.6% |
Wichita | Kansas | 23 | 2185 | 1052.6 | 450.9% |
Wilson | Kansas | 32 | 17723 | 180.6 | 77.3% |
Woodson | Kansas | 26 | 8014 | 324.4 | 139.0% |
Wyandotte | Kansas | 364 | 145071 | 250.9 | 107.5% |
Adair | Kentucky | 45 | 18566 | 242.4 | 103.8% |
Allen | Kentucky | 34 | 15496 | 219.4 | 94.0% |
Anderson | Kentucky | 27 | 8936 | 302.1 | 129.4% |
Ballard | Kentucky | 27 | 9480 | 284.8 | 122.0% |
Barren | Kentucky | 52 | 27559 | 188.7 | 80.8% |
Bath | Kentucky | 29 | 11451 | 253.3 | 108.5% |
Bell | Kentucky | 91 | 43812 | 207.7 | 89.0% |
Boone | Kentucky | 26 | 10820 | 240.3 | 102.9% |
Bourbon | Kentucky | 33 | 17932 | 184.0 | 78.8% |
Boyd | Kentucky | 118 | 45938 | 256.9 | 110.0% |
Boyle | Kentucky | 39 | 17075 | 228.4 | 97.8% |
Bracken | Kentucky | 21 | 9389 | 223.7 | 95.8% |
Breathitt | Kentucky | 78 | 23946 | 325.7 | 139.5% |
Breckinridge | Kentucky | 51 | 17744 | 287.4 | 123.1% |
Bullitt | Kentucky | 23 | 9511 | 241.8 | 103.6% |
Butler | Kentucky | 33 | 14371 | 229.6 | 98.4% |
Caldwell | Kentucky | 23 | 14499 | 158.6 | 67.9% |
Calloway | Kentucky | 39 | 19041 | 204.8 | 87.7% |
Campbell | Kentucky | 174 | 71918 | 241.9 | 103.6% |
Carlisle | Kentucky | 25 | 7650 | 326.8 | 140.0% |
Carroll | Kentucky | 24 | 8657 | 277.2 | 118.8% |
Carter | Kentucky | 60 | 25545 | 234.9 | 100.6% |
Casey | Kentucky | 44 | 19962 | 220.4 | 94.4% |
Christian | Kentucky | 48 | 36129 | 132.9 | 56.9% |
Clark | Kentucky | 46 | 17988 | 255.7 | 109.5% |
Clay | Kentucky | 68 | 23901 | 284.5 | 121.9% |
Clinton | Kentucky | 24 | 10279 | 233.5 | 100.0% |
Crittenden | Kentucky | 29 | 12115 | 239.4 | 102.5% |
Cumberland | Kentucky | 35 | 11923 | 293.6 | 125.7% |
Daviess | Kentucky | 129 | 52335 | 246.5 | 105.6% |
Edmonson | Kentucky | 27 | 11344 | 238.0 | 102.0% |
Elliott | Kentucky | 25 | 8713 | 286.9 | 122.9% |
Estill | Kentucky | 51 | 17978 | 283.7 | 121.5% |
Fayette | Kentucky | 206 | 78899 | 261.1 | 111.8% |
Fleming | Kentucky | 38 | 13327 | 285.1 | 122.1% |
Floyd | Kentucky | 117 | 52986 | 220.8 | 94.6% |
Franklin | Kentucky | 46 | 23308 | 197.4 | 84.5% |
Fulton | Kentucky | 34 | 15413 | 220.6 | 94.5% |
Gallatin | Kentucky | 9 | 4307 | 209.0 | 89.5% |
Garrard | Kentucky | 23 | 11910 | 193.1 | 82.7% |
Grant | Kentucky | 17 | 9876 | 172.1 | 73.7% |
Graves | Kentucky | 71 | 31763 | 223.5 | 95.7% |
Grayson | Kentucky | 49 | 17562 | 279.0 | 119.5% |
Green | Kentucky | 23 | 12321 | 186.7 | 80.0% |
Greenup | Kentucky | 61 | 24917 | 244.8 | 104.9% |
Hancock | Kentucky | 10 | 6807 | 146.9 | 62.9% |
Hardin | Kentucky | 80 | 29108 | 274.8 | 117.7% |
Harlan | Kentucky | 183 | 75275 | 243.1 | 104.1% |
Harrison | Kentucky | 31 | 15124 | 205.0 | 87.8% |
Hart | Kentucky | 36 | 17239 | 208.8 | 89.5% |
Henderson | Kentucky | 70 | 27020 | 259.1 | 111.0% |
Henry | Kentucky | 30 | 12220 | 245.5 | 105.2% |
Hickman | Kentucky | 18 | 9142 | 196.9 | 84.3% |
Hopkins | Kentucky | 93 | 37789 | 246.1 | 105.4% |
Jackson | Kentucky | 34 | 16339 | 208.1 | 89.1% |
Jefferson | Kentucky | 896 | 385392 | 232.5 | 99.6% |
Jessamine | Kentucky | 25 | 12174 | 205.4 | 88.0% |
Johnson | Kentucky | 73 | 25771 | 283.3 | 121.3% |
Kenton | Kentucky | 225 | 93139 | 241.6 | 103.5% |
Knott | Kentucky | 68 | 20007 | 339.9 | 145.6% |
Knox | Kentucky | 81 | 31029 | 261.0 | 111.8% |
Larue | Kentucky | 22 | 9622 | 228.6 | 97.9% |
Laurel | Kentucky | 65 | 25640 | 253.5 | 108.6% |
Lawrence | Kentucky | 42 | 17275 | 243.1 | 104.1% |
Lee | Kentucky | 42 | 10860 | 386.7 | 165.7% |
Leslie | Kentucky | 40 | 14981 | 267.0 | 114.4% |
Letcher | Kentucky | 119 | 40592 | 293.2 | 125.6% |
Lewis | Kentucky | 48 | 15686 | 306.0 | 131.1% |
Lincoln | Kentucky | 36 | 19859 | 181.3 | 77.7% |
Livingston | Kentucky | 22 | 9127 | 241.0 | 103.3% |
Logan | Kentucky | 45 | 23345 | 192.8 | 82.6% |
Lyon | Kentucky | 15 | 9067 | 165.4 | 70.9% |
Madison | Kentucky | 54 | 28541 | 189.2 | 81.0% |
Magoffin | Kentucky | 38 | 17490 | 217.3 | 93.1% |
Marion | Kentucky | 32 | 16913 | 189.2 | 81.0% |
Marshall | Kentucky | 43 | 16602 | 259.0 | 110.9% |
Martin | Kentucky | 27 | 10970 | 246.1 | 105.4% |
Mason | Kentucky | 51 | 19066 | 267.5 | 114.6% |
Mccracken | Kentucky | 102 | 48534 | 210.2 | 90.0% |
Mccreary | Kentucky | 31 | 16451 | 188.4 | 80.7% |
Mclean | Kentucky | 27 | 11446 | 235.9 | 101.0% |
Meade | Kentucky | 23 | 8827 | 260.6 | 111.6% |
Menifee | Kentucky | 16 | 5691 | 281.1 | 120.4% |
Mercer | Kentucky | 67 | 14629 | 458.0 | 196.2% |
Metcalfe | Kentucky | 17 | 10853 | 156.6 | 67.1% |
Monroe | Kentucky | 36 | 14070 | 255.9 | 109.6% |
Montgomery | Kentucky | 30 | 12280 | 244.3 | 104.6% |
Morgan | Kentucky | 43 | 16827 | 255.5 | 109.5% |
Muhlenberg | Kentucky | 81 | 37554 | 215.7 | 92.4% |
Nelson | Kentucky | 37 | 18004 | 205.5 | 88.0% |
Nicholas | Kentucky | 20 | 8617 | 232.1 | 99.4% |
Ohio | Kentucky | 43 | 24421 | 176.1 | 75.4% |
Oldham | Kentucky | 14 | 10716 | 130.6 | 56.0% |
Owen | Kentucky | 17 | 10942 | 155.4 | 66.6% |
Owsley | Kentucky | 23 | 8957 | 256.8 | 110.0% |
Pendleton | Kentucky | 19 | 10392 | 182.8 | 78.3% |
Perry | Kentucky | 144 | 47828 | 301.1 | 129.0% |
Pike | Kentucky | 176 | 71122 | 247.5 | 106.0% |
Powell | Kentucky | 20 | 7671 | 260.7 | 111.7% |
Pulaski | Kentucky | 119 | 39863 | 298.5 | 127.9% |
Robertson | Kentucky | 6 | 3419 | 175.5 | 75.2% |
Rockcastle | Kentucky | 40 | 17165 | 233.0 | 99.8% |
Rowan | Kentucky | 49 | 12734 | 384.8 | 164.8% |
Russell | Kentucky | 30 | 13615 | 220.3 | 94.4% |
Scott | Kentucky | 38 | 14314 | 265.5 | 113.7% |
Shelby | Kentucky | 37 | 17759 | 208.3 | 89.2% |
Simpson | Kentucky | 19 | 11752 | 161.7 | 69.3% |
Spencer | Kentucky | 15 | 6757 | 222.0 | 95.1% |
Taylor | Kentucky | 29 | 13556 | 213.9 | 91.6% |
Todd | Kentucky | 18 | 14234 | 126.5 | 54.2% |
Trigg | Kentucky | 19 | 12784 | 148.6 | 63.7% |
Trimble | Kentucky | 11 | 5601 | 196.4 | 84.1% |
Union | Kentucky | 38 | 17411 | 218.3 | 93.5% |
Warren | Kentucky | 84 | 36631 | 229.3 | 98.2% |
Washington | Kentucky | 28 | 12965 | 216.0 | 92.5% |
Wayne | Kentucky | 40 | 17204 | 232.5 | 99.6% |
Webster | Kentucky | 34 | 19198 | 177.1 | 75.9% |
Whitley | Kentucky | 102 | 33186 | 307.4 | 131.7% |
Wolfe | Kentucky | 21 | 9997 | 210.1 | 90.0% |
Woodford | Kentucky | 30 | 11847 | 253.2 | 108.5% |
Acadia | Louisiana | 86 | 46260 | 185.9 | 79.6% |
Allen | Louisiana | 31 | 17540 | 176.7 | 75.7% |
Ascension | Louisiana | 31 | 21215 | 146.1 | 62.6% |
Assumption | Louisiana | 23 | 18541 | 124.0 | 53.1% |
Avoyelles | Louisiana | 74 | 39256 | 188.5 | 80.7% |
Beauregard | Louisiana | 27 | 14847 | 181.9 | 77.9% |
Bienville | Louisiana | 36 | 23933 | 150.4 | 64.4% |
Bossier | Louisiana | 29 | 33162 | 87.4 | 37.5% |
Caddo | Louisiana | 320 | 150203 | 213.0 | 91.3% |
Calcasieu | Louisiana | 135 | 56506 | 238.9 | 102.3% |
Caldwell | Louisiana | 22 | 12046 | 182.6 | 78.2% |
Cameron | Louisiana | 17 | 7203 | 236.0 | 101.1% |
Catahoula | Louisiana | 30 | 14618 | 205.2 | 87.9% |
Claiborne | Louisiana | 54 | 29855 | 180.9 | 77.5% |
Concordia | Louisiana | 12 | 14562 | 82.4 | 35.3% |
Desoto | Louisiana | 44 | 31803 | 138.4 | 59.3% |
East Baton Rouge | Louisiana | 150 | 88415 | 169.7 | 72.7% |
East Carroll | Louisiana | 18 | 19023 | 94.6 | 40.5% |
East Feliciana | Louisiana | 11 | 18039 | 61.0 | 26.1% |
Evangeline | Louisiana | 34 | 30497 | 111.5 | 47.8% |
Franklin | Louisiana | 50 | 32382 | 154.4 | 66.1% |
Grant | Louisiana | 35 | 15933 | 219.7 | 94.1% |
Iberia | Louisiana | 32 | 37183 | 86.1 | 36.9% |
Iberville | Louisiana | 38 | 27721 | 137.1 | 58.7% |
Jackson | Louisiana | 48 | 17807 | 269.6 | 115.5% |
Jefferson | Louisiana | 89 | 50427 | 176.5 | 75.6% |
Jefferson Davis | Louisiana | 44 | 24191 | 181.9 | 77.9% |
Lafayette | Louisiana | 81 | 43941 | 184.3 | 79.0% |
Lafourche | Louisiana | 57 | 38615 | 147.6 | 63.2% |
La Salle | Louisiana | 29 | 10959 | 264.6 | 113.4% |
Lincoln | Louisiana | 41 | 24790 | 165.4 | 70.8% |
Livingston | Louisiana | 32 | 17790 | 179.9 | 77.0% |
Madison | Louisiana | 18 | 18443 | 97.6 | 41.8% |
Morehouse | Louisiana | 40 | 27571 | 145.1 | 62.1% |
Natchitoches | Louisiana | 71 | 40997 | 173.2 | 74.2% |
Orleans | Louisiana | 773 | 494537 | 156.3 | 67.0% |
Ouachita | Louisiana | 114 | 59168 | 192.7 | 82.5% |
Plaquemines | Louisiana | 14 | 12318 | 113.7 | 48.7% |
Pointe Coupee | Louisiana | 30 | 24004 | 125.0 | 53.5% |
Rapides | Louisiana | 146 | 73370 | 199.0 | 85.2% |
Red River | Louisiana | 34 | 15881 | 214.1 | 91.7% |
Richland | Louisiana | 52 | 28829 | 180.4 | 77.3% |
Sabine | Louisiana | 68 | 23586 | 288.3 | 123.5% |
St Bernard | Louisiana | 11 | 7280 | 151.1 | 64.7% |
St Charles | Louisiana | 16 | 12321 | 129.9 | 55.6% |
St Helena | Louisiana | 4 | 9542 | 41.9 | 18.0% |
St James | Louisiana | 27 | 16596 | 162.7 | 69.7% |
St John The Baptist | Louisiana | 23 | 14766 | 155.8 | 66.7% |
St Landry | Louisiana | 81 | 71481 | 113.3 | 48.5% |
St Martin | Louisiana | 32 | 26394 | 121.2 | 51.9% |
St Mary | Louisiana | 59 | 31458 | 187.6 | 80.3% |
St Tammany | Louisiana | 37 | 23624 | 156.6 | 67.1% |
Tangipahoa | Louisiana | 78 | 45519 | 171.4 | 73.4% |
Tensas | Louisiana | 14 | 15940 | 87.8 | 37.6% |
Terrebonne | Louisiana | 64 | 35880 | 178.4 | 76.4% |
Union | Louisiana | 30 | 20943 | 143.2 | 61.4% |
Vermilion | Louisiana | 82 | 37750 | 217.2 | 93.0% |
Vernon | Louisiana | 45 | 19142 | 235.1 | 100.7% |
Washington | Louisiana | 62 | 34443 | 180.0 | 77.1% |
Webster | Louisiana | 44 | 33676 | 130.7 | 56.0% |
West Baton Rouge | Louisiana | 9 | 11263 | 79.9 | 34.2% |
West Carroll | Louisiana | 50 | 19252 | 259.7 | 111.2% |
West Feliciana | Louisiana | 11 | 11720 | 93.9 | 40.2% |
Winn | Louisiana | 24 | 16923 | 141.8 | 60.7% |
Barnstable | Massachusetts | 58 | 37295 | 155.5 | 66.6% |
Berkshire | Massachusetts | 323 | 122273 | 264.2 | 113.2% |
Bristol | Massachusetts | 870 | 364637 | 238.6 | 102.2% |
Dukes | Massachusetts | 21 | 5669 | 370.4 | 158.7% |
Essex | Massachusetts | 1081 | 496313 | 217.8 | 93.3% |
Franklin | Massachusetts | 144 | 49453 | 291.2 | 124.7% |
Hampden | Massachusetts | 819 | 332107 | 246.6 | 105.6% |
Hampshire | Massachusetts | 200 | 72461 | 276.0 | 118.2% |
Middlesex | Massachusetts | 2246 | 971390 | 231.2 | 99.0% |
Nantucket | Massachusetts | 8 | 3401 | 235.2 | 100.8% |
Norfolk | Massachusetts | 688 | 325180 | 211.6 | 90.6% |
Plymouth | Massachusetts | 356 | 168824 | 210.9 | 90.3% |
Suffolk | Massachusetts | 1856 | 863248 | 215.0 | 92.1% |
Worcester | Massachusetts | 1282 | 504470 | 254.1 | 108.9% |
Allegany | Maryland | 282 | 86973 | 324.2 | 138.9% |
Anne Arundel | Maryland | 146 | 68375 | 213.5 | 91.5% |
Baltimore | Maryland | 367 | 155825 | 235.5 | 100.9% |
Baltimore (city) | Maryland | 2031 | 859100 | 236.4 | 101.3% |
Calvert | Maryland | 20 | 10484 | 190.8 | 81.7% |
Caroline | Maryland | 29 | 17549 | 165.3 | 70.8% |
Carroll | Maryland | 88 | 39054 | 225.3 | 96.5% |
Cecil | Maryland | 63 | 26407 | 238.6 | 102.2% |
Charles | Maryland | 38 | 17612 | 215.8 | 92.4% |
Dorchester | Maryland | 61 | 28006 | 217.8 | 93.3% |
Frederick | Maryland | 141 | 57312 | 246.0 | 105.4% |
Garrett | Maryland | 52 | 21981 | 236.6 | 101.3% |
Harford | Maryland | 109 | 35060 | 310.9 | 133.2% |
Howard | Maryland | 43 | 17175 | 250.4 | 107.2% |
Kent | Maryland | 29 | 13465 | 215.4 | 92.3% |
Montgomery | Maryland | 189 | 83912 | 225.2 | 96.5% |
Prince Georges | Maryland | 212 | 89490 | 236.9 | 101.5% |
Queen Annes | Maryland | 27 | 14476 | 186.5 | 79.9% |
Somerset | Maryland | 51 | 20965 | 243.3 | 104.2% |
St Marys | Maryland | 33 | 14626 | 225.6 | 96.6% |
Talbot | Maryland | 29 | 18784 | 154.4 | 66.1% |
Washington | Maryland | 174 | 68838 | 252.8 | 108.3% |
Wicomico | Maryland | 69 | 34530 | 199.8 | 85.6% |
Worcester | Maryland | 40 | 21245 | 188.3 | 80.6% |
Androscoggin | Maine | 203 | 76679 | 264.7 | 113.4% |
Aroostook | Maine | 223 | 94436 | 236.1 | 101.1% |
Cumberland | Maine | 389 | 146000 | 266.4 | 114.1% |
Franklin | Maine | 56 | 19896 | 281.5 | 120.6% |
Hancock | Maine | 67 | 32422 | 206.6 | 88.5% |
Kennebec | Maine | 209 | 77231 | 270.6 | 115.9% |
Knox | Maine | 63 | 27191 | 231.7 | 99.2% |
Lincoln | Maine | 34 | 16294 | 208.7 | 89.4% |
Oxford | Maine | 127 | 42662 | 297.7 | 127.5% |
Penobscot | Maine | 238 | 97104 | 245.1 | 105.0% |
Piscataquis | Maine | 57 | 18467 | 308.7 | 132.2% |
Sagadahoc | Maine | 38 | 19123 | 198.7 | 85.1% |
Somerset | Maine | 93 | 38245 | 243.2 | 104.2% |
Waldo | Maine | 53 | 21159 | 250.5 | 107.3% |
Washington | Maine | 76 | 37767 | 201.2 | 86.2% |
York | Maine | 206 | 82550 | 249.5 | 106.9% |
Alcona | Michigan | 16 | 5463 | 292.9 | 125.5% |
Alger | Michigan | 29 | 10167 | 285.2 | 122.2% |
Allegan | Michigan | 87 | 41839 | 207.9 | 89.1% |
Alpena | Michigan | 46 | 20766 | 221.5 | 94.9% |
Antrim | Michigan | 33 | 10964 | 301.0 | 128.9% |
Arenac | Michigan | 24 | 9233 | 259.9 | 111.3% |
Baraga | Michigan | 24 | 9356 | 256.5 | 109.9% |
Barry | Michigan | 46 | 22613 | 203.4 | 87.1% |
Bay | Michigan | 182 | 74981 | 242.7 | 104.0% |
Benzie | Michigan | 16 | 7800 | 205.1 | 87.9% |
Berrien | Michigan | 237 | 89117 | 265.9 | 113.9% |
Branch | Michigan | 51 | 25845 | 197.3 | 84.5% |
Calhoun | Michigan | 207 | 94206 | 219.7 | 94.1% |
Cass | Michigan | 36 | 21910 | 164.3 | 70.4% |
Charlevoix | Michigan | 33 | 13031 | 253.2 | 108.5% |
Cheboygan | Michigan | 43 | 13644 | 315.2 | 135.0% |
Chippewa | Michigan | 72 | 27807 | 258.9 | 110.9% |
Clare | Michigan | 26 | 9163 | 283.7 | 121.5% |
Clinton | Michigan | 46 | 26671 | 172.5 | 73.9% |
Crawford | Michigan | 11 | 3765 | 292.2 | 125.1% |
Delta | Michigan | 106 | 34037 | 311.4 | 133.4% |
Dickinson | Michigan | 88 | 28731 | 306.3 | 131.2% |
Eaton | Michigan | 64 | 34124 | 187.6 | 80.3% |
Emmet | Michigan | 33 | 15791 | 209.0 | 89.5% |
Genesee | Michigan | 498 | 227944 | 218.5 | 93.6% |
Gladwin | Michigan | 27 | 9385 | 287.7 | 123.2% |
Gogebic | Michigan | 127 | 31797 | 399.4 | 171.1% |
Grand Traverse | Michigan | 46 | 23390 | 196.7 | 84.2% |
Gratiot | Michigan | 51 | 32205 | 158.4 | 67.8% |
Hillsdale | Michigan | 55 | 29092 | 189.1 | 81.0% |
Houghton | Michigan | 130 | 47631 | 272.9 | 116.9% |
Huron | Michigan | 77 | 32584 | 236.3 | 101.2% |
Ingham | Michigan | 322 | 130616 | 246.5 | 105.6% |
Ionia | Michigan | 91 | 35710 | 254.8 | 109.2% |
Iosco | Michigan | 16 | 8560 | 186.9 | 80.1% |
Iron | Michigan | 82 | 20243 | 405.1 | 173.5% |
Isabella | Michigan | 52 | 25982 | 200.1 | 85.7% |
Jackson | Michigan | 186 | 93108 | 199.8 | 85.6% |
Kalamazoo | Michigan | 246 | 100085 | 245.8 | 105.3% |
Kalkaska | Michigan | 15 | 5159 | 290.8 | 124.5% |
Kent | Michigan | 583 | 246338 | 236.7 | 101.4% |
Keweenaw | Michigan | 11 | 4004 | 274.7 | 117.7% |
Lake | Michigan | 9 | 4798 | 187.6 | 80.3% |
Lapeer | Michigan | 65 | 32116 | 202.4 | 86.7% |
Leelanau | Michigan | 11 | 8436 | 130.4 | 55.9% |
Lenawee | Michigan | 133 | 53110 | 250.4 | 107.3% |
Livingston | Michigan | 46 | 20863 | 220.5 | 94.4% |
Luce | Michigan | 11 | 7423 | 148.2 | 63.5% |
Mackinac | Michigan | 20 | 9438 | 211.9 | 90.8% |
Macomb | Michigan | 252 | 107638 | 234.1 | 100.3% |
Manistee | Michigan | 48 | 18450 | 260.2 | 111.4% |
Marquette | Michigan | 107 | 47144 | 227.0 | 97.2% |
Mason | Michigan | 56 | 19378 | 289.0 | 123.8% |
Mecosta | Michigan | 44 | 16902 | 260.3 | 111.5% |
Menominee | Michigan | 61 | 24883 | 245.1 | 105.0% |
Midland | Michigan | 64 | 27094 | 236.2 | 101.2% |
Missaukee | Michigan | 19 | 8034 | 236.5 | 101.3% |
Monroe | Michigan | 131 | 58620 | 223.5 | 95.7% |
Montcalm | Michigan | 56 | 28581 | 195.9 | 83.9% |
Montmorency | Michigan | 10 | 3840 | 260.4 | 111.5% |
Muskegon | Michigan | 274 | 94501 | 289.9 | 124.2% |
Newaygo | Michigan | 44 | 19286 | 228.1 | 97.7% |
Oakland | Michigan | 600 | 254068 | 236.2 | 101.2% |
Oceana | Michigan | 37 | 14812 | 249.8 | 107.0% |
Ogemaw | Michigan | 15 | 8720 | 172.0 | 73.7% |
Ontonagon | Michigan | 39 | 11359 | 343.3 | 147.1% |
Osceola | Michigan | 32 | 13309 | 240.4 | 103.0% |
Oscoda | Michigan | 6 | 2543 | 235.9 | 101.1% |
Otsego | Michigan | 11 | 5827 | 188.8 | 80.9% |
Ottawa | Michigan | 166 | 59660 | 278.2 | 119.2% |
Presque Isle | Michigan | 32 | 12250 | 261.2 | 111.9% |
Roscommon | Michigan | 13 | 3668 | 354.4 | 151.8% |
Saginaw | Michigan | 317 | 130468 | 243.0 | 104.1% |
Sanilac | Michigan | 39 | 30114 | 129.5 | 55.5% |
Schoolcraft | Michigan | 34 | 9524 | 357.0 | 152.9% |
Shiawassee | Michigan | 81 | 41207 | 196.6 | 84.2% |
St Clair | Michigan | 195 | 76222 | 255.8 | 109.6% |
St Joseph | Michigan | 60 | 31749 | 189.0 | 81.0% |
Tuscola | Michigan | 62 | 35694 | 173.7 | 74.4% |
Van Buren | Michigan | 85 | 35111 | 242.1 | 103.7% |
Washtenaw | Michigan | 201 | 80810 | 248.7 | 106.5% |
Wayne | Michigan | 5104 | 2015623 | 253.2 | 108.5% |
Wexford | Michigan | 48 | 17976 | 267.0 | 114.4% |
Aitkin | Minnesota | 50 | 17865 | 279.9 | 119.9% |
Anoka | Minnesota | 32 | 22443 | 142.6 | 61.1% |
Becker | Minnesota | 61 | 26562 | 229.7 | 98.4% |
Beltrami | Minnesota | 81 | 26107 | 310.3 | 132.9% |
Benton | Minnesota | 47 | 16106 | 291.8 | 125.0% |
Big St.one | Minnesota | 38 | 10447 | 363.7 | 155.8% |
Blue Earth | Minnesota | 73 | 36203 | 201.6 | 86.4% |
Brown | Minnesota | 42 | 25544 | 164.4 | 70.4% |
Carlton | Minnesota | 66 | 24212 | 272.6 | 116.8% |
Carver | Minnesota | 23 | 17606 | 130.6 | 56.0% |
Cass | Minnesota | 43 | 20646 | 208.3 | 89.2% |
Chippewa | Minnesota | 46 | 16927 | 271.8 | 116.4% |
Chisago | Minnesota | 25 | 13124 | 190.5 | 81.6% |
Clay | Minnesota | 59 | 25337 | 232.9 | 99.7% |
Clearwater | Minnesota | 40 | 11153 | 358.6 | 153.6% |
Cook | Minnesota | 8 | 3030 | 264.0 | 113.1% |
Cottonwood | Minnesota | 31 | 16143 | 192.0 | 82.3% |
Crow Wing | Minnesota | 84 | 30226 | 277.9 | 119.0% |
Dakota | Minnesota | 86 | 39660 | 216.8 | 92.9% |
Dodge | Minnesota | 19 | 12931 | 146.9 | 62.9% |
Douglas | Minnesota | 29 | 20369 | 142.4 | 61.0% |
Faribault | Minnesota | 54 | 23941 | 225.6 | 96.6% |
Fillmore | Minnesota | 54 | 25830 | 209.1 | 89.6% |
Freeborn | Minnesota | 84 | 31780 | 264.3 | 113.2% |
Goodhue | Minnesota | 72 | 31564 | 228.1 | 97.7% |
Grant | Minnesota | 18 | 9828 | 183.2 | 78.5% |
Hennepin | Minnesota | 1340 | 568899 | 235.5 | 100.9% |
Houston | Minnesota | 27 | 14735 | 183.2 | 78.5% |
Hubbard | Minnesota | 26 | 11085 | 234.6 | 100.5% |
Isanti | Minnesota | 20 | 12950 | 154.4 | 66.2% |
Itasca | Minnesota | 90 | 32996 | 272.8 | 116.8% |
Jackson | Minnesota | 38 | 16805 | 226.1 | 96.9% |
Kanabec | Minnesota | 17 | 9651 | 176.1 | 75.5% |
Kandiyohi | Minnesota | 54 | 26524 | 203.6 | 87.2% |
Kittson | Minnesota | 26 | 10717 | 242.6 | 103.9% |
Koochiching | Minnesota | 58 | 16930 | 342.6 | 146.7% |
Lac Qui Parle | Minnesota | 45 | 15509 | 290.2 | 124.3% |
Lake | Minnesota | 18 | 6956 | 258.8 | 110.8% |
Lake Of The Woods | Minnesota | 16 | 5975 | 267.8 | 114.7% |
Le Sueur | Minnesota | 27 | 19227 | 140.4 | 60.2% |
Lincoln | Minnesota | 28 | 10797 | 259.3 | 111.1% |
Lyon | Minnesota | 62 | 21569 | 287.4 | 123.1% |
Mahnomen | Minnesota | 24 | 8054 | 298.0 | 127.6% |
Marshall | Minnesota | 31 | 18364 | 168.8 | 72.3% |
Martin | Minnesota | 51 | 24656 | 206.8 | 88.6% |
Mcleod | Minnesota | 45 | 21380 | 210.5 | 90.2% |
Meeker | Minnesota | 40 | 19277 | 207.5 | 88.9% |
Mille Lacs | Minnesota | 38 | 15558 | 244.2 | 104.6% |
Morrison | Minnesota | 68 | 27473 | 247.5 | 106.0% |
Mower | Minnesota | 88 | 36113 | 243.7 | 104.4% |
Murray | Minnesota | 27 | 15060 | 179.3 | 76.8% |
Nicollet | Minnesota | 29 | 18282 | 158.6 | 67.9% |
Nobles | Minnesota | 40 | 21215 | 188.5 | 80.8% |
Norman | Minnesota | 38 | 14746 | 257.7 | 110.4% |
Olmsted | Minnesota | 81 | 42658 | 189.9 | 81.3% |
Otter Tail | Minnesota | 87 | 53192 | 163.6 | 70.1% |
Pennington | Minnesota | 35 | 12913 | 271.0 | 116.1% |
Pine | Minnesota | 48 | 21478 | 223.5 | 95.7% |
Pipestone | Minnesota | 38 | 13794 | 275.5 | 118.0% |
Polk | Minnesota | 83 | 37734 | 220.0 | 94.2% |
Pope | Minnesota | 39 | 13544 | 288.0 | 123.3% |
Ramsey | Minnesota | 746 | 309935 | 240.7 | 103.1% |
Red Lake | Minnesota | 17 | 7413 | 229.3 | 98.2% |
Redwood | Minnesota | 59 | 22290 | 264.7 | 113.4% |
Renville | Minnesota | 58 | 24625 | 235.5 | 100.9% |
Rice | Minnesota | 60 | 32160 | 186.6 | 79.9% |
Rock | Minnesota | 17 | 10933 | 155.5 | 66.6% |
Roseau | Minnesota | 27 | 15103 | 178.8 | 76.6% |
Scott | Minnesota | 21 | 15585 | 134.7 | 57.7% |
Sherburne | Minnesota | 30 | 10456 | 286.9 | 122.9% |
Sibley | Minnesota | 30 | 16625 | 180.5 | 77.3% |
St.earns | Minnesota | 120 | 67200 | 178.6 | 76.5% |
St.eele | Minnesota | 43 | 19749 | 217.7 | 93.3% |
St.evens | Minnesota | 22 | 11039 | 199.3 | 85.4% |
St Louis | Minnesota | 573 | 206917 | 276.9 | 118.6% |
Swift | Minnesota | 48 | 15469 | 310.3 | 132.9% |
Todd | Minnesota | 54 | 27438 | 196.8 | 84.3% |
Traverse | Minnesota | 19 | 8283 | 229.4 | 98.3% |
Wabasha | Minnesota | 42 | 17653 | 237.9 | 101.9% |
Wadena | Minnesota | 41 | 12772 | 321.0 | 137.5% |
Waseca | Minnesota | 31 | 15186 | 204.1 | 87.4% |
Washington | Minnesota | 58 | 26430 | 219.4 | 94.0% |
Watonwan | Minnesota | 34 | 13902 | 244.6 | 104.8% |
Wilkin | Minnesota | 20 | 10475 | 190.9 | 81.8% |
Winona | Minnesota | 64 | 37795 | 169.3 | 72.5% |
Wright | Minnesota | 42 | 27550 | 152.5 | 65.3% |
Yellow Medicine | Minnesota | 34 | 16917 | 201.0 | 86.1% |
Adair | Missouri | 44 | 20246 | 217.3 | 93.1% |
Andrew | Missouri | 29 | 13015 | 222.8 | 95.4% |
Atchison | Missouri | 41 | 12897 | 317.9 | 136.2% |
Audrain | Missouri | 57 | 22673 | 251.4 | 107.7% |
Barry | Missouri | 45 | 23546 | 191.1 | 81.9% |
Barton | Missouri | 36 | 14148 | 254.5 | 109.0% |
Bates | Missouri | 38 | 19531 | 194.6 | 83.3% |
Benton | Missouri | 25 | 11142 | 224.4 | 96.1% |
Bollinger | Missouri | 17 | 12898 | 131.8 | 56.5% |
Boone | Missouri | 84 | 34991 | 240.1 | 102.8% |
Buchanan | Missouri | 216 | 94067 | 229.6 | 98.4% |
Butler | Missouri | 61 | 34276 | 178.0 | 76.2% |
Caldwell | Missouri | 38 | 11629 | 326.8 | 140.0% |
Callaway | Missouri | 43 | 23094 | 186.2 | 79.8% |
Camden | Missouri | 24 | 8971 | 267.5 | 114.6% |
Cape Girardeau | Missouri | 80 | 37775 | 211.8 | 90.7% |
Carroll | Missouri | 31 | 17814 | 174.0 | 74.5% |
Carter | Missouri | 11 | 6226 | 176.7 | 75.7% |
Cass | Missouri | 44 | 19534 | 225.2 | 96.5% |
Cedar | Missouri | 33 | 11697 | 282.1 | 120.8% |
Chariton | Missouri | 40 | 18084 | 221.2 | 94.7% |
Christian | Missouri | 23 | 13538 | 169.9 | 72.8% |
Clark | Missouri | 12 | 10166 | 118.0 | 50.6% |
Clay | Missouri | 61 | 30417 | 200.5 | 85.9% |
Clinton | Missouri | 37 | 13261 | 279.0 | 119.5% |
Cole | Missouri | 83 | 34912 | 237.7 | 101.8% |
Cooper | Missouri | 47 | 18075 | 260.0 | 111.4% |
Crawford | Missouri | 41 | 12693 | 323.0 | 138.4% |
Dade | Missouri | 26 | 11248 | 231.2 | 99.0% |
Dallas | Missouri | 21 | 11523 | 182.2 | 78.1% |
Daviess | Missouri | 35 | 13398 | 261.2 | 111.9% |
De Kalb | Missouri | 19 | 9751 | 194.9 | 83.5% |
Dent | Missouri | 35 | 11763 | 297.5 | 127.5% |
Douglas | Missouri | 29 | 15600 | 185.9 | 79.6% |
Dunklin | Missouri | 95 | 44957 | 211.3 | 90.5% |
Franklin | Missouri | 67 | 33868 | 197.8 | 84.7% |
Gasconade | Missouri | 24 | 12414 | 193.3 | 82.8% |
Gentry | Missouri | 35 | 13359 | 262.0 | 112.2% |
Greene | Missouri | 186 | 90541 | 205.4 | 88.0% |
Grundy | Missouri | 34 | 15716 | 216.3 | 92.7% |
Harrison | Missouri | 30 | 16525 | 181.5 | 77.8% |
Henry | Missouri | 53 | 22313 | 237.5 | 101.7% |
Hickory | Missouri | 8 | 6506 | 123.0 | 52.7% |
Holt | Missouri | 30 | 12476 | 240.5 | 103.0% |
Howard | Missouri | 27 | 13026 | 207.3 | 88.8% |
Howell | Missouri | 73 | 22270 | 327.8 | 140.4% |
Iron | Missouri | 25 | 10440 | 239.5 | 102.6% |
Jackson | Missouri | 1045 | 477828 | 218.7 | 93.7% |
Jasper | Missouri | 194 | 78705 | 246.5 | 105.6% |
Jefferson | Missouri | 74 | 32023 | 231.1 | 99.0% |
Johnson | Missouri | 46 | 21617 | 212.8 | 91.2% |
Knox | Missouri | 16 | 8878 | 180.2 | 77.2% |
Laclede | Missouri | 43 | 18718 | 229.7 | 98.4% |
Lafayette | Missouri | 82 | 27856 | 294.4 | 126.1% |
Lawrence | Missouri | 38 | 24637 | 154.2 | 66.1% |
Lewis | Missouri | 25 | 11490 | 217.6 | 93.2% |
Lincoln | Missouri | 35 | 14395 | 243.1 | 104.1% |
Linn | Missouri | 47 | 21416 | 219.5 | 94.0% |
Livingston | Missouri | 29 | 18000 | 161.1 | 69.0% |
Macon | Missouri | 42 | 21396 | 196.3 | 84.1% |
Madison | Missouri | 24 | 9656 | 248.6 | 106.5% |
Maries | Missouri | 15 | 8638 | 173.7 | 74.4% |
Marion | Missouri | 72 | 31576 | 228.0 | 97.7% |
Mcdonald | Missouri | 40 | 15749 | 254.0 | 108.8% |
Mercer | Missouri | 24 | 8766 | 273.8 | 117.3% |
Miller | Missouri | 23 | 14798 | 155.4 | 66.6% |
Mississippi | Missouri | 43 | 23149 | 185.8 | 79.6% |
Moniteau | Missouri | 27 | 11775 | 229.3 | 98.2% |
Monroe | Missouri | 19 | 13195 | 144.0 | 61.7% |
Montgomery | Missouri | 27 | 12442 | 217.0 | 93.0% |
Morgan | Missouri | 30 | 11140 | 269.3 | 115.4% |
New Madrid | Missouri | 81 | 39787 | 203.6 | 87.2% |
Newton | Missouri | 58 | 29039 | 199.7 | 85.6% |
Nodaway | Missouri | 62 | 25556 | 242.6 | 103.9% |
Oregon | Missouri | 28 | 13390 | 209.1 | 89.6% |
Osage | Missouri | 28 | 12375 | 226.3 | 96.9% |
Ozark | Missouri | 25 | 10766 | 232.2 | 99.5% |
Pemiscot | Missouri | 87 | 46857 | 185.7 | 79.5% |
Perry | Missouri | 26 | 15358 | 169.3 | 72.5% |
Pettis | Missouri | 61 | 33336 | 183.0 | 78.4% |
Phelps | Missouri | 44 | 17437 | 252.3 | 108.1% |
Pike | Missouri | 31 | 18327 | 169.1 | 72.5% |
Platte | Missouri | 34 | 13862 | 245.3 | 105.1% |
Polk | Missouri | 42 | 17400 | 241.4 | 103.4% |
Pulaski | Missouri | 22 | 10775 | 204.2 | 87.5% |
Putnam | Missouri | 17 | 11327 | 150.1 | 64.3% |
Ralls | Missouri | 15 | 10040 | 149.4 | 64.0% |
Randolph | Missouri | 48 | 24458 | 196.3 | 84.1% |
Ray | Missouri | 51 | 18584 | 274.4 | 117.6% |
Reynolds | Missouri | 21 | 9370 | 224.1 | 96.0% |
Ripley | Missouri | 26 | 12606 | 206.3 | 88.3% |
Saline | Missouri | 74 | 29416 | 251.6 | 107.8% |
Schuyler | Missouri | 20 | 6627 | 301.8 | 129.3% |
Scotland | Missouri | 19 | 8557 | 222.0 | 95.1% |
Scott | Missouri | 74 | 30377 | 243.6 | 104.3% |
Shannon | Missouri | 20 | 11831 | 169.0 | 72.4% |
Shelby | Missouri | 14 | 11224 | 124.7 | 53.4% |
St Charles | Missouri | 43 | 25562 | 168.2 | 72.1% |
St Clair | Missouri | 25 | 13146 | 190.2 | 81.5% |
St.e. Genevieve | Missouri | 27 | 10905 | 247.6 | 106.1% |
St Francois | Missouri | 71 | 35950 | 197.5 | 84.6% |
St Louis | Missouri | 453 | 274230 | 165.2 | 70.8% |
St Louis (city) | Missouri | 1636 | 816048 | 200.5 | 85.9% |
St.oddard | Missouri | 53 | 33009 | 160.6 | 68.8% |
St.one | Missouri | 26 | 11298 | 230.1 | 98.6% |
Sullivan | Missouri | 34 | 13701 | 248.2 | 106.3% |
Taney | Missouri | 20 | 10323 | 193.7 | 83.0% |
Texas | Missouri | 48 | 19813 | 242.3 | 103.8% |
Vernon | Missouri | 66 | 25586 | 258.0 | 110.5% |
Warren | Missouri | 16 | 7734 | 206.9 | 88.6% |
Washington | Missouri | 39 | 17492 | 223.0 | 95.5% |
Wayne | Missouri | 28 | 12794 | 218.9 | 93.7% |
Webster | Missouri | 31 | 17226 | 180.0 | 77.1% |
Worth | Missouri | 15 | 6345 | 236.4 | 101.3% |
Wright | Missouri | 39 | 17967 | 217.1 | 93.0% |
Adams | Mississippi | 36 | 27238 | 132.2 | 56.6% |
Alcorn | Mississippi | 59 | 26969 | 218.8 | 93.7% |
Amite | Mississippi | 31 | 21892 | 141.6 | 60.7% |
Attala | Mississippi | 51 | 30227 | 168.7 | 72.3% |
Benton | Mississippi | 24 | 10429 | 230.1 | 98.6% |
Bolivar | Mississippi | 67 | 67574 | 99.2 | 42.5% |
Calhoun | Mississippi | 52 | 20893 | 248.9 | 106.6% |
Carroll | Mississippi | 29 | 20651 | 140.4 | 60.2% |
Chickasaw | Mississippi | 37 | 21427 | 172.7 | 74.0% |
Choctaw | Mississippi | 31 | 13548 | 228.8 | 98.0% |
Claiborne | Mississippi | 18 | 12810 | 140.5 | 60.2% |
Clarke | Mississippi | 32 | 20596 | 155.4 | 66.6% |
Clay | Mississippi | 30 | 19030 | 157.6 | 67.5% |
Coahoma | Mississippi | 50 | 48333 | 103.4 | 44.3% |
Copiah | Mississippi | 58 | 33974 | 170.7 | 73.1% |
Covington | Mississippi | 32 | 17030 | 187.9 | 80.5% |
De Soto | Mississippi | 26 | 26663 | 97.5 | 41.8% |
Forrest | Mississippi | 88 | 34901 | 252.1 | 108.0% |
Franklin | Mississippi | 30 | 12504 | 239.9 | 102.8% |
George | Mississippi | 22 | 8704 | 252.8 | 108.3% |
Greene | Mississippi | 23 | 9512 | 241.8 | 103.6% |
Grenada | Mississippi | 42 | 19052 | 220.4 | 94.4% |
Hancock | Mississippi | 23 | 11328 | 203.0 | 87.0% |
Harrison | Mississippi | 119 | 50799 | 234.3 | 100.3% |
Hinds | Mississippi | 222 | 107273 | 206.9 | 88.6% |
Holmes | Mississippi | 44 | 39710 | 110.8 | 47.5% |
Humphreys | Mississippi | 42 | 26257 | 160.0 | 68.5% |
Issaquena | Mississippi | 7 | 6433 | 108.8 | 46.6% |
Itawamba | Mississippi | 45 | 19922 | 225.9 | 96.8% |
Jackson | Mississippi | 61 | 20601 | 296.1 | 126.8% |
Jasper | Mississippi | 35 | 19484 | 179.6 | 76.9% |
Jefferson | Mississippi | 16 | 13969 | 114.5 | 49.1% |
Jefferson Davis | Mississippi | 9 | 15869 | 56.7 | 24.3% |
Jones | Mississippi | 84 | 49227 | 170.6 | 73.1% |
Kemper | Mississippi | 33 | 21867 | 150.9 | 64.6% |
Lafayette | Mississippi | 46 | 21257 | 216.4 | 92.7% |
Lamar | Mississippi | 40 | 12096 | 330.7 | 141.6% |
Lauderdale | Mississippi | 111 | 58247 | 190.6 | 81.6% |
Lawrence | Mississippi | 26 | 13983 | 185.9 | 79.6% |
Leake | Mississippi | 42 | 24570 | 170.9 | 73.2% |
Lee | Mississippi | 101 | 38838 | 260.1 | 111.4% |
Leflore | Mississippi | 59 | 53406 | 110.5 | 47.3% |
Lincoln | Mississippi | 43 | 27506 | 156.3 | 67.0% |
Lowndes | Mississippi | 55 | 35245 | 156.1 | 66.8% |
Madison | Mississippi | 37 | 37504 | 98.7 | 42.3% |
Marion | Mississippi | 44 | 24085 | 182.7 | 78.3% |
Marshall | Mississippi | 18 | 25522 | 70.5 | 30.2% |
Monroe | Mississippi | 62 | 37648 | 164.7 | 70.5% |
Montgomery | Mississippi | 37 | 15703 | 235.6 | 100.9% |
Neshoba | Mississippi | 33 | 27882 | 118.4 | 50.7% |
Newton | Mississippi | 45 | 24249 | 185.6 | 79.5% |
Noxubee | Mississippi | 25 | 25669 | 97.4 | 41.7% |
Oktibbeha | Mississippi | 38 | 22151 | 171.5 | 73.5% |
Panola | Mississippi | 37 | 34421 | 107.5 | 46.0% |
Pearl River | Mississippi | 48 | 19125 | 251.0 | 107.5% |
Perry | Mississippi | 9 | 9292 | 96.9 | 41.5% |
Pike | Mississippi | 70 | 35002 | 200.0 | 85.7% |
Pontotoc | Mississippi | 43 | 22904 | 187.7 | 80.4% |
Prentiss | Mississippi | 38 | 20921 | 181.6 | 77.8% |
Quitman | Mississippi | 35 | 27191 | 128.7 | 55.1% |
Rankin | Mississippi | 21 | 27934 | 75.2 | 32.2% |
Scott | Mississippi | 37 | 23144 | 159.9 | 68.5% |
Sharkey | Mississippi | 10 | 15433 | 64.8 | 27.8% |
Simpson | Mississippi | 43 | 22024 | 195.2 | 83.6% |
Smith | Mississippi | 40 | 19403 | 206.2 | 88.3% |
St.one | Mississippi | 14 | 6155 | 227.5 | 97.4% |
Sunflower | Mississippi | 57 | 61007 | 93.4 | 40.0% |
Tallahatchie | Mississippi | 30 | 34166 | 87.8 | 37.6% |
Tate | Mississippi | 19 | 19309 | 98.4 | 42.1% |
Tippah | Mississippi | 52 | 19680 | 264.2 | 113.2% |
Tishomingo | Mississippi | 43 | 16974 | 253.3 | 108.5% |
Tunica | Mississippi | 15 | 22610 | 66.3 | 28.4% |
Union | Mississippi | 53 | 21867 | 242.4 | 103.8% |
Walthall | Mississippi | 22 | 17534 | 125.5 | 53.7% |
Warren | Mississippi | 52 | 39595 | 131.3 | 56.3% |
Washington | Mississippi | 71 | 67576 | 105.1 | 45.0% |
Wayne | Mississippi | 30 | 16928 | 177.2 | 75.9% |
Webster | Mississippi | 26 | 14160 | 183.6 | 78.7% |
Wilkinson | Mississippi | 23 | 15955 | 144.2 | 61.7% |
Winston | Mississippi | 40 | 22751 | 175.8 | 75.3% |
Yalobusha | Mississippi | 37 | 18387 | 201.2 | 86.2% |
Yazoo | Mississippi | 46 | 40091 | 114.7 | 49.1% |
Beaverhead | Montana | 18 | 6943 | 259.3 | 111.1% |
Big Horn | Montana | 22 | 10419 | 211.2 | 90.4% |
Blaine | Montana | 31 | 9566 | 324.1 | 138.8% |
Broadwater | Montana | 17 | 3451 | 492.6 | 211.0% |
Carbon | Montana | 44 | 11865 | 370.8 | 158.8% |
Carter | Montana | 21 | 3280 | 640.2 | 274.2% |
Cascade | Montana | 114 | 41999 | 271.4 | 116.3% |
Chouteau | Montana | 25 | 7316 | 341.7 | 146.4% |
Custer | Montana | 30 | 10422 | 287.9 | 123.3% |
Daniels | Montana | 10 | 4563 | 219.2 | 93.9% |
Dawson | Montana | 36 | 8618 | 417.7 | 178.9% |
Deer Lodge | Montana | 41 | 13627 | 300.9 | 128.9% |
Fallon | Montana | 14 | 3719 | 376.4 | 161.2% |
Fergus | Montana | 42 | 14040 | 299.1 | 128.1% |
Flathead | Montana | 81 | 24271 | 333.7 | 143.0% |
Gallatin | Montana | 58 | 18269 | 317.5 | 136.0% |
Garfield | Montana | 13 | 2641 | 492.2 | 210.8% |
Glacier | Montana | 27 | 9034 | 298.9 | 128.0% |
Golden Valley | Montana | 8 | 1607 | 497.8 | 213.2% |
Granite | Montana | 11 | 3401 | 323.4 | 138.5% |
Hill | Montana | 41 | 13304 | 308.2 | 132.0% |
Jefferson | Montana | 10 | 4664 | 214.4 | 91.8% |
Judith Basin | Montana | 7 | 3655 | 191.5 | 82.0% |
Lake | Montana | 35 | 13490 | 259.5 | 111.1% |
Lewis And Clark | Montana | 56 | 22131 | 253.0 | 108.4% |
Liberty | Montana | 3 | 2209 | 135.8 | 58.2% |
Lincoln | Montana | 16 | 7882 | 203.0 | 87.0% |
Madison | Montana | 11 | 7294 | 150.8 | 64.6% |
Mccone | Montana | 10 | 3798 | 263.3 | 112.8% |
Meagher | Montana | 5 | 2237 | 223.5 | 95.7% |
Mineral | Montana | 6 | 2135 | 281.0 | 120.4% |
Missoula | Montana | 77 | 29038 | 265.2 | 113.6% |
Musselshell | Montana | 15 | 5717 | 262.4 | 112.4% |
Park | Montana | 33 | 11566 | 285.3 | 122.2% |
Petroleum | Montana | 3 | 1083 | 277.0 | 118.7% |
Phillips | Montana | 30 | 7892 | 380.1 | 162.8% |
Pondera | Montana | 17 | 6716 | 253.1 | 108.4% |
Powder River | Montana | 4 | 3159 | 126.6 | 54.2% |
Powell | Montana | 13 | 6152 | 211.3 | 90.5% |
Prairie | Montana | 10 | 2410 | 414.9 | 177.7% |
Ravalli | Montana | 37 | 12978 | 285.1 | 122.1% |
Richland | Montana | 25 | 10209 | 244.9 | 104.9% |
Roosevelt | Montana | 37 | 9806 | 377.3 | 161.6% |
Rosebud | Montana | 16 | 6477 | 247.0 | 105.8% |
Sanders | Montana | 13 | 6926 | 187.7 | 80.4% |
Sheridan | Montana | 17 | 7814 | 217.6 | 93.2% |
Silver Bow | Montana | 120 | 53207 | 225.5 | 96.6% |
St.illwater | Montana | 10 | 5694 | 175.6 | 75.2% |
Sweet Grass | Montana | 8 | 3719 | 215.1 | 92.1% |
Teton | Montana | 18 | 6922 | 260.0 | 111.4% |
Toole | Montana | 19 | 6769 | 280.7 | 120.2% |
Treasure | Montana | 4 | 1499 | 266.8 | 114.3% |
Valley | Montana | 30 | 15181 | 197.6 | 84.6% |
Wheatland | Montana | 15 | 3286 | 456.5 | 195.5% |
Wibaux | Montana | 8 | 2161 | 370.2 | 158.6% |
Yellowstone | Montana | 99 | 41182 | 240.4 | 103.0% |
Yellowstone Np (pt) | Montana | 1 | 43 | 2325.6 | 996.2% |
Alamance | North Carolina | 153 | 57427 | 266.4 | 114.1% |
Alexander | North Carolina | 48 | 13454 | 356.8 | 152.8% |
Alleghany | North Carolina | 21 | 8341 | 251.8 | 107.8% |
Anson | North Carolina | 39 | 28443 | 137.1 | 58.7% |
Ashe | North Carolina | 53 | 22664 | 233.9 | 100.2% |
Avery | North Carolina | 42 | 13561 | 309.7 | 132.7% |
Beaufort | North Carolina | 56 | 36431 | 153.7 | 65.8% |
Bertie | North Carolina | 34 | 26201 | 129.8 | 55.6% |
Bladen | North Carolina | 39 | 27156 | 143.6 | 61.5% |
Brunswick | North Carolina | 25 | 17125 | 146.0 | 62.5% |
Buncombe | North Carolina | 255 | 108755 | 234.5 | 100.4% |
Burke | North Carolina | 109 | 38615 | 282.3 | 120.9% |
Cabarrus | North Carolina | 171 | 59393 | 287.9 | 123.3% |
Caldwell | North Carolina | 87 | 35795 | 243.1 | 104.1% |
Camden | North Carolina | 5 | 5440 | 91.9 | 39.4% |
Carteret | North Carolina | 26 | 18284 | 142.2 | 60.9% |
Caswell | North Carolina | 23 | 20032 | 114.8 | 49.2% |
Catawba | North Carolina | 114 | 51653 | 220.7 | 94.5% |
Chatham | North Carolina | 36 | 24726 | 145.6 | 62.4% |
Cherokee | North Carolina | 47 | 18813 | 249.8 | 107.0% |
Chowan | North Carolina | 16 | 11572 | 138.3 | 59.2% |
Clay | North Carolina | 12 | 6405 | 187.4 | 80.3% |
Cleveland | North Carolina | 122 | 58055 | 210.1 | 90.0% |
Columbus | North Carolina | 88 | 45663 | 192.7 | 82.5% |
Craven | North Carolina | 34 | 31298 | 108.6 | 46.5% |
Cumberland | North Carolina | 128 | 59320 | 215.8 | 92.4% |
Currituck | North Carolina | 7 | 6709 | 104.3 | 44.7% |
Dare | North Carolina | 5 | 6041 | 82.8 | 35.5% |
Davidson | North Carolina | 121 | 53377 | 226.7 | 97.1% |
Davie | North Carolina | 37 | 14909 | 248.2 | 106.3% |
Duplin | North Carolina | 66 | 39739 | 166.1 | 71.1% |
Durham | North Carolina | 157 | 80244 | 195.7 | 83.8% |
Edgecombe | North Carolina | 51 | 49162 | 103.7 | 44.4% |
Forsyth | North Carolina | 265 | 126475 | 209.5 | 89.8% |
Franklin | North Carolina | 26 | 30382 | 85.6 | 36.7% |
Gaston | North Carolina | 254 | 87531 | 290.2 | 124.3% |
Gates | North Carolina | 12 | 10060 | 119.3 | 51.1% |
Graham | North Carolina | 17 | 6418 | 264.9 | 113.5% |
Granville | North Carolina | 34 | 29344 | 115.9 | 49.6% |
Greene | North Carolina | 20 | 18548 | 107.8 | 46.2% |
Guilford | North Carolina | 355 | 153916 | 230.6 | 98.8% |
Halifax | North Carolina | 79 | 56512 | 139.8 | 59.9% |
Harnett | North Carolina | 65 | 44239 | 146.9 | 62.9% |
Haywood | North Carolina | 100 | 34804 | 287.3 | 123.1% |
Henderson | North Carolina | 60 | 26049 | 230.3 | 98.7% |
Hertford | North Carolina | 26 | 19352 | 134.4 | 57.5% |
Hoke | North Carolina | 14 | 14937 | 93.7 | 40.1% |
Hyde | North Carolina | 14 | 7860 | 178.1 | 76.3% |
Iredell | North Carolina | 97 | 50424 | 192.4 | 82.4% |
Jackson | North Carolina | 55 | 19366 | 284.0 | 121.7% |
Johnston | North Carolina | 98 | 63798 | 153.6 | 65.8% |
Jones | North Carolina | 9 | 10926 | 82.4 | 35.3% |
Lee | North Carolina | 53 | 18743 | 282.8 | 121.1% |
Lenoir | North Carolina | 68 | 41211 | 165.0 | 70.7% |
Lincoln | North Carolina | 71 | 24187 | 293.5 | 125.7% |
Macon | North Carolina | 44 | 15880 | 277.1 | 118.7% |
Madison | North Carolina | 43 | 22522 | 190.9 | 81.8% |
Martin | North Carolina | 31 | 26111 | 118.7 | 50.9% |
Mcdowell | North Carolina | 62 | 22996 | 269.6 | 115.5% |
Mecklenburg | North Carolina | 363 | 151826 | 239.1 | 102.4% |
Mitchell | North Carolina | 35 | 15980 | 219.0 | 93.8% |
Montgomery | North Carolina | 40 | 16280 | 245.7 | 105.2% |
Moore | North Carolina | 71 | 30969 | 229.3 | 98.2% |
Nash | North Carolina | 92 | 55608 | 165.4 | 70.9% |
New Hanover | North Carolina | 94 | 47935 | 196.1 | 84.0% |
Northampton | North Carolina | 22 | 28299 | 77.7 | 33.3% |
Onslow | North Carolina | 41 | 17939 | 228.6 | 97.9% |
Orange | North Carolina | 42 | 23072 | 182.0 | 78.0% |
Pamlico | North Carolina | 12 | 9706 | 123.6 | 53.0% |
Pasquotank | North Carolina | 26 | 20568 | 126.4 | 54.1% |
Pender | North Carolina | 22 | 17710 | 124.2 | 53.2% |
Perquimans | North Carolina | 13 | 9773 | 133.0 | 57.0% |
Person | North Carolina | 53 | 25029 | 211.8 | 90.7% |
Pitt | North Carolina | 80 | 61244 | 130.6 | 56.0% |
Polk | North Carolina | 34 | 11874 | 286.3 | 122.7% |
Randolph | North Carolina | 86 | 44554 | 193.0 | 82.7% |
Richmond | North Carolina | 63 | 36810 | 171.1 | 73.3% |
Robeson | North Carolina | 141 | 76860 | 183.5 | 78.6% |
Rockingham | North Carolina | 131 | 57898 | 226.3 | 96.9% |
Rowan | North Carolina | 146 | 69206 | 211.0 | 90.4% |
Rutherford | North Carolina | 88 | 45577 | 193.1 | 82.7% |
Sampson | North Carolina | 56 | 47440 | 118.0 | 50.6% |
Scotland | North Carolina | 38 | 23232 | 163.6 | 70.1% |
St.anly | North Carolina | 76 | 32834 | 231.5 | 99.1% |
St.okes | North Carolina | 39 | 22656 | 172.1 | 73.7% |
Surry | North Carolina | 113 | 41783 | 270.4 | 115.8% |
Swain | North Carolina | 43 | 12177 | 353.1 | 151.3% |
Transylvania | North Carolina | 35 | 12241 | 285.9 | 122.5% |
Tyrrell | North Carolina | 8 | 5556 | 144.0 | 61.7% |
Union | North Carolina | 89 | 39097 | 227.6 | 97.5% |
Vance | North Carolina | 67 | 29961 | 223.6 | 95.8% |
Wake | North Carolina | 198 | 109544 | 180.7 | 77.4% |
Warren | North Carolina | 35 | 23145 | 151.2 | 64.8% |
Washington | North Carolina | 20 | 12323 | 162.3 | 69.5% |
Watauga | North Carolina | 35 | 18114 | 193.2 | 82.8% |
Wayne | North Carolina | 82 | 58328 | 140.6 | 60.2% |
Wilkes | North Carolina | 97 | 43003 | 225.6 | 96.6% |
Wilson | North Carolina | 87 | 50219 | 173.2 | 74.2% |
Yadkin | North Carolina | 35 | 20657 | 169.4 | 72.6% |
Yancey | North Carolina | 29 | 17202 | 168.6 | 72.2% |
Adams | North Dakota | 8 | 4664 | 171.5 | 73.5% |
Barnes | North Dakota | 41 | 17814 | 230.2 | 98.6% |
Benson | North Dakota | 34 | 12629 | 269.2 | 115.3% |
Billings | North Dakota | 9 | 2531 | 355.6 | 152.3% |
Bottineau | North Dakota | 32 | 13253 | 241.5 | 103.4% |
Bowman | North Dakota | 13 | 3860 | 336.8 | 144.3% |
Burke | North Dakota | 21 | 7653 | 274.4 | 117.5% |
Burleigh | North Dakota | 47 | 22736 | 206.7 | 88.5% |
Cass | North Dakota | 147 | 52849 | 278.2 | 119.1% |
Cavalier | North Dakota | 33 | 13923 | 237.0 | 101.5% |
Dickey | North Dakota | 25 | 9696 | 257.8 | 110.4% |
Divide | North Dakota | 15 | 7086 | 211.7 | 90.7% |
Dunn | North Dakota | 24 | 8376 | 286.5 | 122.7% |
Eddy | North Dakota | 15 | 5741 | 261.3 | 111.9% |
Emmons | North Dakota | 24 | 11699 | 205.1 | 87.9% |
Foster | North Dakota | 15 | 5824 | 257.6 | 110.3% |
Golden Valley | North Dakota | 11 | 3498 | 314.5 | 134.7% |
Grand Forks | North Dakota | 95 | 34518 | 275.2 | 117.9% |
Grant | North Dakota | 13 | 8264 | 157.3 | 67.4% |
Griggs | North Dakota | 16 | 5818 | 275.0 | 117.8% |
Hettinger | North Dakota | 21 | 7457 | 281.6 | 120.6% |
Kidder | North Dakota | 16 | 6692 | 239.1 | 102.4% |
La Moure | North Dakota | 25 | 10298 | 242.8 | 104.0% |
Logan | North Dakota | 16 | 7561 | 211.6 | 90.6% |
Mchenry | North Dakota | 31 | 14034 | 220.9 | 94.6% |
Mcintosh | North Dakota | 20 | 8984 | 222.6 | 95.4% |
Mckenzie | North Dakota | 22 | 8426 | 261.1 | 111.8% |
Mclean | North Dakota | 45 | 16082 | 279.8 | 119.9% |
Mercer | North Dakota | 9 | 9611 | 93.6 | 40.1% |
Morton | North Dakota | 43 | 20184 | 213.0 | 91.3% |
Mountrail | North Dakota | 25 | 10482 | 238.5 | 102.2% |
Nelson | North Dakota | 24 | 9129 | 262.9 | 112.6% |
Oliver | North Dakota | 3 | 3859 | 77.7 | 33.3% |
Pembina | North Dakota | 38 | 15671 | 242.5 | 103.9% |
Pierce | North Dakota | 19 | 9208 | 206.3 | 88.4% |
Ramsey | North Dakota | 36 | 15626 | 230.4 | 98.7% |
Ransom | North Dakota | 28 | 10061 | 278.3 | 119.2% |
Renville | North Dakota | 15 | 5533 | 271.1 | 116.1% |
Richland | North Dakota | 41 | 20519 | 199.8 | 85.6% |
Rolette | North Dakota | 32 | 12583 | 254.3 | 108.9% |
Sargent | North Dakota | 22 | 8693 | 253.1 | 108.4% |
Sheridan | North Dakota | 13 | 6616 | 196.5 | 84.2% |
Sioux | North Dakota | 11 | 4419 | 248.9 | 106.6% |
Slope | North Dakota | 7 | 2932 | 238.7 | 102.3% |
St.ark | North Dakota | 39 | 15414 | 253.0 | 108.4% |
St.eele | North Dakota | 20 | 6193 | 322.9 | 138.3% |
St.utsman | North Dakota | 57 | 23495 | 242.6 | 103.9% |
Towner | North Dakota | 17 | 7200 | 236.1 | 101.1% |
Traill | North Dakota | 37 | 12300 | 300.8 | 128.9% |
Walsh | North Dakota | 66 | 20747 | 318.1 | 136.3% |
Ward | North Dakota | 82 | 31981 | 256.4 | 109.8% |
Wells | North Dakota | 33 | 11198 | 294.7 | 126.2% |
Williams | North Dakota | 58 | 16315 | 355.5 | 152.3% |
Adams | Nebraska | 64 | 24576 | 260.4 | 111.5% |
Antelope | Nebraska | 30 | 13289 | 225.8 | 96.7% |
Arthur | Nebraska | 1 | 1045 | 95.7 | 41.0% |
Banner | Nebraska | 0 | 1403 | 0.0 | 0.0% |
Blaine | Nebraska | 4 | 1538 | 260.1 | 111.4% |
Boone | Nebraska | 22 | 12127 | 181.4 | 77.7% |
Box Butte | Nebraska | 10 | 10736 | 93.1 | 39.9% |
Boyd | Nebraska | 7 | 6060 | 115.5 | 49.5% |
Brown | Nebraska | 12 | 5962 | 201.3 | 86.2% |
Buffalo | Nebraska | 48 | 23655 | 202.9 | 86.9% |
Burt | Nebraska | 33 | 12546 | 263.0 | 112.7% |
Butler | Nebraska | 27 | 13106 | 206.0 | 88.2% |
Cass | Nebraska | 37 | 16992 | 217.7 | 93.3% |
Cedar | Nebraska | 34 | 15126 | 224.8 | 96.3% |
Chase | Nebraska | 15 | 5310 | 282.5 | 121.0% |
Cherry | Nebraska | 32 | 9637 | 332.1 | 142.2% |
Cheyenne | Nebraska | 17 | 9505 | 178.9 | 76.6% |
Clay | Nebraska | 19 | 10445 | 181.9 | 77.9% |
Colfax | Nebraska | 31 | 10627 | 291.7 | 125.0% |
Cuming | Nebraska | 19 | 13562 | 140.1 | 60.0% |
Custer | Nebraska | 47 | 22591 | 208.0 | 89.1% |
Dakota | Nebraska | 24 | 9836 | 244.0 | 104.5% |
Dawes | Nebraska | 26 | 10128 | 256.7 | 110.0% |
Dawson | Nebraska | 54 | 17890 | 301.8 | 129.3% |
Deuel | Nebraska | 12 | 3580 | 335.2 | 143.6% |
Dixon | Nebraska | 18 | 10413 | 172.9 | 74.0% |
Dodge | Nebraska | 52 | 23799 | 218.5 | 93.6% |
Douglas | Nebraska | 595 | 247562 | 240.3 | 103.0% |
Dundy | Nebraska | 10 | 5122 | 195.2 | 83.6% |
Fillmore | Nebraska | 24 | 11417 | 210.2 | 90.0% |
Franklin | Nebraska | 19 | 7740 | 245.5 | 105.2% |
Frontier | Nebraska | 11 | 6417 | 171.4 | 73.4% |
Furnas | Nebraska | 24 | 10098 | 237.7 | 101.8% |
Gage | Nebraska | 75 | 29588 | 253.5 | 108.6% |
Garden | Nebraska | 12 | 4680 | 256.4 | 109.8% |
Garfield | Nebraska | 15 | 3444 | 435.5 | 186.6% |
Gosper | Nebraska | 10 | 3687 | 271.2 | 116.2% |
Grant | Nebraska | 9 | 1327 | 678.2 | 290.5% |
Greeley | Nebraska | 16 | 6845 | 233.7 | 100.1% |
Hall | Nebraska | 78 | 27523 | 283.4 | 121.4% |
Hamilton | Nebraska | 16 | 9982 | 160.3 | 68.7% |
Harlan | Nebraska | 8 | 7130 | 112.2 | 48.1% |
Hayes | Nebraska | 6 | 2958 | 202.8 | 86.9% |
Hitchcock | Nebraska | 16 | 6404 | 249.8 | 107.0% |
Holt | Nebraska | 48 | 16552 | 290.0 | 124.2% |
Hooker | Nebraska | 5 | 1253 | 399.0 | 170.9% |
Howard | Nebraska | 15 | 8422 | 178.1 | 76.3% |
Jefferson | Nebraska | 38 | 15532 | 244.7 | 104.8% |
Johnson | Nebraska | 19 | 8662 | 219.3 | 94.0% |
Kearney | Nebraska | 11 | 6854 | 160.5 | 68.7% |
Keith | Nebraska | 19 | 8333 | 228.0 | 97.7% |
Keya Paha | Nebraska | 5 | 3235 | 154.6 | 66.2% |
Kimball | Nebraska | 9 | 3913 | 230.0 | 98.5% |
Knox | Nebraska | 32 | 16478 | 194.2 | 83.2% |
Lancaster | Nebraska | 233 | 100585 | 231.6 | 99.2% |
Lincoln | Nebraska | 71 | 25425 | 279.3 | 119.6% |
Logan | Nebraska | 15 | 1742 | 861.1 | 368.8% |
Loup | Nebraska | 3 | 1777 | 168.8 | 72.3% |
Madison | Nebraska | 52 | 24269 | 214.3 | 91.8% |
Mcpherson | Nebraska | 1 | 1175 | 85.1 | 36.5% |
Merrick | Nebraska | 24 | 9354 | 256.6 | 109.9% |
Morrill | Nebraska | 21 | 9436 | 222.6 | 95.3% |
Nance | Nebraska | 11 | 7653 | 143.7 | 61.6% |
Nemaha | Nebraska | 45 | 12781 | 352.1 | 150.8% |
Nuckolls | Nebraska | 21 | 10446 | 201.0 | 86.1% |
Otoe | Nebraska | 39 | 18994 | 205.3 | 88.0% |
Pawnee | Nebraska | 16 | 8514 | 187.9 | 80.5% |
Perkins | Nebraska | 5 | 5197 | 96.2 | 41.2% |
Phelps | Nebraska | 11 | 8452 | 130.1 | 55.7% |
Pierce | Nebraska | 21 | 10211 | 205.7 | 88.1% |
Platte | Nebraska | 40 | 20191 | 198.1 | 84.9% |
Polk | Nebraska | 12 | 8748 | 137.2 | 58.8% |
Red Willow | Nebraska | 20 | 11951 | 167.4 | 71.7% |
Richardson | Nebraska | 44 | 19178 | 229.4 | 98.3% |
Rock | Nebraska | 9 | 3977 | 226.3 | 96.9% |
Saline | Nebraska | 33 | 15010 | 219.9 | 94.2% |
Sarpy | Nebraska | 16 | 10835 | 147.7 | 63.3% |
Saunders | Nebraska | 37 | 17892 | 206.8 | 88.6% |
Scotts Bluff | Nebraska | 59 | 33917 | 174.0 | 74.5% |
Seward | Nebraska | 29 | 14167 | 204.7 | 87.7% |
Sheridan | Nebraska | 22 | 9869 | 222.9 | 95.5% |
Sherman | Nebraska | 14 | 7764 | 180.3 | 77.2% |
Sioux | Nebraska | 3 | 4001 | 75.0 | 32.1% |
St.anton | Nebraska | 12 | 6887 | 174.2 | 74.6% |
Thayer | Nebraska | 32 | 12262 | 261.0 | 111.8% |
Thomas | Nebraska | 5 | 1553 | 322.0 | 137.9% |
Thurston | Nebraska | 22 | 10243 | 214.8 | 92.0% |
Valley | Nebraska | 15 | 8163 | 183.8 | 78.7% |
Washington | Nebraska | 25 | 11578 | 215.9 | 92.5% |
Wayne | Nebraska | 18 | 9880 | 182.2 | 78.0% |
Webster | Nebraska | 14 | 8071 | 173.5 | 74.3% |
Wheeler | Nebraska | 2 | 2170 | 92.2 | 39.5% |
York | Nebraska | 23 | 14874 | 154.6 | 66.2% |
Belknap | New Hampshire | 50 | 24328 | 205.5 | 88.0% |
Carroll | New Hampshire | 34 | 15589 | 218.1 | 93.4% |
Cheshire | New Hampshire | 92 | 34953 | 263.2 | 112.7% |
Coos | New Hampshire | 99 | 39274 | 252.1 | 108.0% |
Grafton | New Hampshire | 124 | 44645 | 277.7 | 119.0% |
Hillsborough | New Hampshire | 360 | 144888 | 248.5 | 106.4% |
Merrimack | New Hampshire | 113 | 60710 | 186.1 | 79.7% |
Rockingham | New Hampshire | 134 | 58142 | 230.5 | 98.7% |
St.rafford | New Hampshire | 108 | 43553 | 248.0 | 106.2% |
Sullivan | New Hampshire | 80 | 25442 | 314.4 | 134.7% |
Atlantic | New Jersey | 236 | 124066 | 190.2 | 81.5% |
Bergen | New Jersey | 1054 | 409646 | 257.3 | 110.2% |
Burlington | New Jersey | 243 | 97013 | 250.5 | 107.3% |
Camden | New Jersey | 608 | 255727 | 237.8 | 101.8% |
Cape May | New Jersey | 76 | 28919 | 262.8 | 112.6% |
Cumberland | New Jersey | 152 | 73184 | 207.7 | 89.0% |
Essex | New Jersey | 2000 | 837340 | 238.9 | 102.3% |
Gloucester | New Jersey | 183 | 72219 | 253.4 | 108.5% |
Hudson | New Jersey | 1602 | 652040 | 245.7 | 105.2% |
Hunterdon | New Jersey | 77 | 36766 | 209.4 | 89.7% |
Mercer | New Jersey | 517 | 197318 | 262.0 | 112.2% |
Middlesex | New Jersey | 673 | 217077 | 310.0 | 132.8% |
Monmouth | New Jersey | 355 | 161238 | 220.2 | 94.3% |
Morris | New Jersey | 302 | 125732 | 240.2 | 102.9% |
Ocean | New Jersey | 85 | 37706 | 225.4 | 96.6% |
Passaic | New Jersey | 759 | 309353 | 245.4 | 105.1% |
Salem | New Jersey | 91 | 42274 | 215.3 | 92.2% |
Somerset | New Jersey | 182 | 74390 | 244.7 | 104.8% |
Sussex | New Jersey | 87 | 29632 | 293.6 | 125.8% |
Union | New Jersey | 851 | 328344 | 259.2 | 111.0% |
Warren | New Jersey | 120 | 50181 | 239.1 | 102.4% |
Bernalillo | New Mexico | 354 | 69391 | 510.2 | 218.5% |
Catron | New Mexico | 14 | 4881 | 286.8 | 122.9% |
Chaves | New Mexico | 93 | 23980 | 387.8 | 166.1% |
Colfax | New Mexico | 61 | 18718 | 325.9 | 139.6% |
Curry | New Mexico | 84 | 18159 | 462.6 | 198.1% |
De Baca | New Mexico | 18 | 3725 | 483.2 | 207.0% |
Dona Ana | New Mexico | 98 | 30411 | 322.3 | 138.0% |
Eddy | New Mexico | 116 | 24311 | 477.2 | 204.4% |
Grant | New Mexico | 108 | 20050 | 538.7 | 230.7% |
Guadalupe | New Mexico | 29 | 8646 | 335.4 | 143.7% |
Harding | New Mexico | 13 | 4374 | 297.2 | 127.3% |
Hidalgo | New Mexico | 14 | 4821 | 290.4 | 124.4% |
Lea | New Mexico | 65 | 21154 | 307.3 | 131.6% |
Lincoln | New Mexico | 34 | 8557 | 397.3 | 170.2% |
Luna | New Mexico | 58 | 6457 | 898.2 | 384.8% |
Mckinley | New Mexico | 103 | 23641 | 435.7 | 186.6% |
Mora | New Mexico | 41 | 10981 | 373.4 | 159.9% |
Otero | New Mexico | 37 | 10522 | 351.6 | 150.6% |
Quay | New Mexico | 24 | 12111 | 198.2 | 84.9% |
Rio Arriba | New Mexico | 60 | 25352 | 236.7 | 101.4% |
Roosevelt | New Mexico | 45 | 14549 | 309.3 | 132.5% |
Sandoval | New Mexico | 41 | 13898 | 295.0 | 126.4% |
San Juan | New Mexico | 45 | 17115 | 262.9 | 112.6% |
San Miguel | New Mexico | 71 | 27910 | 254.4 | 109.0% |
Santa Fe | New Mexico | 117 | 30826 | 379.5 | 162.6% |
Sierra | New Mexico | 25 | 6962 | 359.1 | 153.8% |
Socorro | New Mexico | 36 | 11422 | 315.2 | 135.0% |
Taos | New Mexico | 69 | 18528 | 372.4 | 159.5% |
Torrance | New Mexico | 33 | 11026 | 299.3 | 128.2% |
Union | New Mexico | 31 | 9095 | 340.8 | 146.0% |
Valencia | New Mexico | 82 | 20245 | 405.0 | 173.5% |
Churchill | Nevada | 20 | 5317 | 376.2 | 161.1% |
Clark | Nevada | 69 | 16414 | 420.4 | 180.1% |
Douglas | Nevada | 7 | 2056 | 340.5 | 145.8% |
Elko | Nevada | 32 | 10912 | 293.3 | 125.6% |
Esmeralda | Nevada | 0 | 1554 | 0.0 | 0.0% |
Eureka | Nevada | 4 | 1361 | 293.9 | 125.9% |
Humboldt | Nevada | 12 | 4743 | 253.0 | 108.4% |
Lander | Nevada | 6 | 1745 | 343.8 | 147.3% |
Lincoln | Nevada | 12 | 4130 | 290.6 | 124.5% |
Lyon | Nevada | 11 | 4076 | 269.9 | 115.6% |
Mineral | Nevada | 16 | 2342 | 683.2 | 292.6% |
Nye | Nevada | 11 | 3606 | 305.0 | 130.7% |
Pershing | Nevada | 8 | 2713 | 294.9 | 126.3% |
St.orey | Nevada | 3 | 1216 | 246.7 | 105.7% |
Washoe | Nevada | 88 | 32476 | 271.0 | 116.1% |
White Pine | Nevada | 25 | 12377 | 202.0 | 86.5% |
Albany | New York | 556 | 221315 | 251.2 | 107.6% |
Allegany | New York | 125 | 39681 | 315.0 | 134.9% |
Bronx | New York | 2014 | 1394711 | 144.4 | 61.9% |
Broome | New York | 438 | 165749 | 264.3 | 113.2% |
Cattaraugus | New York | 191 | 72652 | 262.9 | 112.6% |
Cayuga | New York | 172 | 65508 | 262.6 | 112.5% |
Chautauqua | New York | 345 | 123580 | 279.2 | 119.6% |
Chemung | New York | 194 | 73718 | 263.2 | 112.7% |
Chenango | New York | 103 | 36454 | 282.5 | 121.0% |
Clinton | New York | 118 | 54006 | 218.5 | 93.6% |
Columbia | New York | 96 | 41464 | 231.5 | 99.2% |
Cortland | New York | 73 | 33668 | 216.8 | 92.9% |
Delaware | New York | 110 | 40989 | 268.4 | 115.0% |
Dutchess | New York | 314 | 120542 | 260.5 | 111.6% |
Erie | New York | 2077 | 798377 | 260.2 | 111.4% |
Essex | New York | 114 | 34178 | 333.5 | 142.9% |
Franklin | New York | 104 | 44286 | 234.8 | 100.6% |
Fulton | New York | 108 | 48597 | 222.2 | 95.2% |
Genesee | New York | 113 | 44481 | 254.0 | 108.8% |
Greene | New York | 77 | 27926 | 275.7 | 118.1% |
Hamilton | New York | 22 | 4188 | 525.3 | 225.0% |
Herkimer | New York | 165 | 59527 | 277.2 | 118.7% |
Jefferson | New York | 220 | 84003 | 261.9 | 112.2% |
Kings | New York | 5840 | 2698285 | 216.4 | 92.7% |
Lewis | New York | 61 | 22815 | 267.4 | 114.5% |
Livingston | New York | 71 | 38510 | 184.4 | 79.0% |
Madison | New York | 103 | 39598 | 260.1 | 111.4% |
Monroe | New York | 1051 | 438230 | 239.8 | 102.7% |
Montgomery | New York | 175 | 59142 | 295.9 | 126.7% |
Nassau | New York | 855 | 406748 | 210.2 | 90.0% |
New York | New York | 4910 | 1889924 | 259.8 | 111.3% |
Niagara | New York | 515 | 160110 | 321.7 | 137.8% |
Oneida | New York | 534 | 203636 | 262.2 | 112.3% |
Onondaga | New York | 787 | 295108 | 266.7 | 114.2% |
Ontario | New York | 129 | 55307 | 233.2 | 99.9% |
Orange | New York | 305 | 140113 | 217.7 | 93.2% |
Orleans | New York | 100 | 27760 | 360.2 | 154.3% |
Oswego | New York | 192 | 71275 | 269.4 | 115.4% |
Otsego | New York | 110 | 46082 | 238.7 | 102.2% |
Putnam | New York | 44 | 16555 | 265.8 | 113.8% |
Queens | New York | 2997 | 1297634 | 231.0 | 98.9% |
Rensselaer | New York | 282 | 121834 | 231.5 | 99.1% |
Richmond | New York | 346 | 174441 | 198.3 | 85.0% |
Rockland | New York | 158 | 74261 | 212.8 | 91.1% |
Saratoga | New York | 178 | 65606 | 271.3 | 116.2% |
Schenectady | New York | 278 | 122494 | 226.9 | 97.2% |
Schoharie | New York | 35 | 20812 | 168.2 | 72.0% |
Schuyler | New York | 37 | 12979 | 285.1 | 122.1% |
Seneca | New York | 50 | 25732 | 194.3 | 83.2% |
St.euben | New York | 232 | 84927 | 273.2 | 117.0% |
St Lawrence | New York | 246 | 91098 | 270.0 | 115.7% |
Suffolk | New York | 325 | 197355 | 164.7 | 70.5% |
Sullivan | New York | 91 | 37901 | 240.1 | 102.8% |
Tioga | New York | 63 | 27072 | 232.7 | 99.7% |
Tompkins | New York | 97 | 42340 | 229.1 | 98.1% |
Ulster | New York | 197 | 87017 | 226.4 | 97.0% |
Warren | New York | 96 | 36035 | 266.4 | 114.1% |
Washington | New York | 113 | 46726 | 241.8 | 103.6% |
Wayne | New York | 140 | 52747 | 265.4 | 113.7% |
Westchester | New York | 1295 | 573558 | 225.8 | 96.7% |
Wyoming | New York | 60 | 31394 | 191.1 | 81.9% |
Yates | New York | 49 | 16381 | 299.1 | 128.1% |
Adams | Ohio | 58 | 21705 | 267.2 | 114.5% |
Allen | Ohio | 171 | 73303 | 233.3 | 99.9% |
Ashland | Ohio | 75 | 29785 | 251.8 | 107.9% |
Ashtabula | Ohio | 160 | 68674 | 233.0 | 99.8% |
Athens | Ohio | 129 | 46166 | 279.4 | 119.7% |
Auglaize | Ohio | 72 | 28037 | 256.8 | 110.0% |
Belmont | Ohio | 252 | 95614 | 263.6 | 112.9% |
Brown | Ohio | 43 | 21638 | 198.7 | 85.1% |
Butler | Ohio | 307 | 120249 | 255.3 | 109.4% |
Carroll | Ohio | 41 | 17449 | 235.0 | 100.6% |
Champaign | Ohio | 47 | 25258 | 186.1 | 79.7% |
Clark | Ohio | 218 | 95647 | 227.9 | 97.6% |
Clermont | Ohio | 71 | 34109 | 208.2 | 89.2% |
Clinton | Ohio | 48 | 22574 | 212.6 | 91.1% |
Columbiana | Ohio | 247 | 90121 | 274.1 | 117.4% |
Coshocton | Ohio | 68 | 30594 | 222.3 | 95.2% |
Crawford | Ohio | 74 | 35571 | 208.0 | 89.1% |
Cuyahoga | Ohio | 3004 | 1217250 | 246.8 | 105.7% |
Darke | Ohio | 91 | 38831 | 234.3 | 100.4% |
Defiance | Ohio | 52 | 24367 | 213.4 | 91.4% |
Delaware | Ohio | 53 | 26780 | 197.9 | 84.8% |
Erie | Ohio | 89 | 43201 | 206.0 | 88.2% |
Fairfield | Ohio | 96 | 48490 | 198.0 | 84.8% |
Fayette | Ohio | 60 | 21385 | 280.6 | 120.2% |
Franklin | Ohio | 890 | 388712 | 229.0 | 98.1% |
Fulton | Ohio | 54 | 23626 | 228.6 | 97.9% |
Gallia | Ohio | 61 | 24930 | 244.7 | 104.8% |
Geauga | Ohio | 45 | 19430 | 231.6 | 99.2% |
Greene | Ohio | 77 | 35863 | 214.7 | 92.0% |
Guernsey | Ohio | 82 | 38822 | 211.2 | 90.5% |
Hamilton | Ohio | 1357 | 621987 | 218.2 | 93.5% |
Hancock | Ohio | 94 | 40793 | 230.4 | 98.7% |
Hardin | Ohio | 77 | 27061 | 284.5 | 121.9% |
Harrison | Ohio | 57 | 20313 | 280.6 | 120.2% |
Henry | Ohio | 66 | 22756 | 290.0 | 124.2% |
Highland | Ohio | 59 | 27099 | 217.7 | 93.3% |
Hocking | Ohio | 43 | 21504 | 200.0 | 85.7% |
Holmes | Ohio | 34 | 17876 | 190.2 | 81.5% |
Huron | Ohio | 97 | 34800 | 278.7 | 119.4% |
Jackson | Ohio | 61 | 27004 | 225.9 | 96.8% |
Jefferson | Ohio | 270 | 98129 | 275.1 | 117.9% |
Knox | Ohio | 77 | 31024 | 248.2 | 106.3% |
Lake | Ohio | 131 | 50020 | 261.9 | 112.2% |
Lawrence | Ohio | 89 | 46705 | 190.6 | 81.6% |
Licking | Ohio | 122 | 62279 | 195.9 | 83.9% |
Logan | Ohio | 52 | 29624 | 175.5 | 75.2% |
Lorain | Ohio | 321 | 112390 | 285.6 | 122.3% |
Lucas | Ohio | 763 | 344333 | 221.6 | 94.9% |
Madison | Ohio | 54 | 21811 | 247.6 | 106.1% |
Mahoning | Ohio | 654 | 240251 | 272.2 | 116.6% |
Marion | Ohio | 109 | 44898 | 242.8 | 104.0% |
Medina | Ohio | 64 | 33034 | 193.7 | 83.0% |
Meigs | Ohio | 70 | 24104 | 290.4 | 124.4% |
Mercer | Ohio | 60 | 26256 | 228.5 | 97.9% |
Miami | Ohio | 144 | 52632 | 273.6 | 117.2% |
Monroe | Ohio | 48 | 18641 | 257.5 | 110.3% |
Montgomery | Ohio | 688 | 295480 | 232.8 | 99.7% |
Morgan | Ohio | 25 | 14227 | 175.7 | 75.3% |
Morrow | Ohio | 25 | 15646 | 159.8 | 68.4% |
Muskingum | Ohio | 166 | 69795 | 237.8 | 101.9% |
Noble | Ohio | 26 | 14587 | 178.2 | 76.3% |
Ottawa | Ohio | 103 | 24360 | 422.8 | 181.1% |
Paulding | Ohio | 32 | 15527 | 206.1 | 88.3% |
Perry | Ohio | 79 | 31087 | 254.1 | 108.9% |
Pickaway | Ohio | 46 | 27889 | 164.9 | 70.7% |
Pike | Ohio | 36 | 16113 | 223.4 | 95.7% |
Portage | Ohio | 139 | 46660 | 297.9 | 127.6% |
Preble | Ohio | 77 | 23329 | 330.1 | 141.4% |
Putnam | Ohio | 50 | 25016 | 199.9 | 85.6% |
Richland | Ohio | 168 | 73853 | 227.5 | 97.4% |
Ross | Ohio | 111 | 52147 | 212.9 | 91.2% |
Sandusky | Ohio | 91 | 41014 | 221.9 | 95.0% |
Scioto | Ohio | 183 | 86565 | 211.4 | 90.6% |
Seneca | Ohio | 105 | 48499 | 216.5 | 92.7% |
Shelby | Ohio | 74 | 26071 | 283.8 | 121.6% |
St.ark | Ohio | 673 | 234887 | 286.5 | 122.7% |
Summit | Ohio | 940 | 339405 | 277.0 | 118.6% |
Trumbull | Ohio | 397 | 132315 | 300.0 | 128.5% |
Tuscarawas | Ohio | 168 | 68816 | 244.1 | 104.6% |
Union | Ohio | 43 | 20012 | 214.9 | 92.0% |
Van Wert | Ohio | 71 | 26759 | 265.3 | 113.7% |
Vinton | Ohio | 26 | 11573 | 224.7 | 96.2% |
Warren | Ohio | 75 | 29894 | 250.9 | 107.5% |
Washington | Ohio | 114 | 43537 | 261.8 | 112.2% |
Wayne | Ohio | 97 | 50520 | 192.0 | 82.2% |
Williams | Ohio | 45 | 25510 | 176.4 | 75.6% |
Wood | Ohio | 115 | 51796 | 222.0 | 95.1% |
Wyandot | Ohio | 42 | 19218 | 218.5 | 93.6% |
Adair | Oklahoma | 46 | 15755 | 292.0 | 125.1% |
Alfalfa | Oklahoma | 30 | 14129 | 212.3 | 91.0% |
Atoka | Oklahoma | 41 | 18702 | 219.2 | 93.9% |
Beaver | Oklahoma | 17 | 8648 | 196.6 | 84.2% |
Beckham | Oklahoma | 55 | 22169 | 248.1 | 106.3% |
Blaine | Oklahoma | 39 | 18543 | 210.3 | 90.1% |
Bryan | Oklahoma | 83 | 38138 | 217.6 | 93.2% |
Caddo | Oklahoma | 89 | 41567 | 214.1 | 91.7% |
Canadian | Oklahoma | 48 | 27329 | 175.6 | 75.2% |
Carter | Oklahoma | 85 | 43292 | 196.3 | 84.1% |
Cherokee | Oklahoma | 63 | 21030 | 299.6 | 128.3% |
Choctaw | Oklahoma | 58 | 28358 | 204.5 | 87.6% |
Cimarron | Oklahoma | 15 | 3654 | 410.5 | 175.8% |
Cleveland | Oklahoma | 75 | 27728 | 270.5 | 115.9% |
Coal | Oklahoma | 16 | 12811 | 124.9 | 53.5% |
Comanche | Oklahoma | 93 | 38988 | 238.5 | 102.2% |
Cotton | Oklahoma | 32 | 12884 | 248.4 | 106.4% |
Craig | Oklahoma | 50 | 21083 | 237.2 | 101.6% |
Creek | Oklahoma | 118 | 55503 | 212.6 | 91.1% |
Custer | Oklahoma | 60 | 23068 | 260.1 | 111.4% |
Delaware | Oklahoma | 25 | 18592 | 134.5 | 57.6% |
Dewey | Oklahoma | 30 | 11981 | 250.4 | 107.3% |
Ellis | Oklahoma | 28 | 8466 | 330.7 | 141.7% |
Garfield | Oklahoma | 119 | 45484 | 261.6 | 112.1% |
Garvin | Oklahoma | 74 | 31150 | 237.6 | 101.8% |
Grady | Oklahoma | 79 | 41116 | 192.1 | 82.3% |
Grant | Oklahoma | 34 | 13128 | 259.0 | 110.9% |
Greer | Oklahoma | 36 | 14550 | 247.4 | 106.0% |
Harmon | Oklahoma | 30 | 10019 | 299.4 | 128.3% |
Harper | Oklahoma | 16 | 6454 | 247.9 | 106.2% |
Haskell | Oklahoma | 43 | 17324 | 248.2 | 106.3% |
Hughes | Oklahoma | 71 | 29189 | 243.2 | 104.2% |
Jackson | Oklahoma | 64 | 22708 | 281.8 | 120.7% |
Jefferson | Oklahoma | 32 | 15107 | 211.8 | 90.7% |
Johnston | Oklahoma | 31 | 15960 | 194.2 | 83.2% |
Kay | Oklahoma | 131 | 47084 | 278.2 | 119.2% |
Kingfisher | Oklahoma | 20 | 15617 | 128.1 | 54.9% |
Kiowa | Oklahoma | 44 | 22817 | 192.8 | 82.6% |
Latimer | Oklahoma | 31 | 12380 | 250.4 | 107.3% |
Le Flore | Oklahoma | 105 | 45866 | 228.9 | 98.1% |
Lincoln | Oklahoma | 69 | 29529 | 233.7 | 100.1% |
Logan | Oklahoma | 49 | 25245 | 194.1 | 83.1% |
Love | Oklahoma | 21 | 11433 | 183.7 | 78.7% |
Major | Oklahoma | 25 | 11946 | 209.3 | 89.6% |
Marshall | Oklahoma | 24 | 12384 | 193.8 | 83.0% |
Mayes | Oklahoma | 54 | 21668 | 249.2 | 106.8% |
Mcclain | Oklahoma | 43 | 19205 | 223.9 | 95.9% |
Mccurtain | Oklahoma | 94 | 41318 | 227.5 | 97.5% |
Mcintosh | Oklahoma | 51 | 24097 | 211.6 | 90.7% |
Murray | Oklahoma | 21 | 13841 | 151.7 | 65.0% |
Muskogee | Oklahoma | 144 | 65914 | 218.5 | 93.6% |
Noble | Oklahoma | 42 | 14826 | 283.3 | 121.3% |
Nowata | Oklahoma | 41 | 15774 | 259.9 | 111.3% |
Okfuskee | Oklahoma | 58 | 26279 | 220.7 | 94.5% |
Oklahoma | Oklahoma | 580 | 244159 | 237.6 | 101.8% |
Okmulgee | Oklahoma | 128 | 50101 | 255.5 | 109.4% |
Osage | Oklahoma | 106 | 41502 | 255.4 | 109.4% |
Ottawa | Oklahoma | 77 | 35849 | 214.8 | 92.0% |
Pawnee | Oklahoma | 55 | 17395 | 316.2 | 135.4% |
Payne | Oklahoma | 107 | 36057 | 296.8 | 127.1% |
Pittsburg | Oklahoma | 107 | 48985 | 218.4 | 93.6% |
Pontotoc | Oklahoma | 103 | 39792 | 258.8 | 110.9% |
Pottawatomie | Oklahoma | 107 | 54377 | 196.8 | 84.3% |
Pushmataha | Oklahoma | 55 | 19466 | 282.5 | 121.0% |
Roger Mills | Oklahoma | 19 | 10736 | 177.0 | 75.8% |
Rogers | Oklahoma | 49 | 21078 | 232.5 | 99.6% |
Seminole | Oklahoma | 113 | 61201 | 184.6 | 79.1% |
Sequoyah | Oklahoma | 49 | 23138 | 211.8 | 90.7% |
St.ephens | Oklahoma | 63 | 31090 | 202.6 | 86.8% |
Texas | Oklahoma | 26 | 9896 | 262.7 | 112.5% |
Tillman | Oklahoma | 34 | 20754 | 163.8 | 70.2% |
Tulsa | Oklahoma | 511 | 193363 | 264.3 | 113.2% |
Wagoner | Oklahoma | 45 | 21642 | 207.9 | 89.1% |
Washington | Oklahoma | 91 | 30559 | 297.8 | 127.6% |
Washita | Oklahoma | 41 | 22279 | 184.0 | 78.8% |
Woods | Oklahoma | 43 | 14915 | 288.3 | 123.5% |
Woodward | Oklahoma | 36 | 16270 | 221.3 | 94.8% |
Baker | Oregon | 42 | 18297 | 229.5 | 98.3% |
Benton | Oregon | 55 | 18629 | 295.2 | 126.5% |
Clackamas | Oregon | 111 | 57130 | 194.3 | 83.2% |
Clatsop | Oregon | 45 | 24697 | 182.2 | 78.0% |
Columbia | Oregon | 50 | 20971 | 238.4 | 102.1% |
Coos | Oregon | 75 | 32466 | 231.0 | 99.0% |
Crook | Oregon | 14 | 5533 | 253.0 | 108.4% |
Curry | Oregon | 12 | 4301 | 279.0 | 119.5% |
Deschutes | Oregon | 49 | 18631 | 263.0 | 112.7% |
Douglas | Oregon | 67 | 25728 | 260.4 | 111.5% |
Gilliam | Oregon | 7 | 2844 | 246.1 | 105.4% |
Grant | Oregon | 14 | 6380 | 219.4 | 94.0% |
Harney | Oregon | 11 | 5374 | 204.7 | 87.7% |
Hood River | Oregon | 21 | 11580 | 181.3 | 77.7% |
Jackson | Oregon | 116 | 36213 | 320.3 | 137.2% |
Jefferson | Oregon | 11 | 2042 | 538.7 | 230.7% |
Josephine | Oregon | 38 | 16301 | 233.1 | 99.9% |
Klamath | Oregon | 113 | 40497 | 279.0 | 119.5% |
Lake | Oregon | 21 | 6293 | 333.7 | 142.9% |
Lane | Oregon | 177 | 69096 | 256.2 | 109.7% |
Lincoln | Oregon | 28 | 14549 | 192.5 | 82.4% |
Linn | Oregon | 83 | 30485 | 272.3 | 116.6% |
Malheur | Oregon | 45 | 19767 | 227.7 | 97.5% |
Marion | Oregon | 163 | 75246 | 216.6 | 92.8% |
Morrow | Oregon | 13 | 4337 | 299.7 | 128.4% |
Multnomah | Oregon | 965 | 355099 | 271.8 | 116.4% |
Polk | Oregon | 37 | 19989 | 185.1 | 79.3% |
Sherman | Oregon | 13 | 2321 | 560.1 | 239.9% |
Tillamook | Oregon | 49 | 12263 | 399.6 | 171.2% |
Umatilla | Oregon | 86 | 26030 | 330.4 | 141.5% |
Union | Oregon | 37 | 17399 | 212.7 | 91.1% |
Wallowa | Oregon | 19 | 7623 | 249.2 | 106.8% |
Wasco | Oregon | 22 | 13069 | 168.3 | 72.1% |
Washington | Oregon | 92 | 39194 | 234.7 | 100.5% |
Wheeler | Oregon | 13 | 2974 | 437.1 | 187.2% |
Yamhill | Oregon | 72 | 26336 | 273.4 | 117.1% |
Adams | Pennsylvania | 110 | 39435 | 278.9 | 119.5% |
Allegheny | Pennsylvania | 3982 | 1411539 | 282.1 | 120.8% |
Armstrong | Pennsylvania | 264 | 81087 | 325.6 | 139.5% |
Beaver | Pennsylvania | 479 | 156754 | 305.6 | 130.9% |
Bedford | Pennsylvania | 109 | 40809 | 267.1 | 114.4% |
Berks | Pennsylvania | 619 | 241884 | 255.9 | 109.6% |
Blair | Pennsylvania | 453 | 140358 | 322.7 | 138.2% |
Bradford | Pennsylvania | 118 | 50615 | 233.1 | 99.9% |
Bucks | Pennsylvania | 264 | 107715 | 245.1 | 105.0% |
Butler | Pennsylvania | 228 | 87590 | 260.3 | 111.5% |
Cambria | Pennsylvania | 696 | 213459 | 326.1 | 139.7% |
Cameron | Pennsylvania | 26 | 6852 | 379.5 | 162.5% |
Carbon | Pennsylvania | 215 | 61735 | 348.3 | 149.2% |
Centre | Pennsylvania | 136 | 52608 | 258.5 | 110.7% |
Chester | Pennsylvania | 314 | 135626 | 231.5 | 99.2% |
Clarion | Pennsylvania | 112 | 38410 | 291.6 | 124.9% |
Clearfield | Pennsylvania | 263 | 92094 | 285.6 | 122.3% |
Clinton | Pennsylvania | 115 | 34557 | 332.8 | 142.5% |
Columbia | Pennsylvania | 175 | 51413 | 340.4 | 145.8% |
Crawford | Pennsylvania | 166 | 71644 | 231.7 | 99.2% |
Cumberland | Pennsylvania | 199 | 74806 | 266.0 | 113.9% |
Dauphin | Pennsylvania | 447 | 177410 | 252.0 | 107.9% |
Delaware | Pennsylvania | 754 | 310756 | 242.6 | 103.9% |
Elk | Pennsylvania | 103 | 34443 | 299.0 | 128.1% |
Erie | Pennsylvania | 514 | 180889 | 284.2 | 121.7% |
Fayette | Pennsylvania | 639 | 200999 | 317.9 | 136.2% |
Forest | Pennsylvania | 17 | 5791 | 293.6 | 125.7% |
Franklin | Pennsylvania | 184 | 69378 | 265.2 | 113.6% |
Fulton | Pennsylvania | 27 | 10673 | 253.0 | 108.4% |
Greene | Pennsylvania | 123 | 44671 | 275.3 | 117.9% |
Huntingdon | Pennsylvania | 129 | 41836 | 308.3 | 132.1% |
Indiana | Pennsylvania | 262 | 79854 | 328.1 | 140.5% |
Jefferson | Pennsylvania | 157 | 54090 | 290.3 | 124.3% |
Juniata | Pennsylvania | 39 | 15373 | 253.7 | 108.7% |
Lackawanna | Pennsylvania | 846 | 301243 | 280.8 | 120.3% |
Lancaster | Pennsylvania | 453 | 212504 | 213.2 | 91.3% |
Lawrence | Pennsylvania | 280 | 96877 | 289.0 | 123.8% |
Lebanon | Pennsylvania | 204 | 72641 | 280.8 | 120.3% |
Lehigh | Pennsylvania | 391 | 177533 | 220.2 | 94.3% |
Luzerne | Pennsylvania | 1216 | 441518 | 275.4 | 118.0% |
Lycoming | Pennsylvania | 252 | 93633 | 269.1 | 115.3% |
Mckean | Pennsylvania | 164 | 56673 | 289.4 | 124.0% |
Mercer | Pennsylvania | 239 | 101039 | 236.5 | 101.3% |
Mifflin | Pennsylvania | 97 | 42993 | 225.6 | 96.6% |
Monroe | Pennsylvania | 81 | 29802 | 271.8 | 116.4% |
Montgomery | Pennsylvania | 654 | 289247 | 226.1 | 96.9% |
Montour | Pennsylvania | 43 | 15466 | 278.0 | 119.1% |
Northampton | Pennsylvania | 474 | 168959 | 280.5 | 120.2% |
Northumberland | Pennsylvania | 407 | 126887 | 320.8 | 137.4% |
Perry | Pennsylvania | 56 | 23213 | 241.2 | 103.3% |
Philadelphia | Pennsylvania | 4392 | 1931334 | 227.4 | 97.4% |
Pike | Pennsylvania | 23 | 7452 | 308.6 | 132.2% |
Potter | Pennsylvania | 33 | 18201 | 181.3 | 77.7% |
Schuylkill | Pennsylvania | 680 | 228331 | 297.8 | 127.6% |
Snyder | Pennsylvania | 50 | 20208 | 247.4 | 106.0% |
Somerset | Pennsylvania | 281 | 84957 | 330.8 | 141.7% |
Sullivan | Pennsylvania | 27 | 7504 | 359.8 | 154.1% |
Susquehanna | Pennsylvania | 88 | 33893 | 259.6 | 111.2% |
Tioga | Pennsylvania | 103 | 35004 | 294.3 | 126.0% |
Union | Pennsylvania | 41 | 20247 | 202.5 | 86.7% |
Venango | Pennsylvania | 143 | 63958 | 223.6 | 95.8% |
Warren | Pennsylvania | 123 | 42789 | 287.5 | 123.1% |
Washington | Pennsylvania | 649 | 210852 | 307.8 | 131.8% |
Wayne | Pennsylvania | 81 | 29934 | 270.6 | 115.9% |
Westmoreland | Pennsylvania | 940 | 303411 | 309.8 | 132.7% |
Wyoming | Pennsylvania | 36 | 16702 | 215.5 | 92.3% |
York | Pennsylvania | 396 | 178022 | 222.4 | 95.3% |
Bristol | Rhode Island | 59 | 25548 | 230.9 | 98.9% |
Kent | Rhode Island | 160 | 58311 | 274.4 | 117.5% |
Newport | Rhode Island | 90 | 46696 | 192.7 | 82.6% |
Providence | Rhode Island | 1258 | 550298 | 228.6 | 97.9% |
Washington | Rhode Island | 76 | 32493 | 233.9 | 100.2% |
Abbeville | South Carolina | 47 | 22931 | 205.0 | 87.8% |
Aiken | South Carolina | 67 | 49916 | 134.2 | 57.5% |
Allendale | South Carolina | 18 | 13040 | 138.0 | 59.1% |
Anderson | South Carolina | 181 | 88712 | 204.0 | 87.4% |
Bamberg | South Carolina | 18 | 18643 | 96.6 | 41.4% |
Barnwell | South Carolina | 19 | 20138 | 94.3 | 40.4% |
Beaufort | South Carolina | 31 | 22037 | 140.7 | 60.3% |
Berkeley | South Carolina | 28 | 27128 | 103.2 | 44.2% |
Calhoun | South Carolina | 16 | 16229 | 98.6 | 42.2% |
Charleston | South Carolina | 166 | 121105 | 137.1 | 58.7% |
Cherokee | South Carolina | 72 | 33290 | 216.3 | 92.6% |
Chester | South Carolina | 50 | 32579 | 153.5 | 65.7% |
Chesterfield | South Carolina | 59 | 35963 | 164.1 | 70.3% |
Clarendon | South Carolina | 45 | 31500 | 142.9 | 61.2% |
Colleton | South Carolina | 46 | 26268 | 175.1 | 75.0% |
Darlington | South Carolina | 77 | 45198 | 170.4 | 73.0% |
Dillon | South Carolina | 43 | 29625 | 145.1 | 62.2% |
Dorchester | South Carolina | 28 | 19928 | 140.5 | 60.2% |
Edgefield | South Carolina | 19 | 17894 | 106.2 | 45.5% |
Fairfield | South Carolina | 36 | 24187 | 148.8 | 63.8% |
Florence | South Carolina | 116 | 70582 | 164.3 | 70.4% |
Georgetown | South Carolina | 29 | 26352 | 110.0 | 47.1% |
Greenville | South Carolina | 324 | 136580 | 237.2 | 101.6% |
Greenwood | South Carolina | 90 | 40083 | 224.5 | 96.2% |
Hampton | South Carolina | 26 | 17465 | 148.9 | 63.8% |
Horry | South Carolina | 70 | 51951 | 134.7 | 57.7% |
Jasper | South Carolina | 11 | 11011 | 99.9 | 42.8% |
Kershaw | South Carolina | 69 | 32913 | 209.6 | 89.8% |
Lancaster | South Carolina | 64 | 33542 | 190.8 | 81.7% |
Laurens | South Carolina | 100 | 44185 | 226.3 | 96.9% |
Lee | South Carolina | 22 | 24908 | 88.3 | 37.8% |
Lexington | South Carolina | 76 | 35994 | 211.1 | 90.4% |
Marion | South Carolina | 58 | 30107 | 192.6 | 82.5% |
Marlboro | South Carolina | 49 | 33281 | 147.2 | 63.1% |
Mccormick | South Carolina | 18 | 10367 | 173.6 | 74.4% |
Newberry | South Carolina | 65 | 33577 | 193.6 | 82.9% |
Oconee | South Carolina | 104 | 36512 | 284.8 | 122.0% |
Orangeburg | South Carolina | 67 | 63707 | 105.2 | 45.0% |
Pickens | South Carolina | 97 | 37111 | 261.4 | 112.0% |
Richland | South Carolina | 199 | 104843 | 189.8 | 81.3% |
Saluda | South Carolina | 27 | 17192 | 157.0 | 67.3% |
Spartanburg | South Carolina | 316 | 127733 | 247.4 | 106.0% |
Sumter | South Carolina | 71 | 52463 | 135.3 | 58.0% |
Union | South Carolina | 72 | 31360 | 229.6 | 98.3% |
Williamsburg | South Carolina | 51 | 41011 | 124.4 | 53.3% |
York | South Carolina | 115 | 58663 | 196.0 | 84.0% |
Armstrong | South Dakota | 1 | 42 | 2381.0 | 1019.9% |
Aurora | South Dakota | 8 | 5387 | 148.5 | 63.6% |
Beadle | South Dakota | 50 | 19648 | 254.5 | 109.0% |
Bennett | South Dakota | 14 | 3983 | 351.5 | 150.6% |
Bon Homme | South Dakota | 21 | 10241 | 205.1 | 87.8% |
Brookings | South Dakota | 50 | 16560 | 301.9 | 129.3% |
Brown | South Dakota | 55 | 29676 | 185.3 | 79.4% |
Brule | South Dakota | 12 | 6195 | 193.7 | 83.0% |
Buffalo | South Dakota | 4 | 1853 | 215.9 | 92.5% |
Butte | South Dakota | 21 | 8004 | 262.4 | 112.4% |
Campbell | South Dakota | 7 | 5033 | 139.1 | 59.6% |
Charles Mix | South Dakota | 19 | 13449 | 141.3 | 60.5% |
Clark | South Dakota | 14 | 8955 | 156.3 | 67.0% |
Clay | South Dakota | 16 | 9592 | 166.8 | 71.5% |
Codington | South Dakota | 36 | 17014 | 211.6 | 90.6% |
Corson | South Dakota | 16 | 6755 | 236.9 | 101.5% |
Custer | South Dakota | 21 | 6023 | 348.7 | 149.3% |
Davison | South Dakota | 35 | 15336 | 228.2 | 97.8% |
Day | South Dakota | 37 | 13565 | 272.8 | 116.8% |
Deuel | South Dakota | 15 | 8450 | 177.5 | 76.0% |
Dewey | South Dakota | 16 | 5709 | 280.3 | 120.0% |
Douglas | South Dakota | 15 | 6348 | 236.3 | 101.2% |
Edmunds | South Dakota | 19 | 7814 | 243.2 | 104.2% |
Fall River | South Dakota | 28 | 8089 | 346.1 | 148.3% |
Faulk | South Dakota | 13 | 5168 | 251.5 | 107.8% |
Grant | South Dakota | 17 | 10552 | 161.1 | 69.0% |
Gregory | South Dakota | 19 | 9554 | 198.9 | 85.2% |
Haakon | South Dakota | 6 | 3515 | 170.7 | 73.1% |
Hamlin | South Dakota | 13 | 7562 | 171.9 | 73.6% |
Hand | South Dakota | 19 | 7166 | 265.1 | 113.6% |
Hanson | South Dakota | 10 | 5400 | 185.2 | 79.3% |
Harding | South Dakota | 8 | 3010 | 265.8 | 113.8% |
Hughes | South Dakota | 10 | 6624 | 151.0 | 64.7% |
Hutchinson | South Dakota | 26 | 12668 | 205.2 | 87.9% |
Hyde | South Dakota | 7 | 3113 | 224.9 | 96.3% |
Jackson | South Dakota | 3 | 1955 | 153.5 | 65.7% |
Jerauld | South Dakota | 14 | 4752 | 294.6 | 126.2% |
Jones | South Dakota | 10 | 2509 | 398.6 | 170.7% |
Kingsbury | South Dakota | 28 | 10831 | 258.5 | 110.7% |
Lake | South Dakota | 39 | 12412 | 314.2 | 134.6% |
Lawrence | South Dakota | 46 | 19093 | 240.9 | 103.2% |
Lincoln | South Dakota | 15 | 13171 | 113.9 | 48.8% |
Lyman | South Dakota | 10 | 5045 | 198.2 | 84.9% |
Marshall | South Dakota | 22 | 8880 | 247.7 | 106.1% |
Mccook | South Dakota | 20 | 9793 | 204.2 | 87.5% |
Mcpherson | South Dakota | 22 | 8353 | 263.4 | 112.8% |
Meade | South Dakota | 26 | 9735 | 267.1 | 114.4% |
Mellette | South Dakota | 8 | 4107 | 194.8 | 83.4% |
Miner | South Dakota | 11 | 6836 | 160.9 | 68.9% |
Minnehaha | South Dakota | 124 | 57697 | 214.9 | 92.1% |
Moody | South Dakota | 16 | 9341 | 171.3 | 73.4% |
Pennington | South Dakota | 62 | 23799 | 260.5 | 111.6% |
Perkins | South Dakota | 12 | 6585 | 182.2 | 78.1% |
Potter | South Dakota | 14 | 4614 | 303.4 | 130.0% |
Roberts | South Dakota | 25 | 15887 | 157.4 | 67.4% |
Sanborn | South Dakota | 12 | 5754 | 208.6 | 89.3% |
Shannon | South Dakota | 15 | 5366 | 279.5 | 119.7% |
Spink | South Dakota | 20 | 12527 | 159.7 | 68.4% |
St.anley | South Dakota | 3 | 1959 | 153.1 | 65.6% |
Sully | South Dakota | 6 | 2668 | 224.9 | 96.3% |
Todd | South Dakota | 15 | 5714 | 262.5 | 112.4% |
Tripp | South Dakota | 34 | 9937 | 342.2 | 146.6% |
Turner | South Dakota | 27 | 13270 | 203.5 | 87.2% |
Union | South Dakota | 22 | 11675 | 188.4 | 80.7% |
Walworth | South Dakota | 16 | 7274 | 220.0 | 94.2% |
Washabaugh (ext) | South Dakota | 3 | 1980 | 151.5 | 64.9% |
Washington | South Dakota | 5 | 1789 | 279.5 | 119.7% |
Yankton | South Dakota | 27 | 16725 | 161.4 | 69.2% |
Ziebach | South Dakota | 7 | 2875 | 243.5 | 104.3% |
Anderson | Tennessee | 54 | 26504 | 203.7 | 87.3% |
Bedford | Tennessee | 45 | 23151 | 194.4 | 83.3% |
Benton | Tennessee | 32 | 11976 | 267.2 | 114.5% |
Bledsoe | Tennessee | 23 | 8358 | 275.2 | 117.9% |
Blount | Tennessee | 114 | 41116 | 277.3 | 118.8% |
Bradley | Tennessee | 74 | 28498 | 259.7 | 111.2% |
Campbell | Tennessee | 104 | 31131 | 334.1 | 143.1% |
Cannon | Tennessee | 20 | 9880 | 202.4 | 86.7% |
Carroll | Tennessee | 54 | 25978 | 207.9 | 89.0% |
Carter | Tennessee | 89 | 35127 | 253.4 | 108.5% |
Cheatham | Tennessee | 35 | 9928 | 352.5 | 151.0% |
Chester | Tennessee | 22 | 11124 | 197.8 | 84.7% |
Claiborne | Tennessee | 59 | 24657 | 239.3 | 102.5% |
Clay | Tennessee | 21 | 10904 | 192.6 | 82.5% |
Cocke | Tennessee | 46 | 24083 | 191.0 | 81.8% |
Coffee | Tennessee | 48 | 18959 | 253.2 | 108.4% |
Crockett | Tennessee | 33 | 17330 | 190.4 | 81.6% |
Cumberland | Tennessee | 49 | 15592 | 314.3 | 134.6% |
Davidson | Tennessee | 534 | 257267 | 207.6 | 88.9% |
Decatur | Tennessee | 23 | 10261 | 224.1 | 96.0% |
Dekalb | Tennessee | 22 | 14588 | 150.8 | 64.6% |
Dickson | Tennessee | 44 | 19718 | 223.1 | 95.6% |
Dyer | Tennessee | 74 | 34920 | 211.9 | 90.8% |
Fayette | Tennessee | 27 | 30322 | 89.0 | 38.1% |
Fentress | Tennessee | 55 | 14262 | 385.6 | 165.2% |
Franklin | Tennessee | 58 | 23892 | 242.8 | 104.0% |
Gibson | Tennessee | 94 | 44835 | 209.7 | 89.8% |
Giles | Tennessee | 62 | 29240 | 212.0 | 90.8% |
Grainger | Tennessee | 29 | 14356 | 202.0 | 86.5% |
Greene | Tennessee | 98 | 39405 | 248.7 | 106.5% |
Grundy | Tennessee | 25 | 11552 | 216.4 | 92.7% |
Hamblen | Tennessee | 53 | 18611 | 284.8 | 122.0% |
Hamilton | Tennessee | 410 | 180478 | 227.2 | 97.3% |
Hancock | Tennessee | 14 | 11231 | 124.7 | 53.4% |
Hardeman | Tennessee | 36 | 23590 | 152.6 | 65.4% |
Hardin | Tennessee | 55 | 17806 | 308.9 | 132.3% |
Hawkins | Tennessee | 80 | 28523 | 280.5 | 120.1% |
Haywood | Tennessee | 25 | 27699 | 90.3 | 38.7% |
Henderson | Tennessee | 41 | 19220 | 213.3 | 91.4% |
Henry | Tennessee | 66 | 25877 | 255.1 | 109.3% |
Hickman | Tennessee | 41 | 14873 | 275.7 | 118.1% |
Houston | Tennessee | 11 | 6432 | 171.0 | 73.3% |
Humphreys | Tennessee | 32 | 12421 | 257.6 | 110.4% |
Jackson | Tennessee | 36 | 15082 | 238.7 | 102.2% |
Jefferson | Tennessee | 79 | 18621 | 424.3 | 181.7% |
Johnson | Tennessee | 34 | 12998 | 261.6 | 112.0% |
Knox | Tennessee | 454 | 178468 | 254.4 | 109.0% |
Lake | Tennessee | 26 | 11235 | 231.4 | 99.1% |
Lauderdale | Tennessee | 43 | 24461 | 175.8 | 75.3% |
Lawrence | Tennessee | 64 | 28726 | 222.8 | 95.4% |
Lewis | Tennessee | 18 | 5849 | 307.7 | 131.8% |
Lincoln | Tennessee | 52 | 27214 | 191.1 | 81.8% |
Loudon | Tennessee | 63 | 19838 | 317.6 | 136.0% |
Macon | Tennessee | 36 | 14904 | 241.5 | 103.5% |
Madison | Tennessee | 91 | 54115 | 168.2 | 72.0% |
Marion | Tennessee | 43 | 19140 | 224.7 | 96.2% |
Marshall | Tennessee | 26 | 16030 | 162.2 | 69.5% |
Maury | Tennessee | 82 | 40357 | 203.2 | 87.0% |
Mcminn | Tennessee | 79 | 30781 | 256.7 | 109.9% |
Mcnairy | Tennessee | 46 | 20424 | 225.2 | 96.5% |
Meigs | Tennessee | 13 | 6393 | 203.3 | 87.1% |
Monroe | Tennessee | 67 | 24275 | 276.0 | 118.2% |
Montgomery | Tennessee | 61 | 33346 | 182.9 | 78.4% |
Moore | Tennessee | 8 | 4093 | 195.5 | 83.7% |
Morgan | Tennessee | 44 | 15242 | 288.7 | 123.7% |
Obion | Tennessee | 54 | 30978 | 174.3 | 74.7% |
Overton | Tennessee | 45 | 18883 | 238.3 | 102.1% |
Perry | Tennessee | 15 | 7535 | 199.1 | 85.3% |
Pickett | Tennessee | 16 | 6213 | 257.5 | 110.3% |
Polk | Tennessee | 38 | 15473 | 245.6 | 105.2% |
Putnam | Tennessee | 68 | 26250 | 259.0 | 111.0% |
Rhea | Tennessee | 49 | 16353 | 299.6 | 128.3% |
Roane | Tennessee | 92 | 27795 | 331.0 | 141.8% |
Robertson | Tennessee | 37 | 29046 | 127.4 | 54.6% |
Rutherford | Tennessee | 53 | 33604 | 157.7 | 67.6% |
Scott | Tennessee | 60 | 15966 | 375.8 | 161.0% |
Sequatchie | Tennessee | 14 | 5038 | 277.9 | 119.0% |
Sevier | Tennessee | 69 | 23291 | 296.3 | 126.9% |
Shelby | Tennessee | 662 | 358250 | 184.8 | 79.2% |
Smith | Tennessee | 40 | 16148 | 247.7 | 106.1% |
St.ewart | Tennessee | 25 | 13549 | 184.5 | 79.0% |
Sullivan | Tennessee | 168 | 69085 | 243.2 | 104.2% |
Sumner | Tennessee | 65 | 32719 | 198.7 | 85.1% |
Tipton | Tennessee | 49 | 28036 | 174.8 | 74.9% |
Trousdale | Tennessee | 12 | 6113 | 196.3 | 84.1% |
Unicoi | Tennessee | 54 | 14128 | 382.2 | 163.7% |
Union | Tennessee | 13 | 9030 | 144.0 | 61.7% |
Van Buren | Tennessee | 9 | 4090 | 220.0 | 94.3% |
Warren | Tennessee | 44 | 19764 | 222.6 | 95.4% |
Washington | Tennessee | 137 | 51631 | 265.3 | 113.7% |
Wayne | Tennessee | 32 | 13638 | 234.6 | 100.5% |
Weakley | Tennessee | 51 | 29498 | 172.9 | 74.1% |
White | Tennessee | 47 | 15983 | 294.1 | 126.0% |
Williamson | Tennessee | 56 | 25220 | 222.0 | 95.1% |
Wilson | Tennessee | 52 | 25267 | 205.8 | 88.2% |
Anderson | Texas | 70 | 37092 | 188.7 | 80.8% |
Andrews | Texas | 8 | 1277 | 626.5 | 268.3% |
Angelina | Texas | 77 | 32201 | 239.1 | 102.4% |
Aransas | Texas | 9 | 3469 | 259.4 | 111.1% |
Archer | Texas | 26 | 7599 | 342.2 | 146.6% |
Armstrong | Texas | 8 | 2495 | 320.6 | 137.3% |
Atascosa | Texas | 50 | 19275 | 259.4 | 111.1% |
Austin | Texas | 41 | 17384 | 235.8 | 101.0% |
Bailey | Texas | 12 | 6318 | 189.9 | 81.4% |
Bandera | Texas | 14 | 4234 | 330.7 | 141.6% |
Bastrop | Texas | 59 | 21610 | 273.0 | 116.9% |
Baylor | Texas | 21 | 7755 | 270.8 | 116.0% |
Bee | Texas | 28 | 16481 | 169.9 | 72.8% |
Bell | Texas | 123 | 44863 | 274.2 | 117.4% |
Bexar | Texas | 1075 | 338176 | 317.9 | 136.2% |
Blanco | Texas | 14 | 4264 | 328.3 | 140.6% |
Borden | Texas | 7 | 1396 | 501.4 | 214.8% |
Bosque | Texas | 35 | 15761 | 222.1 | 95.1% |
Bowie | Texas | 131 | 50208 | 260.9 | 111.8% |
Brazoria | Texas | 61 | 27069 | 225.4 | 96.5% |
Brazos | Texas | 62 | 26977 | 229.8 | 98.4% |
Brewster | Texas | 21 | 6478 | 324.2 | 138.9% |
Briscoe | Texas | 14 | 4056 | 345.2 | 147.9% |
Brooks | Texas | 19 | 6362 | 298.6 | 127.9% |
Brown | Texas | 104 | 25924 | 401.2 | 171.8% |
Burleson | Texas | 28 | 18334 | 152.7 | 65.4% |
Burnet | Texas | 32 | 10771 | 297.1 | 127.3% |
Caldwell | Texas | 59 | 24893 | 237.0 | 101.5% |
Calhoun | Texas | 23 | 5911 | 389.1 | 166.7% |
Callahan | Texas | 37 | 11568 | 319.8 | 137.0% |
Cameron | Texas | 182 | 83202 | 218.7 | 93.7% |
Camp | Texas | 23 | 10285 | 223.6 | 95.8% |
Carson | Texas | 24 | 6624 | 362.3 | 155.2% |
Cass | Texas | 71 | 33496 | 212.0 | 90.8% |
Castro | Texas | 5 | 4631 | 108.0 | 46.2% |
Chambers | Texas | 14 | 7511 | 186.4 | 79.8% |
Cherokee | Texas | 72 | 43970 | 163.7 | 70.1% |
Childress | Texas | 34 | 12149 | 279.9 | 119.9% |
Clay | Texas | 29 | 12524 | 231.6 | 99.2% |
Cochran | Texas | 21 | 3735 | 562.2 | 240.8% |
Coke | Texas | 15 | 4590 | 326.8 | 140.0% |
Coleman | Texas | 57 | 20571 | 277.1 | 118.7% |
Collin | Texas | 117 | 47190 | 247.9 | 106.2% |
Collingsworth | Texas | 30 | 10331 | 290.4 | 124.4% |
Colorado | Texas | 33 | 17812 | 185.3 | 79.4% |
Comal | Texas | 28 | 12321 | 227.3 | 97.3% |
Comanche | Texas | 47 | 19245 | 244.2 | 104.6% |
Concho | Texas | 19 | 6192 | 306.8 | 131.4% |
Cooke | Texas | 81 | 24909 | 325.2 | 139.3% |
Coryell | Texas | 62 | 20226 | 306.5 | 131.3% |
Cottle | Texas | 27 | 7079 | 381.4 | 163.4% |
Crane | Texas | 7 | 2841 | 246.4 | 105.5% |
Crockett | Texas | 12 | 2809 | 427.2 | 183.0% |
Crosby | Texas | 21 | 10046 | 209.0 | 89.5% |
Culberson | Texas | 7 | 1653 | 423.5 | 181.4% |
Dallam | Texas | 24 | 6494 | 369.6 | 158.3% |
Dallas | Texas | 1038 | 398564 | 260.4 | 111.6% |
Dawson | Texas | 41 | 15367 | 266.8 | 114.3% |
Deaf Smith | Texas | 22 | 6056 | 363.3 | 155.6% |
Delta | Texas | 32 | 12858 | 248.9 | 106.6% |
Denton | Texas | 83 | 33658 | 246.6 | 105.6% |
De Witt | Texas | 49 | 24935 | 196.5 | 84.2% |
Dickens | Texas | 24 | 7847 | 305.8 | 131.0% |
Dimmit | Texas | 11 | 8542 | 128.8 | 55.2% |
Donley | Texas | 25 | 7487 | 333.9 | 143.0% |
Duval | Texas | 37 | 20565 | 179.9 | 77.1% |
Eastland | Texas | 82 | 30345 | 270.2 | 115.8% |
Ector | Texas | 36 | 15051 | 239.2 | 102.5% |
Edwards | Texas | 9 | 2933 | 306.9 | 131.4% |
Ellis | Texas | 107 | 47733 | 224.2 | 96.0% |
El Paso | Texas | 369 | 131067 | 281.5 | 120.6% |
Erath | Texas | 75 | 20760 | 361.3 | 154.8% |
Falls | Texas | 56 | 35984 | 155.6 | 66.7% |
Fannin | Texas | 97 | 41064 | 236.2 | 101.2% |
Fayette | Texas | 69 | 29246 | 235.9 | 101.1% |
Fisher | Texas | 29 | 12932 | 224.2 | 96.1% |
Floyd | Texas | 23 | 10659 | 215.8 | 92.4% |
Foard | Texas | 16 | 5237 | 305.5 | 130.9% |
Fort Bend | Texas | 54 | 32963 | 163.8 | 70.2% |
Franklin | Texas | 21 | 8378 | 250.7 | 107.4% |
Freestone | Texas | 36 | 21138 | 170.3 | 73.0% |
Frio | Texas | 15 | 9207 | 162.9 | 69.8% |
Gaines | Texas | 17 | 8136 | 208.9 | 89.5% |
Galveston | Texas | 178 | 81173 | 219.3 | 93.9% |
Garza | Texas | 14 | 5678 | 246.6 | 105.6% |
Gillespie | Texas | 22 | 10670 | 206.2 | 88.3% |
Glasscock | Texas | 4 | 1193 | 335.3 | 143.6% |
Goliad | Texas | 21 | 8798 | 238.7 | 102.2% |
Gonzales | Texas | 62 | 26075 | 237.8 | 101.9% |
Gray | Texas | 60 | 23911 | 250.9 | 107.5% |
Grayson | Texas | 179 | 69499 | 257.6 | 110.3% |
Gregg | Texas | 127 | 58027 | 218.9 | 93.7% |
Grimes | Texas | 35 | 21960 | 159.4 | 68.3% |
Guadalupe | Texas | 41 | 25596 | 160.2 | 68.6% |
Hale | Texas | 59 | 18813 | 313.6 | 134.3% |
Hall | Texas | 41 | 12117 | 338.4 | 144.9% |
Hamilton | Texas | 43 | 13303 | 323.2 | 138.5% |
Hansford | Texas | 10 | 2783 | 359.3 | 153.9% |
Hardeman | Texas | 22 | 11073 | 198.7 | 85.1% |
Hardin | Texas | 29 | 15875 | 182.7 | 78.2% |
Harris | Texas | 1059 | 528961 | 200.2 | 85.8% |
Harrison | Texas | 59 | 50900 | 115.9 | 49.7% |
Hartley | Texas | 6 | 1873 | 320.3 | 137.2% |
Haskell | Texas | 47 | 14905 | 315.3 | 135.1% |
Hays | Texas | 36 | 15349 | 234.5 | 100.5% |
Hemphill | Texas | 17 | 4170 | 407.7 | 174.6% |
Henderson | Texas | 75 | 31822 | 235.7 | 101.0% |
Hidalgo | Texas | 208 | 106059 | 196.1 | 84.0% |
Hill | Texas | 95 | 38355 | 247.7 | 106.1% |
Hockley | Texas | 27 | 12693 | 212.7 | 91.1% |
Hood | Texas | 21 | 6674 | 314.7 | 134.8% |
Hopkins | Texas | 67 | 30274 | 221.3 | 94.8% |
Houston | Texas | 91 | 31137 | 292.3 | 125.2% |
Howard | Texas | 66 | 20990 | 314.4 | 134.7% |
Hudspeth | Texas | 7 | 3149 | 222.3 | 95.2% |
Hunt | Texas | 117 | 48793 | 239.8 | 102.7% |
Hutchinson | Texas | 42 | 19069 | 220.3 | 94.3% |
Irion | Texas | 6 | 1963 | 305.7 | 130.9% |
Jack | Texas | 37 | 10206 | 362.5 | 155.3% |
Jackson | Texas | 22 | 11720 | 187.7 | 80.4% |
Jasper | Texas | 36 | 17491 | 205.8 | 88.2% |
Jeff Davis | Texas | 5 | 2375 | 210.5 | 90.2% |
Jefferson | Texas | 326 | 145329 | 224.3 | 96.1% |
Jim Hogg | Texas | 10 | 5449 | 183.5 | 78.6% |
Jim Wells | Texas | 58 | 20239 | 286.6 | 122.8% |
Johnson | Texas | 81 | 30384 | 266.6 | 114.2% |
Jones | Texas | 62 | 23378 | 265.2 | 113.6% |
Karnes | Texas | 35 | 19248 | 181.8 | 77.9% |
Kaufman | Texas | 74 | 38308 | 193.2 | 82.7% |
Kendall | Texas | 10 | 5080 | 196.9 | 84.3% |
Kenedy | Texas | 4 | 700 | 571.4 | 244.8% |
Kent | Texas | 16 | 3413 | 468.8 | 200.8% |
Kerr | Texas | 31 | 11650 | 266.1 | 114.0% |
Kimble | Texas | 14 | 5064 | 276.5 | 118.4% |
King | Texas | 0 | 1066 | 0.0 | 0.0% |
Kinney | Texas | 24 | 4533 | 529.5 | 226.8% |
Kleberg | Texas | 35 | 13344 | 262.3 | 112.4% |
Knox | Texas | 25 | 10090 | 247.8 | 106.1% |
Lamar | Texas | 116 | 50425 | 230.0 | 98.5% |
Lamb | Texas | 42 | 17606 | 238.6 | 102.2% |
Lampasas | Texas | 28 | 9167 | 305.4 | 130.8% |
La Salle | Texas | 30 | 8003 | 374.9 | 160.6% |
Lavaca | Texas | 90 | 25485 | 353.1 | 151.3% |
Lee | Texas | 21 | 12751 | 164.7 | 70.5% |
Leon | Texas | 42 | 17733 | 236.8 | 101.5% |
Liberty | Texas | 52 | 24541 | 211.9 | 90.8% |
Limestone | Texas | 76 | 33781 | 225.0 | 96.4% |
Lipscomb | Texas | 10 | 3764 | 265.7 | 113.8% |
Live Oak | Texas | 14 | 9799 | 142.9 | 61.2% |
Llano | Texas | 13 | 5996 | 216.8 | 92.9% |
Loving | Texas | 4 | 285 | 1403.5 | 601.2% |
Lubbock | Texas | 171 | 51782 | 330.2 | 141.5% |
Lynn | Texas | 35 | 11931 | 293.4 | 125.7% |
Madison | Texas | 13 | 12029 | 108.1 | 46.3% |
Marion | Texas | 10 | 11457 | 87.3 | 37.4% |
Martin | Texas | 15 | 5556 | 270.0 | 115.6% |
Mason | Texas | 14 | 5378 | 260.3 | 111.5% |
Matagorda | Texas | 47 | 20066 | 234.2 | 100.3% |
Maverick | Texas | 30 | 10071 | 297.9 | 127.6% |
Mcculloch | Texas | 42 | 13208 | 318.0 | 136.2% |
Mclennan | Texas | 291 | 101898 | 285.6 | 122.3% |
Mcmullen | Texas | 8 | 1374 | 582.2 | 249.4% |
Medina | Texas | 23 | 16106 | 142.8 | 61.2% |
Menard | Texas | 21 | 4521 | 464.5 | 199.0% |
Midland | Texas | 41 | 11721 | 349.8 | 149.8% |
Milam | Texas | 73 | 33120 | 220.4 | 94.4% |
Mills | Texas | 19 | 7951 | 239.0 | 102.4% |
Mitchell | Texas | 35 | 12477 | 280.5 | 120.2% |
Montague | Texas | 56 | 20442 | 273.9 | 117.3% |
Montgomery | Texas | 44 | 23055 | 190.8 | 81.7% |
Moore | Texas | 13 | 4461 | 291.4 | 124.8% |
Morris | Texas | 22 | 9810 | 224.3 | 96.1% |
Motley | Texas | 10 | 4994 | 200.2 | 85.8% |
Nacogdoches | Texas | 81 | 35392 | 228.9 | 98.0% |
Navarro | Texas | 116 | 51308 | 226.1 | 96.8% |
Newton | Texas | 13 | 13700 | 94.9 | 40.6% |
Nolan | Texas | 64 | 17309 | 369.7 | 158.4% |
Nueces | Texas | 244 | 92661 | 263.3 | 112.8% |
Ochiltree | Texas | 12 | 4213 | 284.8 | 122.0% |
Oldham | Texas | 8 | 1385 | 577.6 | 247.4% |
Orange | Texas | 52 | 17382 | 299.2 | 128.1% |
Palo Pinto | Texas | 48 | 18456 | 260.1 | 111.4% |
Panola | Texas | 34 | 22513 | 151.0 | 64.7% |
Parker | Texas | 62 | 20482 | 302.7 | 129.7% |
Parmer | Texas | 15 | 5890 | 254.7 | 109.1% |
Pecos | Texas | 23 | 8185 | 281.0 | 120.4% |
Polk | Texas | 43 | 20635 | 208.4 | 89.3% |
Potter | Texas | 166 | 54265 | 305.9 | 131.0% |
Presidio | Texas | 29 | 10925 | 265.4 | 113.7% |
Rains | Texas | 16 | 7334 | 218.2 | 93.4% |
Randall | Texas | 17 | 7185 | 236.6 | 101.3% |
Reagan | Texas | 8 | 1997 | 400.6 | 171.6% |
Real | Texas | 7 | 2420 | 289.3 | 123.9% |
Red River | Texas | 84 | 29769 | 282.2 | 120.9% |
Reeves | Texas | 23 | 8006 | 287.3 | 123.1% |
Refugio | Texas | 28 | 10383 | 269.7 | 115.5% |
Roberts | Texas | 5 | 1289 | 387.9 | 166.2% |
Robertson | Texas | 47 | 25710 | 182.8 | 78.3% |
Rockwall | Texas | 17 | 7051 | 241.1 | 103.3% |
Runnels | Texas | 46 | 18903 | 243.3 | 104.2% |
Rusk | Texas | 86 | 51023 | 168.6 | 72.2% |
Sabine | Texas | 21 | 10896 | 192.7 | 82.6% |
San Augustine | Texas | 22 | 12471 | 176.4 | 75.6% |
San Jacinto | Texas | 15 | 9056 | 165.6 | 70.9% |
San Patricio | Texas | 49 | 28871 | 169.7 | 72.7% |
San Saba | Texas | 21 | 11012 | 190.7 | 81.7% |
Schleicher | Texas | 3 | 3083 | 97.3 | 41.7% |
Scurry | Texas | 45 | 11545 | 389.8 | 167.0% |
Shackelford | Texas | 15 | 6211 | 241.5 | 103.4% |
Shelby | Texas | 85 | 29235 | 290.7 | 124.5% |
Sherman | Texas | 5 | 2026 | 246.8 | 105.7% |
Smith | Texas | 115 | 69090 | 166.4 | 71.3% |
Somervell | Texas | 5 | 3071 | 162.8 | 69.7% |
St.arr | Texas | 41 | 13312 | 308.0 | 131.9% |
St.ephens | Texas | 33 | 12356 | 267.1 | 114.4% |
St.erling | Texas | 4 | 1404 | 284.9 | 122.0% |
St.onewall | Texas | 14 | 5589 | 250.5 | 107.3% |
Sutton | Texas | 13 | 3977 | 326.9 | 140.0% |
Swisher | Texas | 26 | 6528 | 398.3 | 170.6% |
Tarrant | Texas | 584 | 225521 | 259.0 | 110.9% |
Taylor | Texas | 135 | 44147 | 305.8 | 131.0% |
Terrell | Texas | 16 | 2952 | 542.0 | 232.2% |
Terry | Texas | 36 | 11160 | 322.6 | 138.2% |
Throckmorton | Texas | 18 | 4275 | 421.1 | 180.4% |
Titus | Texas | 46 | 19228 | 239.2 | 102.5% |
Tom Green | Texas | 101 | 39302 | 257.0 | 110.1% |
Travis | Texas | 231 | 111053 | 208.0 | 89.1% |
Trinity | Texas | 31 | 13705 | 226.2 | 96.9% |
Tyler | Texas | 29 | 11948 | 242.7 | 104.0% |
Upshur | Texas | 38 | 26178 | 145.2 | 62.2% |
Upton | Texas | 8 | 4297 | 186.2 | 79.7% |
Uvalde | Texas | 44 | 13246 | 332.2 | 142.3% |
Val Verde | Texas | 43 | 15453 | 278.3 | 119.2% |
Van Zandt | Texas | 70 | 31155 | 224.7 | 96.2% |
Victoria | Texas | 74 | 23741 | 311.7 | 133.5% |
Walker | Texas | 36 | 19868 | 181.2 | 77.6% |
Waller | Texas | 18 | 10280 | 175.1 | 75.0% |
Ward | Texas | 27 | 9575 | 282.0 | 120.8% |
Washington | Texas | 39 | 25387 | 153.6 | 65.8% |
Webb | Texas | 123 | 45916 | 267.9 | 114.7% |
Wharton | Texas | 61 | 36158 | 168.7 | 72.3% |
Wheeler | Texas | 36 | 12411 | 290.1 | 124.2% |
Wichita | Texas | 189 | 73604 | 256.8 | 110.0% |
Wilbarger | Texas | 52 | 20474 | 254.0 | 108.8% |
Willacy | Texas | 25 | 13230 | 189.0 | 80.9% |
Williamson | Texas | 74 | 41698 | 177.5 | 76.0% |
Wilson | Texas | 39 | 17066 | 228.5 | 97.9% |
Winkler | Texas | 17 | 6141 | 276.8 | 118.6% |
Wise | Texas | 54 | 19074 | 283.1 | 121.3% |
Wood | Texas | 76 | 24360 | 312.0 | 133.6% |
Yoakum | Texas | 7 | 5354 | 130.7 | 56.0% |
Young | Texas | 65 | 19004 | 342.0 | 146.5% |
Zapata | Texas | 7 | 3916 | 178.8 | 76.6% |
Zavala | Texas | 28 | 11603 | 241.3 | 103.4% |
Beaver | Utah | 14 | 5014 | 279.2 | 119.6% |
Box Elder | Utah | 59 | 18832 | 313.3 | 134.2% |
Cache | Utah | 82 | 29797 | 275.2 | 117.9% |
Carbon | Utah | 55 | 18459 | 298.0 | 127.6% |
Daggett | Utah | 3 | 564 | 531.9 | 227.8% |
Davis | Utah | 40 | 15784 | 253.4 | 108.6% |
Duchesne | Utah | 27 | 8958 | 301.4 | 129.1% |
Emery | Utah | 16 | 7072 | 226.2 | 96.9% |
Garfield | Utah | 15 | 5253 | 285.6 | 122.3% |
Grand | Utah | 8 | 2070 | 386.5 | 165.5% |
Iron | Utah | 21 | 8331 | 252.1 | 108.0% |
Juab | Utah | 12 | 7392 | 162.3 | 69.5% |
Kane | Utah | 7 | 2561 | 273.3 | 117.1% |
Millard | Utah | 28 | 9613 | 291.3 | 124.8% |
Morgan | Utah | 12 | 2611 | 459.6 | 196.9% |
Piute | Utah | 3 | 2203 | 136.2 | 58.3% |
Rich | Utah | 9 | 2028 | 443.8 | 190.1% |
Salt Lake | Utah | 520 | 211623 | 245.7 | 105.3% |
San Juan | Utah | 10 | 4712 | 212.2 | 90.9% |
Sanpete | Utah | 43 | 16063 | 267.7 | 114.7% |
Sevier | Utah | 17 | 12112 | 140.4 | 60.1% |
Summit | Utah | 10 | 8714 | 114.8 | 49.2% |
Tooele | Utah | 26 | 9133 | 284.7 | 121.9% |
Uintah | Utah | 30 | 9898 | 303.1 | 129.8% |
Utah | Utah | 149 | 57382 | 259.7 | 111.2% |
Wasatch | Utah | 18 | 5754 | 312.8 | 134.0% |
Washington | Utah | 31 | 9269 | 334.4 | 143.3% |
Wayne | Utah | 3 | 2394 | 125.3 | 53.7% |
Weber | Utah | 160 | 56714 | 282.1 | 120.8% |
Accomack | Virginia | 43 | 33030 | 130.2 | 55.8% |
Albemarle | Virginia | 108 | 24652 | 438.1 | 187.7% |
Alleghany | Virginia | 76 | 22688 | 335.0 | 143.5% |
Amelia | Virginia | 16 | 8495 | 188.3 | 80.7% |
Amherst | Virginia | 39 | 20273 | 192.4 | 82.4% |
Appomattox | Virginia | 14 | 9020 | 155.2 | 66.5% |
Arlington | Virginia | 225 | 57040 | 394.5 | 169.0% |
Augusta | Virginia | 125 | 42772 | 292.2 | 125.2% |
Bath | Virginia | 29 | 7191 | 403.3 | 172.7% |
Bedford | Virginia | 80 | 29687 | 269.5 | 115.4% |
Bland | Virginia | 19 | 6731 | 282.3 | 120.9% |
Botetourt | Virginia | 19 | 16447 | 115.5 | 49.5% |
Brunswick | Virginia | 24 | 19575 | 122.6 | 52.5% |
Buchanan | Virginia | 65 | 31477 | 206.5 | 88.5% |
Buckingham | Virginia | 27 | 13398 | 201.5 | 86.3% |
Campbell | Virginia | 170 | 26048 | 652.6 | 279.6% |
Caroline | Virginia | 14 | 13945 | 100.4 | 43.0% |
Carroll | Virginia | 57 | 25904 | 220.0 | 94.3% |
Charles City | Virginia | 7 | 4275 | 163.7 | 70.1% |
Charlotte | Virginia | 49 | 15861 | 308.9 | 132.3% |
Chesterfield | Virginia | 52 | 31183 | 166.8 | 71.4% |
Clarke | Virginia | 26 | 7159 | 363.2 | 155.6% |
Craig | Virginia | 8 | 3769 | 212.3 | 90.9% |
Culpeper | Virginia | 26 | 13365 | 194.5 | 83.3% |
Cumberland | Virginia | 10 | 7505 | 133.2 | 57.1% |
Dickenson | Virginia | 64 | 21266 | 300.9 | 128.9% |
Dinwiddie | Virginia | 100 | 18166 | 550.5 | 235.8% |
Elizabeth City | Virginia | 87 | 32283 | 269.5 | 115.4% |
Essex | Virginia | 19 | 7006 | 271.2 | 116.2% |
Fairfax | Virginia | 70 | 40929 | 171.0 | 73.3% |
Fauquier | Virginia | 55 | 21039 | 261.4 | 112.0% |
Floyd | Virginia | 34 | 11967 | 284.1 | 121.7% |
Fluvanna | Virginia | 18 | 7088 | 254.0 | 108.8% |
Franklin | Virginia | 50 | 25864 | 193.3 | 82.8% |
Frederick | Virginia | 57 | 14008 | 406.9 | 174.3% |
Giles | Virginia | 47 | 14635 | 321.1 | 137.6% |
Gloucester | Virginia | 14 | 9548 | 146.6 | 62.8% |
Goochland | Virginia | 15 | 8454 | 177.4 | 76.0% |
Grayson | Virginia | 69 | 21916 | 314.8 | 134.9% |
Greene | Virginia | 16 | 5218 | 306.6 | 131.3% |
Greensville | Virginia | 17 | 14866 | 114.4 | 49.0% |
Halifax | Virginia | 76 | 41271 | 184.1 | 78.9% |
Hanover | Virginia | 33 | 18500 | 178.4 | 76.4% |
Henrico | Virginia | 483 | 41960 | 1151.1 | 493.1% |
Henry | Virginia | 97 | 26481 | 366.3 | 156.9% |
Highland | Virginia | 18 | 4875 | 369.2 | 158.2% |
Isle Of Wight | Virginia | 12 | 13381 | 89.7 | 38.4% |
James City | Virginia | 20 | 4907 | 407.6 | 174.6% |
King And Queen | Virginia | 14 | 6954 | 201.3 | 86.2% |
King George | Virginia | 15 | 5431 | 276.2 | 118.3% |
King William | Virginia | 13 | 7855 | 165.5 | 70.9% |
Lancaster | Virginia | 16 | 8786 | 182.1 | 78.0% |
Lee | Virginia | 101 | 39296 | 257.0 | 110.1% |
Loudoun | Virginia | 36 | 20291 | 177.4 | 76.0% |
Louisa | Virginia | 20 | 13665 | 146.4 | 62.7% |
Lunenburg | Virginia | 39 | 13844 | 281.7 | 120.7% |
Madison | Virginia | 21 | 8465 | 248.1 | 106.3% |
Mathews | Virginia | 10 | 7149 | 139.9 | 59.9% |
Mecklenburg | Virginia | 52 | 31933 | 162.8 | 69.8% |
Middlesex | Virginia | 11 | 6673 | 164.8 | 70.6% |
Montgomery | Virginia | 107 | 21206 | 504.6 | 216.1% |
Nansemond (ext) | Virginia | 43 | 22771 | 188.8 | 80.9% |
Nelson | Virginia | 45 | 16241 | 277.1 | 118.7% |
New Kent | Virginia | 6 | 4092 | 146.6 | 62.8% |
Norfolk (city) | Virginia | 502 | 144332 | 347.8 | 149.0% |
Northampton | Virginia | 46 | 17597 | 261.4 | 112.0% |
Northumberland | Virginia | 12 | 10463 | 114.7 | 49.1% |
Nottoway | Virginia | 35 | 15556 | 225.0 | 96.4% |
Orange | Virginia | 23 | 12649 | 181.8 | 77.9% |
Page | Virginia | 19 | 14863 | 127.8 | 54.8% |
Patrick | Virginia | 35 | 16613 | 210.7 | 90.2% |
Pittsylvania | Virginia | 199 | 61697 | 322.5 | 138.2% |
Powhatan | Virginia | 20 | 5671 | 352.7 | 151.1% |
Prince Edward | Virginia | 40 | 14922 | 268.1 | 114.8% |
Prince George | Virginia | 40 | 12226 | 327.2 | 140.1% |
Prince William | Virginia | 29 | 17738 | 163.5 | 70.0% |
Pulaski | Virginia | 52 | 22767 | 228.4 | 97.8% |
Rappahannock | Virginia | 14 | 7208 | 194.2 | 83.2% |
Richmond | Virginia | 54 | 6634 | 814.0 | 348.7% |
Roanoke | Virginia | 315 | 42897 | 734.3 | 314.5% |
Rockbridge | Virginia | 60 | 22384 | 268.0 | 114.8% |
Rockingham | Virginia | 89 | 31289 | 284.4 | 121.8% |
Russell | Virginia | 67 | 26627 | 251.6 | 107.8% |
Scott | Virginia | 59 | 26989 | 218.6 | 93.6% |
Shenandoah | Virginia | 52 | 20898 | 248.8 | 106.6% |
Smyth | Virginia | 76 | 28861 | 263.3 | 112.8% |
Southampton | Virginia | 28 | 26442 | 105.9 | 45.4% |
Spotsylvania | Virginia | 46 | 9905 | 464.4 | 198.9% |
St.afford | Virginia | 15 | 9548 | 157.1 | 67.3% |
Surry | Virginia | 9 | 6193 | 145.3 | 62.2% |
Sussex | Virginia | 15 | 12485 | 120.1 | 51.5% |
Tazewell | Virginia | 130 | 41607 | 312.4 | 133.8% |
Warren | Virginia | 27 | 11352 | 237.8 | 101.9% |
Warwick | Virginia | 101 | 9248 | 1092.1 | 467.8% |
Washington | Virginia | 120 | 38197 | 314.2 | 134.6% |
Westmoreland | Virginia | 20 | 9512 | 210.3 | 90.1% |
Wise | Virginia | 165 | 52458 | 314.5 | 134.7% |
Wythe | Virginia | 66 | 22721 | 290.5 | 124.4% |
York | Virginia | 7 | 8857 | 79.0 | 33.9% |
Addison | Vermont | 53 | 17944 | 295.4 | 126.5% |
Bennington | Vermont | 52 | 22286 | 233.3 | 99.9% |
Caledonia | Vermont | 50 | 24320 | 205.6 | 88.1% |
Chittenden | Vermont | 140 | 52098 | 268.7 | 115.1% |
Essex | Vermont | 22 | 6490 | 339.0 | 145.2% |
Franklin | Vermont | 63 | 29601 | 212.8 | 91.2% |
Grand Isle | Vermont | 10 | 3802 | 263.0 | 112.7% |
Lamoille | Vermont | 28 | 11028 | 253.9 | 108.8% |
Orange | Vermont | 21 | 17048 | 123.2 | 52.8% |
Orleans | Vermont | 64 | 21718 | 294.7 | 126.2% |
Rutland | Vermont | 110 | 45638 | 241.0 | 103.2% |
Washington | Vermont | 86 | 41546 | 207.0 | 88.7% |
Windham | Vermont | 58 | 27850 | 208.3 | 89.2% |
Windsor | Vermont | 102 | 37862 | 269.4 | 115.4% |
Adams | Washington | 13 | 6209 | 209.4 | 89.7% |
Asotin | Washington | 25 | 8365 | 298.9 | 128.0% |
Benton | Washington | 34 | 12053 | 282.1 | 120.8% |
Chelan | Washington | 61 | 34412 | 177.3 | 75.9% |
Clallam | Washington | 45 | 21848 | 206.0 | 88.2% |
Clark | Washington | 150 | 49852 | 300.9 | 128.9% |
Columbia | Washington | 19 | 5549 | 342.4 | 146.7% |
Cowlitz | Washington | 92 | 40155 | 229.1 | 98.1% |
Douglas | Washington | 17 | 8651 | 196.5 | 84.2% |
Ferry | Washington | 11 | 4701 | 234.0 | 100.2% |
Franklin | Washington | 21 | 6307 | 333.0 | 142.6% |
Garfield | Washington | 7 | 3383 | 206.9 | 88.6% |
Grant | Washington | 25 | 14668 | 170.4 | 73.0% |
Grays Harbor | Washington | 121 | 53166 | 227.6 | 97.5% |
Island | Washington | 10 | 6098 | 164.0 | 70.2% |
Jefferson | Washington | 16 | 8918 | 179.4 | 76.9% |
King | Washington | 1106 | 504980 | 219.0 | 93.8% |
Kitsap | Washington | 142 | 44387 | 319.9 | 137.0% |
Kittitas | Washington | 57 | 20230 | 281.8 | 120.7% |
Klickitat | Washington | 22 | 11357 | 193.7 | 83.0% |
Lewis | Washington | 110 | 41393 | 265.7 | 113.8% |
Lincoln | Washington | 28 | 11361 | 246.5 | 105.6% |
Mason | Washington | 28 | 11603 | 241.3 | 103.4% |
Okanogan | Washington | 45 | 24546 | 183.3 | 78.5% |
Pacific | Washington | 33 | 15928 | 207.2 | 88.7% |
Pend Oreille | Washington | 15 | 7156 | 209.6 | 89.8% |
Pierce | Washington | 417 | 182081 | 229.0 | 98.1% |
San Juan | Washington | 5 | 3157 | 158.4 | 67.8% |
Skagit | Washington | 81 | 37650 | 215.1 | 92.2% |
Skamania | Washington | 4 | 4633 | 86.3 | 37.0% |
Snohomish | Washington | 189 | 88754 | 212.9 | 91.2% |
Spokane | Washington | 396 | 164652 | 240.5 | 103.0% |
St.evens | Washington | 52 | 19275 | 269.8 | 115.6% |
Thurston | Washington | 67 | 37285 | 179.7 | 77.0% |
Wahkiakum | Washington | 3 | 4286 | 70.0 | 30.0% |
Walla Walla | Washington | 70 | 30547 | 229.2 | 98.2% |
Whatcom | Washington | 114 | 60355 | 188.9 | 80.9% |
Whitman | Washington | 45 | 27221 | 165.3 | 70.8% |
Yakima | Washington | 224 | 99019 | 226.2 | 96.9% |
Adams | Wisconsin | 10 | 8449 | 118.4 | 50.7% |
Ashland | Wisconsin | 68 | 21801 | 311.9 | 133.6% |
Barron | Wisconsin | 69 | 34289 | 201.2 | 86.2% |
Bayfield | Wisconsin | 38 | 15827 | 240.1 | 102.8% |
Brown | Wisconsin | 185 | 83109 | 222.6 | 95.3% |
Buffalo | Wisconsin | 24 | 16090 | 149.2 | 63.9% |
Burnett | Wisconsin | 24 | 11382 | 210.9 | 90.3% |
Calumet | Wisconsin | 29 | 17618 | 164.6 | 70.5% |
Chippewa | Wisconsin | 78 | 40703 | 191.6 | 82.1% |
Clark | Wisconsin | 80 | 33972 | 235.5 | 100.9% |
Columbia | Wisconsin | 73 | 32517 | 224.5 | 96.2% |
Crawford | Wisconsin | 38 | 18328 | 207.3 | 88.8% |
Dane | Wisconsin | 330 | 130660 | 252.6 | 108.2% |
Dodge | Wisconsin | 95 | 54280 | 175.0 | 75.0% |
Door | Wisconsin | 40 | 19095 | 209.5 | 89.7% |
Douglas | Wisconsin | 143 | 47119 | 303.5 | 130.0% |
Dunn | Wisconsin | 61 | 27375 | 222.8 | 95.4% |
Eau Claire | Wisconsin | 84 | 46999 | 178.7 | 76.6% |
Florence | Wisconsin | 10 | 4177 | 239.4 | 102.5% |
Fond Du Lac | Wisconsin | 127 | 62353 | 203.7 | 87.2% |
Forest | Wisconsin | 31 | 11805 | 262.6 | 112.5% |
Grant | Wisconsin | 88 | 40639 | 216.5 | 92.8% |
Green | Wisconsin | 23 | 23146 | 99.4 | 42.6% |
Green Lake | Wisconsin | 29 | 14092 | 205.8 | 88.1% |
Iowa | Wisconsin | 34 | 20595 | 165.1 | 70.7% |
Iron | Wisconsin | 34 | 10049 | 338.3 | 144.9% |
Jackson | Wisconsin | 39 | 16599 | 235.0 | 100.6% |
Jefferson | Wisconsin | 76 | 38868 | 195.5 | 83.8% |
Juneau | Wisconsin | 41 | 18708 | 219.2 | 93.9% |
Kenosha | Wisconsin | 151 | 63505 | 237.8 | 101.9% |
Kewaunee | Wisconsin | 32 | 16680 | 191.8 | 82.2% |
La Crosse | Wisconsin | 125 | 59653 | 209.5 | 89.8% |
Lafayette | Wisconsin | 39 | 18695 | 208.6 | 89.4% |
Langlade | Wisconsin | 68 | 23227 | 292.8 | 125.4% |
Lincoln | Wisconsin | 59 | 22536 | 261.8 | 112.1% |
Manitowoc | Wisconsin | 131 | 61617 | 212.6 | 91.1% |
Marathon | Wisconsin | 152 | 75915 | 200.2 | 85.8% |
Marinette | Wisconsin | 78 | 36225 | 215.3 | 92.2% |
Marquette | Wisconsin | 17 | 9097 | 186.9 | 80.0% |
Milwaukee | Wisconsin | 1684 | 766885 | 219.6 | 94.1% |
Monroe | Wisconsin | 73 | 30080 | 242.7 | 104.0% |
Oconto | Wisconsin | 60 | 27075 | 221.6 | 94.9% |
Oneida | Wisconsin | 61 | 18938 | 322.1 | 138.0% |
Outagamie | Wisconsin | 155 | 70032 | 221.3 | 94.8% |
Ozaukee | Wisconsin | 44 | 18985 | 231.8 | 99.3% |
Pepin | Wisconsin | 11 | 7897 | 139.3 | 59.7% |
Pierce | Wisconsin | 56 | 21471 | 260.8 | 111.7% |
Polk | Wisconsin | 54 | 26197 | 206.1 | 88.3% |
Portage | Wisconsin | 85 | 35800 | 237.4 | 101.7% |
Price | Wisconsin | 69 | 18467 | 373.6 | 160.0% |
Racine | Wisconsin | 245 | 94047 | 260.5 | 111.6% |
Richland | Wisconsin | 38 | 20381 | 186.4 | 79.9% |
Rock | Wisconsin | 237 | 80173 | 295.6 | 126.6% |
Rusk | Wisconsin | 34 | 17737 | 191.7 | 82.1% |
Sauk | Wisconsin | 89 | 33700 | 264.1 | 113.1% |
Sawyer | Wisconsin | 39 | 11540 | 338.0 | 144.8% |
Shawano | Wisconsin | 74 | 35378 | 209.2 | 89.6% |
Sheboygan | Wisconsin | 153 | 76221 | 200.7 | 86.0% |
St Croix | Wisconsin | 46 | 24842 | 185.2 | 79.3% |
Taylor | Wisconsin | 39 | 20105 | 194.0 | 83.1% |
Trempealeau | Wisconsin | 48 | 24381 | 196.9 | 84.3% |
Vernon | Wisconsin | 51 | 29940 | 170.3 | 73.0% |
Vilas | Wisconsin | 23 | 8894 | 258.6 | 110.8% |
Walworth | Wisconsin | 64 | 33103 | 193.3 | 82.8% |
Washburn | Wisconsin | 26 | 12496 | 208.1 | 89.1% |
Washington | Wisconsin | 57 | 28430 | 200.5 | 85.9% |
Waukesha | Wisconsin | 121 | 62744 | 192.8 | 82.6% |
Waupaca | Wisconsin | 56 | 34614 | 161.8 | 69.3% |
Waushara | Wisconsin | 21 | 14268 | 147.2 | 63.0% |
Winnebago | Wisconsin | 164 | 80507 | 203.7 | 87.3% |
Wood | Wisconsin | 123 | 44465 | 276.6 | 118.5% |
Barbour | West Virginia | 40 | 19869 | 201.3 | 86.2% |
Berkeley | West Virginia | 77 | 29016 | 265.4 | 113.7% |
Boone | West Virginia | 77 | 28556 | 269.6 | 115.5% |
Braxton | West Virginia | 68 | 21658 | 314.0 | 134.5% |
Brooke | West Virginia | 87 | 25513 | 341.0 | 146.1% |
Cabell | West Virginia | 208 | 97459 | 213.4 | 91.4% |
Calhoun | West Virginia | 24 | 12455 | 192.7 | 82.5% |
Clay | West Virginia | 48 | 15206 | 315.7 | 135.2% |
Doddridge | West Virginia | 29 | 10923 | 265.5 | 113.7% |
Fayette | West Virginia | 223 | 80628 | 276.6 | 118.5% |
Gilmer | West Virginia | 23 | 12046 | 190.9 | 81.8% |
Grant | West Virginia | 18 | 8805 | 204.4 | 87.6% |
Greenbrier | West Virginia | 88 | 38520 | 228.5 | 97.9% |
Hampshire | West Virginia | 36 | 12974 | 277.5 | 118.9% |
Hancock | West Virginia | 111 | 31572 | 351.6 | 150.6% |
Hardy | West Virginia | 25 | 10813 | 231.2 | 99.0% |
Harrison | West Virginia | 201 | 82911 | 242.4 | 103.8% |
Jackson | West Virginia | 38 | 16598 | 228.9 | 98.1% |
Jefferson | West Virginia | 33 | 16762 | 196.9 | 84.3% |
Kanawha | West Virginia | 500 | 195619 | 255.6 | 109.5% |
Lewis | West Virginia | 56 | 22271 | 251.4 | 107.7% |
Lincoln | West Virginia | 69 | 22886 | 301.5 | 129.1% |
Logan | West Virginia | 167 | 67768 | 246.4 | 105.6% |
Marion | West Virginia | 173 | 68683 | 251.9 | 107.9% |
Marshall | West Virginia | 103 | 40189 | 256.3 | 109.8% |
Mason | West Virginia | 58 | 22270 | 260.4 | 111.6% |
Mcdowell | West Virginia | 235 | 94354 | 249.1 | 106.7% |
Mercer | West Virginia | 173 | 68289 | 253.3 | 108.5% |
Mineral | West Virginia | 76 | 22215 | 342.1 | 146.5% |
Mingo | West Virginia | 89 | 40802 | 218.1 | 93.4% |
Monongalia | West Virginia | 156 | 51252 | 304.4 | 130.4% |
Monroe | West Virginia | 44 | 13577 | 324.1 | 138.8% |
Morgan | West Virginia | 25 | 8743 | 285.9 | 122.5% |
Nicholas | West Virginia | 58 | 24070 | 241.0 | 103.2% |
Ohio | West Virginia | 216 | 73115 | 295.4 | 126.5% |
Pendleton | West Virginia | 35 | 10884 | 321.6 | 137.7% |
Pleasants | West Virginia | 15 | 6692 | 224.1 | 96.0% |
Pocahontas | West Virginia | 38 | 13906 | 273.3 | 117.1% |
Preston | West Virginia | 73 | 30416 | 240.0 | 102.8% |
Putnam | West Virginia | 41 | 19511 | 210.1 | 90.0% |
Raleigh | West Virginia | 200 | 86687 | 230.7 | 98.8% |
Randolph | West Virginia | 84 | 30259 | 277.6 | 118.9% |
Ritchie | West Virginia | 48 | 15389 | 311.9 | 133.6% |
Roane | West Virginia | 49 | 20787 | 235.7 | 101.0% |
Summers | West Virginia | 53 | 20409 | 259.7 | 111.2% |
Taylor | West Virginia | 36 | 19919 | 180.7 | 77.4% |
Tucker | West Virginia | 35 | 13173 | 265.7 | 113.8% |
Tyler | West Virginia | 34 | 12559 | 270.7 | 116.0% |
Upshur | West Virginia | 54 | 18360 | 294.1 | 126.0% |
Wayne | West Virginia | 82 | 35566 | 230.6 | 98.8% |
Webster | West Virginia | 48 | 18080 | 265.5 | 113.7% |
Wetzel | West Virginia | 55 | 22342 | 246.2 | 105.4% |
Wirt | West Virginia | 18 | 6475 | 278.0 | 119.1% |
Wood | West Virginia | 107 | 62399 | 171.5 | 73.5% |
Wyoming | West Virginia | 74 | 29774 | 248.5 | 106.5% |
Albany | Wyoming | 39 | 13946 | 279.7 | 119.8% |
Big Horn | Wyoming | 27 | 12911 | 209.1 | 89.6% |
Campbell | Wyoming | 15 | 6048 | 248.0 | 106.2% |
Carbon | Wyoming | 48 | 12644 | 379.6 | 162.6% |
Converse | Wyoming | 18 | 6631 | 271.5 | 116.3% |
Crook | Wyoming | 10 | 5463 | 183.0 | 78.4% |
Fremont | Wyoming | 35 | 16095 | 217.5 | 93.1% |
Goshen | Wyoming | 29 | 12207 | 237.6 | 101.8% |
Hot Springs | Wyoming | 12 | 4607 | 260.5 | 111.6% |
Johnson | Wyoming | 13 | 4980 | 261.0 | 111.8% |
Laramie | Wyoming | 98 | 33651 | 291.2 | 124.7% |
Lincoln | Wyoming | 21 | 10286 | 204.2 | 87.5% |
Natrona | Wyoming | 52 | 23858 | 218.0 | 93.4% |
Niobrara | Wyoming | 8 | 5988 | 133.6 | 57.2% |
Park | Wyoming | 32 | 10976 | 291.5 | 124.9% |
Platte | Wyoming | 25 | 8013 | 312.0 | 133.6% |
Sheridan | Wyoming | 46 | 19255 | 238.9 | 102.3% |
Sublette | Wyoming | 6 | 2778 | 216.0 | 92.5% |
Sweetwater | Wyoming | 50 | 19407 | 257.6 | 110.4% |
Teton | Wyoming | 6 | 2543 | 235.9 | 101.1% |
Uinta | Wyoming | 18 | 7223 | 249.2 | 106.7% |
Washakie | Wyoming | 19 | 5858 | 324.3 | 138.9% |
Weston | Wyoming | 15 | 4958 | 302.5 | 129.6% |
Yellowstone Np (pt) | Wyoming | 0 | 416 | 0.0 | 0.0% |
Graphing the counties according to these statistics reveals something of a “normal” distribution. I should note that sparsely populated counties often exceeded the national norm, although that is often simply a function of math. When Private First Class Keith E. Eberl of Armstrong County, South Dakota, population 42, was killed in action, Armstrong, a county that no longer exists, endured a per capita loss of 2,380 per 100k.
However, as we shall see, what is interesting is that the highly populated counties throughout the country (except for the South, more on that later) fell along the national per capita. Highly populated areas like Chicago, Los Angeles, and New York City sent their boys to fight and die at rates largely in line with the national average (graphic two). The boroughs of New York sent so many of its residents that Army records broke out their losses separately from that of the state of New York. 27,659 New Yorkers were killed in the war.
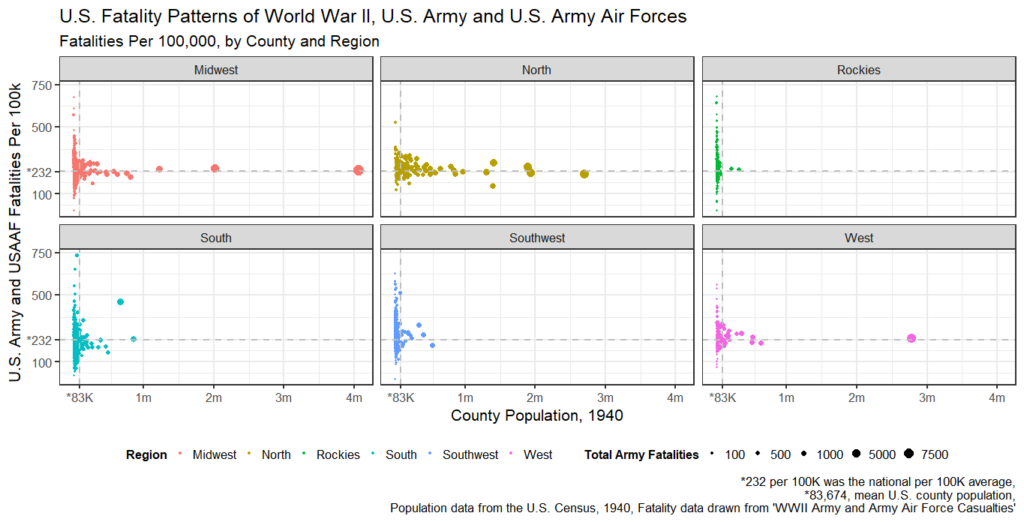
These regional trends began to change first with Korea and then with Vietnam, but not as dramatically as what would come later. During the Big One, of the top 50 most populated counties, 44 were within 90% (or higher) of the national per 100.2 By the Korean War, it fell dramatically to 14 of the top 50 populated counties but rose again in Vietnam to 23. While the geographical spread had indeed changed by Vietnam, a number of America’s most populous counties still pulled their weight. Los Angeles (26.6), Wayne County, MI (which contains Detriot, 30.97), and Kings County, New York (52.6)3, to name a few, all nearly matched or exceeded the national average (graphic 3).
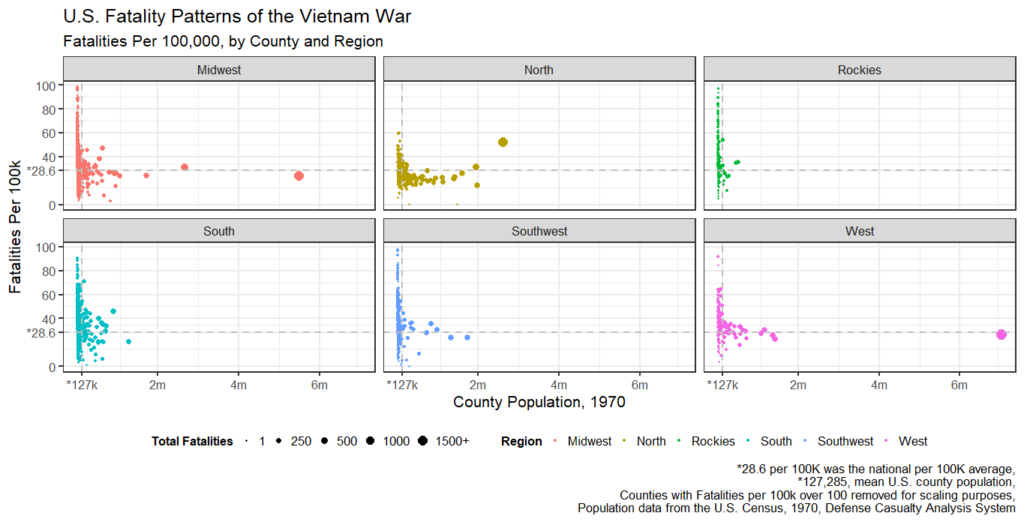
This would change dramatically with the end of conscription in 1973, coupled with socioeconomic and cultural changes associated with military service that arose thereafter. The All-Volunteer Force fundamentally transformed the face of the American military. As the Institute for Defense Analyses has noted, starting in the mid-1980s, recruitment in the Northeast dropped off the table and was largely replaced by Southern and Western states. In the wake of Vietnam, many of the North’s elite schools, including the Ivies, pushed ROTC programs off of their campuses. This slack was taken up by schools in the South and the West and contributed to the shift of identifying military service with their respective regions and political cultures.
The result was the accelerated decline of relative military fatalities from America’s most populated places, especially in the North. Only three of the 25 most populated counties during WWII failed to hit 90% of the national per capita average (Bronx County, St. Louis, and Harris County, Texas, 144, 200, and 200, respectively). If we fast forward to the Global War on Terror, only two of the 25 counties exceeded the national per capita average of 2.10 per 100k (using the 2020 census). See graphic 4.
Those two are Erie County, New York, which lost 27 of its residents (2.27 per 100k), and Baltimore City, which lost 25 (4.25 per 100k). Both are “Rusbelt” cities (the former contains Buffalo) that have bled off residents and their respective industrial bases. The economic landscapes of these counties, like rural areas, incentivized military service.
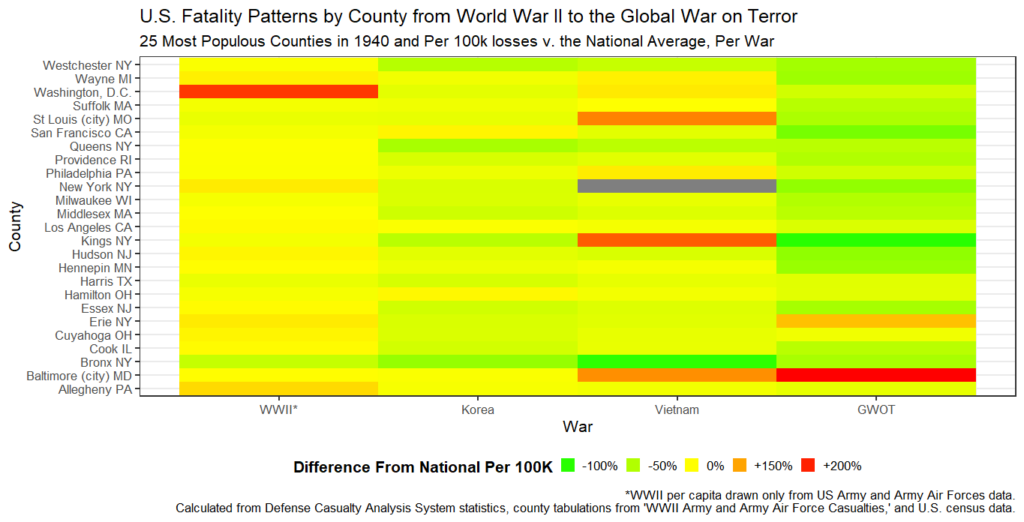
The trend is reinforced by looking at modern population data. Of the 50 most populated counties in the United States (as defined by 2020 data), only seven met or exceeded the national per capita of military fatalities endured in the Global War on Terror. Two of them, San Diego, CA, and Bexar, TX, are military towns, examples of America’s now strained multigenerational military caste.
These transformations have pushed the burden of military fatalities down to less populated counties in the suburbs and exurbs (graphic 5). It should be noted, though, that most large counties still have lost a number of their resident in absolute terms. Los Angeles sacrificed 158, Cook County, 61. Yet these fatalities have been absorbed by massive populations and obscured by the growing socio-economic divide associated with military service. And, in an extreme example, Kings County, New York, population 2.7 million, lost no one in the Global War on Terror.
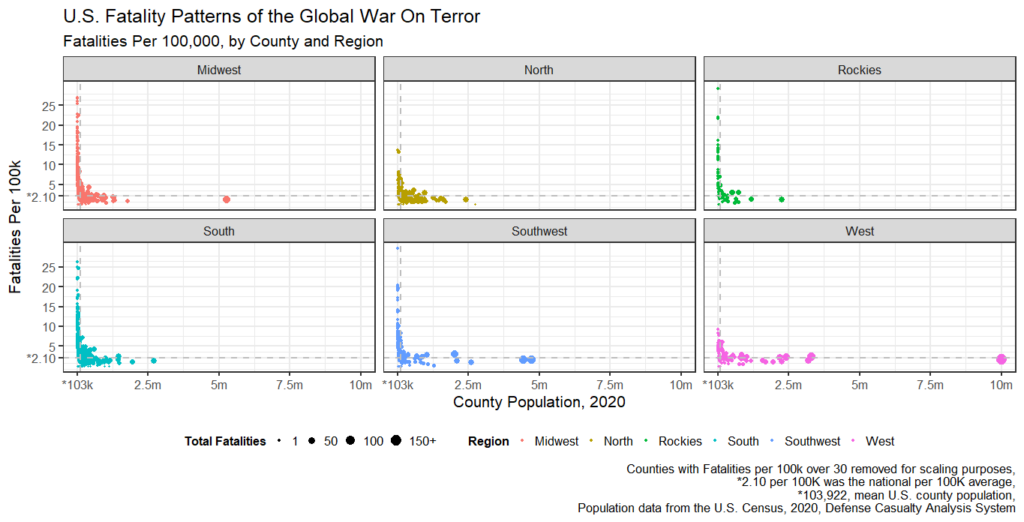
And now for the not-at-all controversial topic of race.
Remember earlier when I noted that the South lost significantly fewer of its residents relative to its population during WWII? Well, there is a reason for that: racism. A combination of exclusionary practices with the draft coupled with a general antipathy towards black combat troops meant a low number of black American wartime fatalities. Despite a number of notable black combat units in armor, infantry, and aviation, a relatively low number of black Americans died in WWII, an estimated 708.
I say estimated because, interestingly enough, the Army fatality records do not record the race (or sex, for that matter) of the deceased. Therefore, tracking racial statistics (an already fraught practice) is especially tough, and it is made tougher still given the black-and-white manner in which racial statistics were gathered in the U.S. in the 1940s (pardon the tasteless pun). Adding to the confusion was the ambiguity of Latinos in the American racial hierarchy of 1940. Latinos were, however, classified as white and served in integrated units.
Yet, if we plot per capita losses against the white population percentage, the South had a lower per capita loss rate as a region because half of its population was (largely) barred from combat (graphic 6). There were outliers, of course, particularly for a few counties in Virginia, but the general pattern holds.
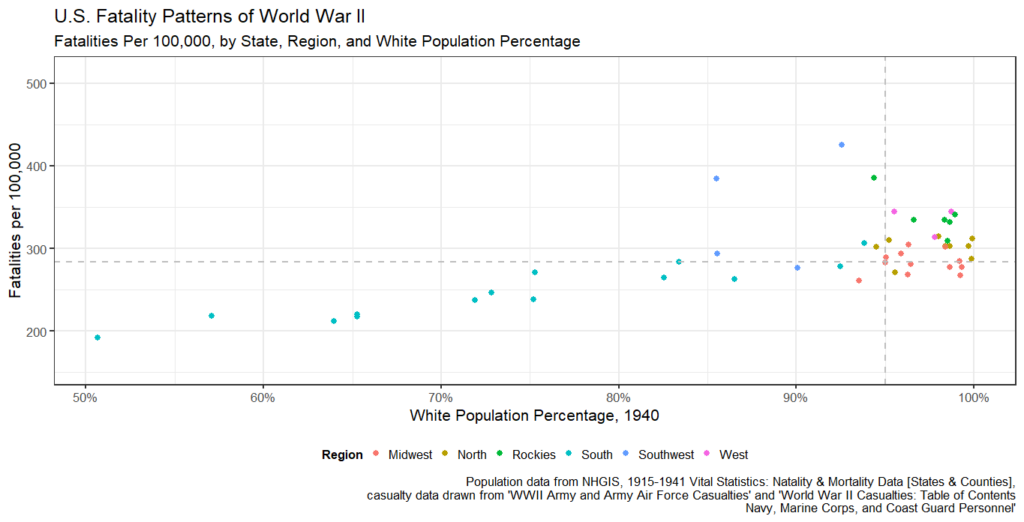
The racial patterns of American combat changed after WWII with the continuation of the draft coupled with the integration of the military. While comparisons across time are difficult due to the changing and often capricious nature of American racial statistics, looking at the DoD’s own fatality statistics, it is clear that by Vietnam, the racial breakdown of American combat largely mirrored that of the county at large (see below).
Yet, since the advent of the All-Volunteer Force, the color of combat has become increasingly white. The elimination of the draft and the adoption of volunteer enlistment coupled with a contract system have created de-facto occupational segregation within the U.S. military, a fact that the Army has itself lamented (if for its own political reasons).
This systematic difference in career paths has led to racial disparities in fatalities endured during the Global War on Terror. As the Department of Defense has acknowledged in its own reporting and its own statistics, around 70% of the country’s GWOT fatalities are white, which is higher than the U.S. military and the nation at large.
There are exceptions, of course. While the DCAS data does not list racial statistics for individual soldiers, the records themselves are enlightening. Looking at the names of the fallen in heavily Latino counties, like Los Angeles, reveals a preponderance of Hispanic surnames, and Baltimore, a predominately black city, lost 32 of its residents, a per capita of 4.2, double the national average.
Yet these are the exceptions that prove the rule: America’s combat fatalities, for the first time since WWII, have become disproportionately white even as the military and, indeed, the country at large are becoming less so. They’ve also become disproportionately rural and underrepresented among America’s upper class. Long gone are the days of UCLA or Harvard sending its students and faculty to fight America’s wars.
The elimination of the draft, moral considerations aside, constructed a military caste within the United States, one served by a volunteer system. After 20 years of lost wars and amplifying domestic issues, that system is heavily under strain. As I argued in my earlier piece for antiwar.com:
These disparities in wartime losses expounded upon another conflict, the culture war. Conservatives, who are overrepresented among veterans, have come to view the foreign policy class and similar coastal elites (to include large swaths of the GOP) with abject disdain. While it is true that the US has been awash in militaristic pageantry since 2001, the right sees the national mood as inherently hostile if not downright contemptuous of them and their values. In their eyes, conservative principles are routinely mocked by the same social class which has sent them or their children to die needlessly abroad. Their home states are referred to derisively as “fly over country.” They have been called “bitter clingers” and “deplorables.” Their religion and patriotism are routinely mocked, as are their cultural values on abortion and guns. Their views on constitutional government are ridiculed as both ahistorical and outdated. They are routinely labeled as racists, sexists, Nazis, homophobes, and so on. Worst of all, they are increasingly compared to those that they fought and are seen as a growing terror threat.
Bear Any Burden: Military Sacrifice and Rise of American Populism – Antiwar.com
It appears that America’s martial caste is now discouraging its sons and daughters from enlisting, draining the U.S. military’s strongest enlistment pool. Now, the smart set, on both the right and left, is floating the idea of bringing back the draft, often invoking World War II nostalgia, framing conscription as a form of “democracy.”
It should go without saying that the United States is not the same place that it was in 1940. Reinstating the draft would create more problems than it would solve. There is, of course, another less draconian solution to the question of who shall bear the burden of America’s future, one rarely floated by the smart set: lighten the load.
Lower the demand by bringing our troops home, make America’s “allies” pay for their own defense, and return to the republic we’re supposed to be. It’s a novel and perhaps impossible idea, but one worth clinging to lest we resign ourselves to future generations buckling under this imperious burden.
- Steven Manson, Jonathan Schroeder, David Van Riper, Katherine Knowles, Tracy Kugler, Finn Roberts, and Steven Ruggles. IPUMS National Historical Geographic Information System: Version 18.0 [1915-1941 Vital Statistics: Natality & Mortality Data [States & Counties]]. Minneapolis, MN: IPUMS. 2023. http://doi.org/10.18128/D050.V18.0 ↩︎
- I used this cut-off for World War II in order to account for the likelihood that naval losses would have put a given country over the threshold of meeting the national average. ↩︎
- That number is likely inflated by an error in the DCAS dataset, one that did not record deaths for New York County. I suspect that residents of New York County were recorded as residing in Kings County, hence the elevated number. ↩︎